The Truth About Building AI Startups Today
09 Feb 2024 (1 year ago)
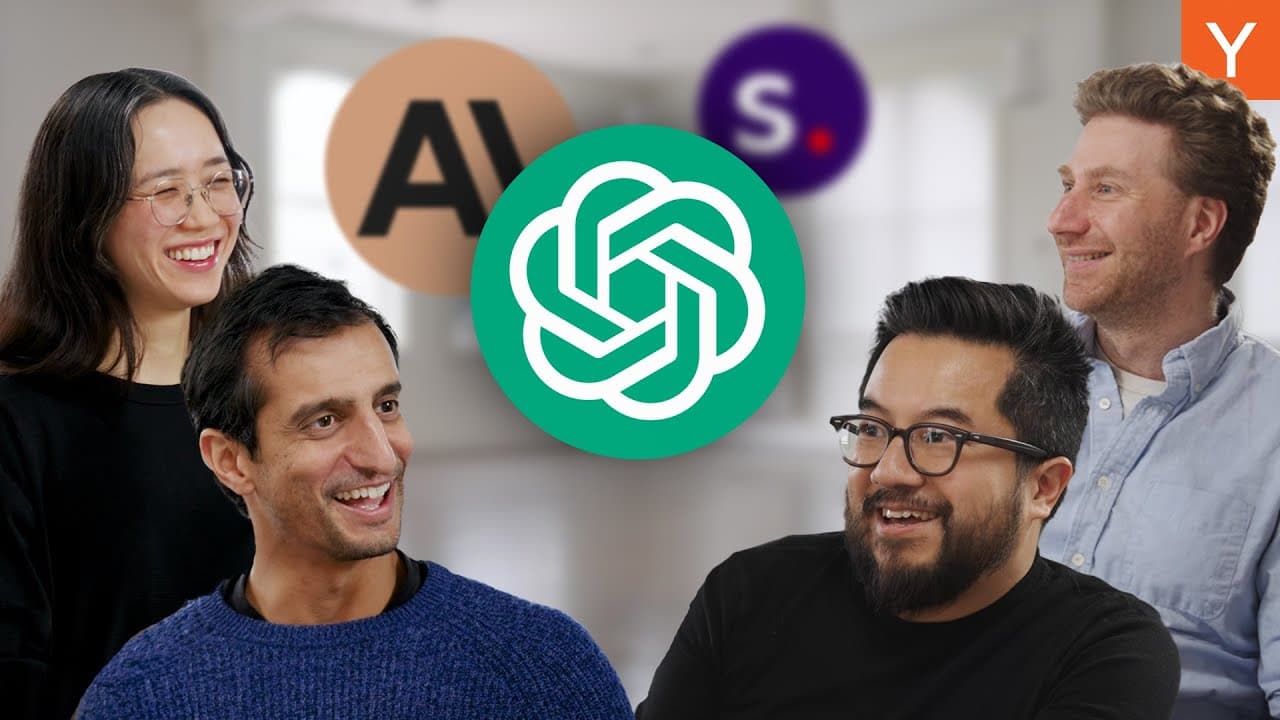
Intro (0s)
- Discusses the potential of AI and how it could lead to the creation of billion-dollar companies.
- Questions the common belief that only exciting or groundbreaking ideas can be successful, suggesting that even seemingly boring ideas might have incredible business potential.
- Raises concerns about the impact of AI agents like GPT-5 and their ability to pass the Turing test, challenging the traditional chat interface.
- Highlights the current excitement surrounding AI and the opportunities it presents for building generational companies.
YC Recent Batch (1m53s)
- Reveals that nearly 50% of the startups in Y Combinator's summer 2023 batch were working on large language models.
- Clarifies that YC does not specifically favor AI companies and that the high percentage of AI startups in the batch reflects the interests and ambitions of the founders themselves.
- Suggests that the current wave of AI advancements, particularly the capabilities of GPT-3.5 and GPT-4, has captured the attention and interest of many smart founders and investors.
- Emphasizes the belief that generational companies are being built in the field of AI and that this is an exciting time for innovation and growth.
College Students and AI Startups (3m34s)
- College students are dropping out to start AI startups because they see it as a once-in-a-lifetime opportunity.
- College students are well-positioned to work in AI because everyone is starting from the same level of experience.
- Developer tools for prompt engineering are becoming popular among college students.
AI Startup Success Factors (4m44s)
- Successful AI startups are often focused on workflow automation, replacing repetitive tasks done by humans with LLMs.
- There are many job opportunities in the world that involve mundane information processing, which is a perfect fit for LLMs.
- Despite the potential, there aren't many applications from founders working on mundane AI tasks.
Opportunities in Mundane AI Tasks (5m53s)
- An example of a successful mundane AI startup is Sweetspot, which automates the process of searching for and submitting proposals for government contracts.
- Boring tasks can actually be incredible business opportunities, as highlighted in an essay by Paul Graham.
Beware of "Tarpit Ideas" (7m30s)
- Tarpit ideas are initially attractive but turn out to be poor startup ideas.
- Founders get stuck in tarpit ideas and continue working on them despite their lack of viability.
- AI co-pilot is a common tarpit idea in AI.
- Potential customers are interested in AI co-pilots but struggle to find practical use cases.
