Getting realistic about AI’s potential with Nick Frosst from Cohere
08 Aug 2024 (7 months ago)
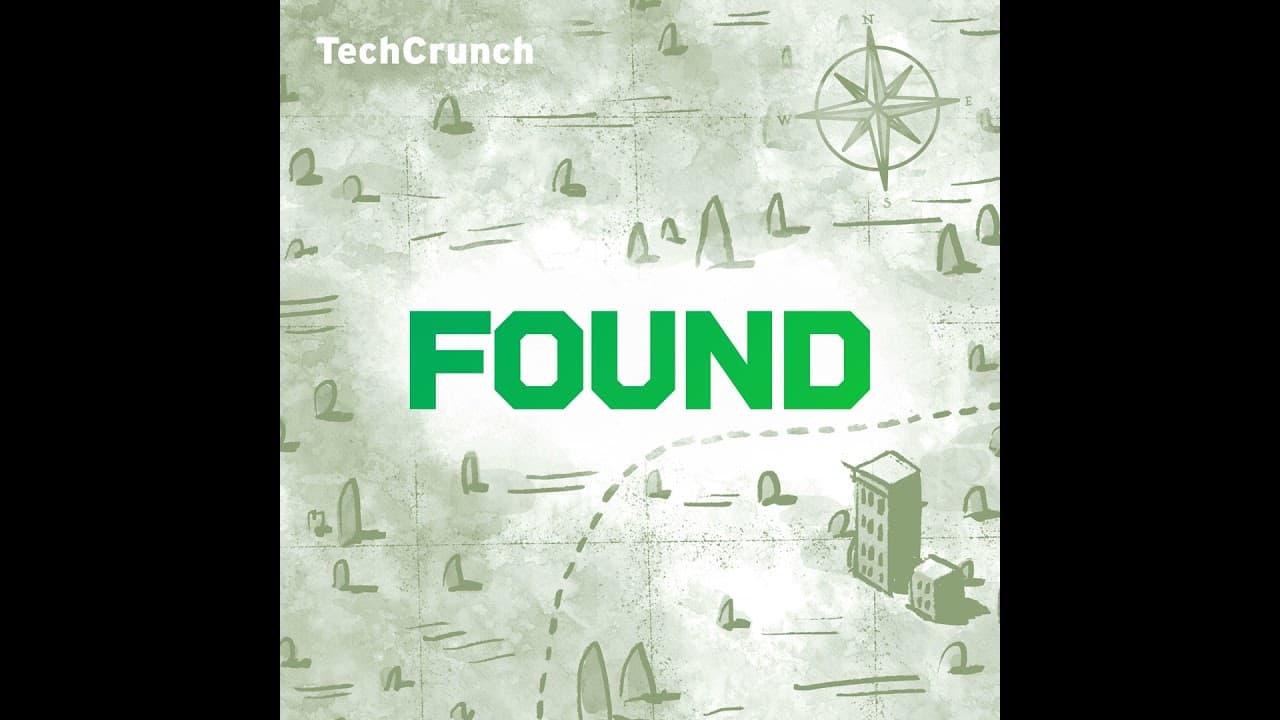
Introduction (0s)
- The text discusses the rise of enterprise AI and the emergence of AI infrastructure companies.
- The text introduces Nick Frosst, co-founder of Cohere, an enterprise-focused large language modeling company.
- Frosst explains that he has been interested in AI since his undergraduate studies at the University of Toronto, where he met Jeff Hinton, a pioneer in the field.
- Frosst describes his early interest in neural networks, particularly in the context of computer vision, and how he was initially surprised by the ability of neural networks to recognize objects like cats and dogs.
- Frosst shares his experience of feeling like he was late to the AI game in 2013, as he was just starting to learn about neural networks while the field was already experiencing significant advancements.
- Frosst discusses the founding of Cohere, which was based on a technical realization by Aiden Gomez, another co-founder, regarding the potential of general-purpose language models.
- Frosst explains that the Transformer, a type of neural network, enabled the creation of general-purpose language models that could perform various tasks, such as extracting numbers from PDFs, summarizing documents, and automating processes.
- Frosst argues that general-purpose language models are more effective than specialized models because language is inherently diverse and complex, requiring a broader understanding of language patterns.
Enterprise applications for AI language models (6m15s)
- Language models require a general understanding of language. Similar to how humans learn language in stages, starting with general concepts and then specializing in specific fields, language models need to be trained on a broad range of text before they can be effectively applied to specific tasks.
