Stanford Webinar - Identifying AI Opportunities: Strategies for Market Success
28 Aug 2024 (21 days ago)
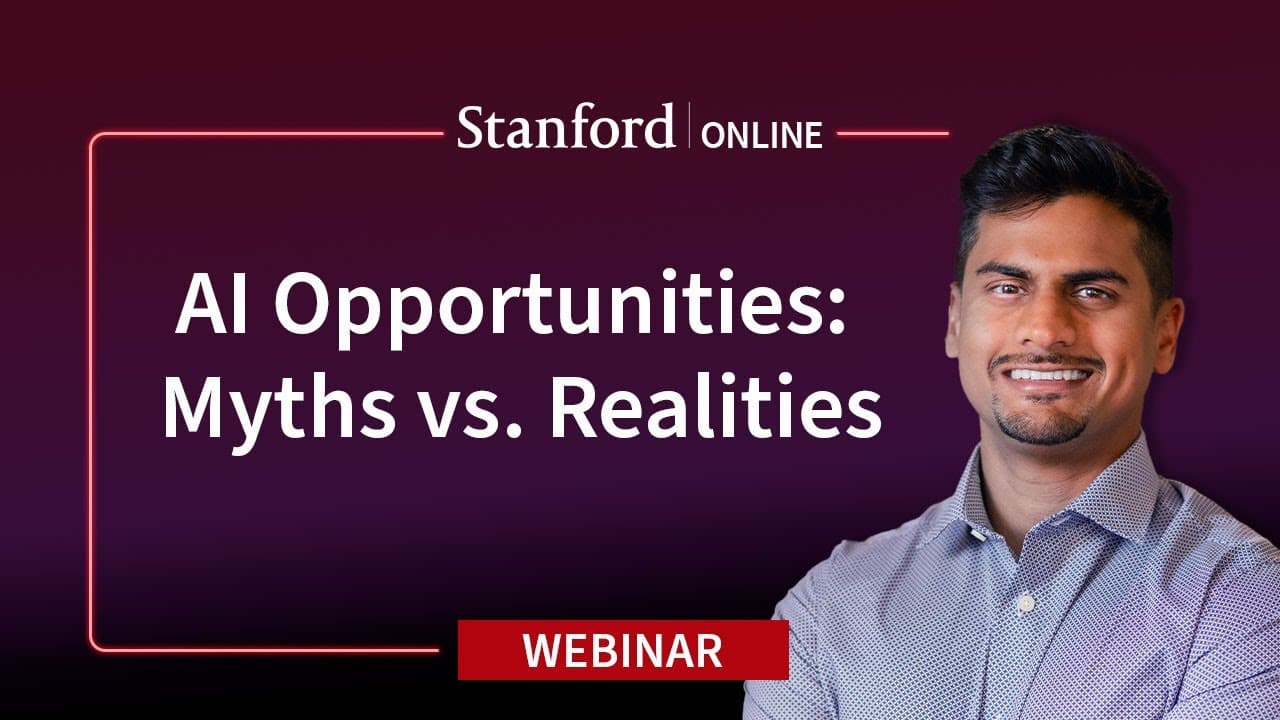
Generative AI Landscape and Opportunities
- Aditya Chapali is a machine learning engineer and product lead at Microsoft, where he helps build products used globally.
- Chapali is also an advisor to several Silicon Valley startups and Fortune 500 companies.
- The information presented in the webinar is derived from a course taught by Chapali and a Stanford professional, based on research involving interviews with over 50 executives and a user research survey of over 300 participants.
- There are three types of generative AI applications: collaborative, personalized, and proactive.
- There are three ways to access generative AI models: calling a model creator through an API, hosting an open-source model, or creating a model.
- There are three types of companies that utilize generative AI: takers who put a user interface on an existing model, model customizers who add their own data to a model, and model creators who build models.
- Mistral AI and Thomson Reuters Westlaw are two companies involved in the AI industry. Mistral AI creates AI models, while Westlaw is a legal database containing legal cases and their outcomes.
- There is a common misconception that model creators like Mistral AI will generate more revenue than companies like Westlaw. However, the reality is that models are becoming increasingly customized, leading to competition among model creators and potentially limiting their profitability.
- Contrary to the belief that data is the most crucial element in AI, large companies possess another significant competitive advantage, which remains undisclosed.
- Distribution is the most important factor for success in the current AI landscape, surpassing the need for superior data or user experience.
- While IBM may appear better positioned for success in AI due to its resources, JP Morgan's wider distribution network and potential for data and user experience flywheel make it the stronger contender.
- Non-tech companies with existing distribution channels and user bases have an advantage in the generative AI market, as they can integrate AI into their systems and leverage their reach.
- Non-technology companies are positioned to succeed with AI like never before, potentially even surpassing tech companies in the future.
- Startups have a significant opportunity in the AI space because many large companies, especially non-tech companies, are slow to adopt AI technology.
Building with Generative AI
- Non-technical professionals can now build with AI without needing to code, enabling them to act as data scientists by providing LLMs with data and tuning prompts.
- There are three stages to becoming technically proficient in generative AI: beginner, intermediate, and advanced. The advanced stage, which involves techniques like multi-channel prompting, JSON formatting, and checker LLMs, offers the highest earning potential.
- Understanding data boundaries and associated systems architecture is crucial. Many companies are unaware that they can use generative AI within their data boundaries without violating privacy regulations. Professionals with this knowledge are highly valuable.
- At an advanced level, there are two paths: diving deep into systems architecture and communicating technical concepts effectively, or utilizing low-code/no-code tools with generative AI capabilities to automate tasks or build applications. Both paths offer significant earning potential.
- Many individuals are generating income by utilizing large language models (LLMs) like ChatGPT to create applications through prompts, even without coding knowledge. For instance, a nurse leveraged ChatGPT to develop a patient intake tool that proved to be profitable.
Effective Implementation of Generative AI
- Building internal-facing tools is a common mistake when implementing generative AI. Research indicates that a significant majority of individuals who have implemented generative AI products (primarily chatbots or internal tools) find them ineffective.
- Instead of internal tools or chatbots, prioritize user-facing features. Begin with a private preview, avoid open-ended interactions, and concentrate on in-product features. This approach facilitates the development of essential skills for creating successful user-facing features.
- Users prefer AI tools that prioritize content consumption (e.g., summarizing key insights from large amounts of information) over content creation.
- Instead of focusing on generating content like legal documents or sales proposals, AI tools should be used to analyze information and provide insights, such as advising on legal strategy or refining sales pitches.
- Building AI features directly into existing products is significantly more effective than creating standalone chatbots.
Generative AI Companies: Success and Failure
- Chatbase takes text and makes it searchable on a website.
- Cohere makes AI models, but has had to lay off employees and cut back deals.
- Riz GPT was sold for millions of dollars.
Business Professionals in the Age of AI
- Business professionals are in high demand to understand and lead in the application of AI, particularly generative AI, within their organizations.
- A survey of business leaders indicated that understanding the technical aspects of AI is now the most important skill for business professionals, even more critical than deep domain expertise.
- While many business professionals lean towards gaining deeper domain expertise, becoming more technical is recommended as it is less competitive and creates valuable "unicorns" who can bridge the gap between technical and business aspects.