- Linguistic knowledge is important but not essential for training language models. While understanding linguistics can be helpful in creating language models, the models themselves are ultimately statistical models that can be trained on any language without requiring specific grammatical knowledge.
- The shift from general-purpose text models to chat models was driven by a change in data, not technology. General-purpose models were trained on text found on the open web, which limited their ability to perform tasks like writing poems. Chat models were fine-tuned on data created by humans that reflected how people wanted to interact with chatbots, making them more versatile and user-friendly.
Building multilingual models (12m12s)
- The conversation has shifted from convincing companies of the usefulness of large language models to focusing on the specific benefits of a particular model.
- The speaker's company prioritizes building models that are useful for enterprises, rather than pursuing general artificial intelligence (AGI).
- The company has invested in multilingual capabilities, particularly in Japanese, due to the large Japanese economy and developer community with limited English proficiency.
- The difficulty in building models for certain languages is primarily due to the lack of available data on the web, as the internet is heavily dominated by English content.
- The speaker explains that if the amount of data available for all languages was equal, there would be no difference in the difficulty of building models for each language.
Fundraising and the AI bubble (14m58s)
- The conversation around AI startups has shifted from focusing on building demos and showcasing technology to emphasizing practical applications and delivering tangible value for enterprises.
- Companies are now prioritizing production-ready solutions, considering factors like total cost of deployment, privacy, and deployment options.
- The speaker believes that the current focus on practical applications and real-world value is a positive development, as it signifies a move away from hype and towards tangible results.
- The speaker's company recently secured significant funding, which will be used to continue building their product and delivering value to enterprises.
- The speaker does not believe that the current AI landscape is a bubble, as they see real value being delivered by their models and believe there is significant potential for further growth and impact.
- The speaker acknowledges that the hype surrounding AI has cooled down, with more people recognizing the technology's limitations and focusing on its practical applications.
- The speaker believes that the shift in focus towards tangible value is a positive development, as it allows for a more realistic and grounded approach to AI development and deployment.
AI and sustainability (19m46s)
- The text discusses the potential negative impacts of AI, including its energy consumption and data privacy implications.
- The speaker emphasizes the importance of addressing these concerns and highlights their company's efforts to mitigate them.
- The speaker acknowledges the potential for overblown rhetoric surrounding AI, both in terms of its capabilities and its risks.
- The speaker advocates for a balanced approach, recognizing the potential benefits of AI while acknowledging its limitations and potential negative consequences.
- The speaker expresses concern about the trend of training increasingly large AI models, arguing that it is unnecessary and potentially harmful due to its energy consumption.
- The speaker believes that AI will become a valuable tool for knowledge workers but will not become a dominant force in energy consumption.
- The speaker emphasizes the importance of considering the source of energy used to power AI models and advocates for the use of sustainable energy sources.
Building the Cohere team (25m14s)
- The speaker discusses the talent pool for AI development, noting that there has always been a strong interest in the field and that the talent pool has grown significantly in recent years.
- The speaker highlights the benefits of building a company in Canada, emphasizing the country's pragmatic and compassionate approach to technology development.
- The speaker emphasizes that Cohere is not limited to building AI in Canada, as they also have offices and operations in San Francisco, New York, London, and Paris.
- The speaker attributes the strong AI talent pool in Canada to the presence of prominent figures like Geoffrey Hinton and the government's investment in education.
- The speaker outlines Cohere's future plans, which include focusing on making large language models (LLMs) more useful for businesses by improving their multilingual capabilities, data security, and ability to reduce hallucinations.
Host discussion (29m44s)
- The discussion centers around the potential of AI and whether it is overhyped. The participants agree that there is a lot of fear-mongering surrounding AI, with people predicting apocalyptic scenarios.
- The participants discuss the sustainability concerns surrounding AI, particularly the energy consumption of AI models. They acknowledge that AI development is still in its early stages and that energy consumption will likely decrease as the technology matures.
- The discussion touches on the rapid growth of the AI industry and the fact that many AI companies are now valued at billions of dollars. The participants discuss the fundraising landscape for AI companies and the role of Canadian investors in the space.
- The participants also discuss AI regulation and how it might differ in Canada compared to the EU and the US. They acknowledge that AI companies with global customers will need to comply with regulations in multiple jurisdictions.
- The discussion concludes with a reflection on whether the AI industry is in a bubble. The participants agree that the industry is not in a bubble because there are real companies building real value. They also discuss the characteristics of a bubble and how the AI industry might not fit the definition.