- Chat interfaces require users to adapt their communication style to suit the computer, which can be limiting.
AI Integration into UIs (9m36s)
- In the near future, we will become more accustomed to using AI in user interfaces.
- The most promising approach currently is to use large language models to perform knowledge work and integrate it into familiar user interfaces.
- This approach leverages the power of AI without requiring users to significantly change their usage patterns.
- It is similar to how past technological advancements have been integrated into software without drastically altering user behavior.
Avoiding the "Checkbox Mentality" (10m30s)
- Many companies adopt AI strategies simply to check a box and satisfy a trend, without a clear plan for success.
- The AI co-pilot concept may not have found product-market fit yet and could potentially fail.
- Founders should consider building a competitor with a co-pilot as the main experience to validate the demand and potential for success.
Focus on Genuine Use Cases (11m54s)
- The AI industry is still in its early stages, and many end applications lack product-market fit.
- Startups may gain initial traction but lack actual usage and value for customers.
- Even companies selling tools to AI companies can be affected when their customers lose contracts due to the rapid evolution of AI technology.
- Offering fine-tuning of open-source models as a service is an idea worth exploring in the current AI landscape.
Fine-Tuning Open-Source Models (13m45s)
- Initially, the demand for fine-tuned open-source models was driven by cost, as they were cheaper alternatives to expensive models like OpenAI's chat GPT.
- However, cost alone is no longer sufficient to retain customers, as the cost of all models continues to decrease.
- To stay competitive, fine-tuning companies need to offer more than just lower cost, such as customizing models to private datasets that organizations cannot share or modify themselves.
Data Privacy Concerns (15m20s)
- The emergence of new technologies like LLMs resets the competitive landscape, leading to the creation of new industries.
- Just as cloud computing gave rise to cloud cybersecurity companies, LLMs are now creating a demand for cybersecurity solutions specific to these models.
- Companies like Prompt Armor are developing solutions to prevent LLMs from revealing private data that has been used for fine-tuning or training.
- Controlling access to LLMs and the data they can access is a promising area for building new software.
- Beyond fine-tuning, there is growing interest in building purpose-trained models that are smaller and can run locally on machines for inference.
- Customizing and training models on specific domains and target data can lead to better performance compared to general models trained on a wide range of tasks.
Purpose-Trained AI Models (17m9s)
- Startups are building AI models specifically trained for tasks like parsing SQL queries, coding assistance, and hardware design.
- Customizing large language models for specific domains can yield better results than using general-purpose models.
- The vocabulary for a specific domain is smaller than human language, making it easier to train a custom model.
AI Models for Prototyping (18m36s)
- Large language models like GPT-4 can be used as prototyping tools for AI applications.
- Customizing a large language model for a specific task can result in a more efficient and accurate model.
- This approach allows startups to quickly iterate on their ideas and build tailored AI solutions.
Surge in Startup Ideas (19m45s)
- The recent advancements in AI have led to a surge in potential startup ideas.
- Many companies that join YC pivot to new ideas within a month of receiving funding.
- The ease of finding great startup ideas was unprecedented in the last batch, with founders coming up with good ideas faster than ever before.
- The popularity of ChatGPT has inspired a wave of generative AI startups focused on automating tasks like marketing copy and creative content.
The "GPT Wrapper" Term (20m54s)
- People are using the term "GPT wrapper" to describe AI startups that are simply building a user interface on top of large language models like ChatGPT.
- This is similar to how SAS software was just a database wrapper in the early days.
- The chat interface is not the only way to build AI-powered software. Good UX, copy, interaction design, and information hierarchy are all important factors in building successful software.
Importance of UX (22m2s)
- Building software that is timeless and transcends the use of large language models requires craft and attention to user experience.
- Founders should focus on solving specific problems and needs for users rather than building generic solutions that can be easily replicated by large language models.
Focus on Specific Problems (23m39s)
- Successful AI startups will focus on solving specific problems and providing value through custom business logic.
- Examples of potential AI-powered software include sales rep voice AI apps, CRM systems that go beyond traditional CRM functionality, and software that automates government form processing.
- The scope of software that can be built with AI today is vast, and founders should look at existing software and reimagine how it could be improved with AI.
AI-Powered Voice Agents (25m3s)
- AI voice agents for small businesses are being developed to automate tasks such as scheduling and customer service.
- Concerns exist about the potential for malicious AI agents and the need for defensive AI to protect against scams.
- Open-source AI is advocated as a means to ensure equity and prevent a single dominant AGI controlled by a single entity.
Advocacy for Open-Source AI (26m38s)
- Open-source AI is seen as a way to democratize access to AI technology and prevent monopolies.
- The importance of open-source AI is emphasized by the growth and energy seen at recent AI conferences.
Resurgence of AI Researcher-Founders (27m29s)
- AI researcher-founders are on the rise, with many researchers from the influential 2017 paper "Attention Is All You Need" starting their own companies.
- This trend is reminiscent of the early days of YC when the internet was new and the people building on it were mostly technologists.
- The current wave of AI startups is driven by real new technology being invented, rather than just innovating on business models with commoditized technology.
Periodic Dismissal of Emerging Tech (30m15s)
- Emerging technologies are often dismissed as toys or fads, but this has been the case with many great technologies in the past.
- The current dismissal of AI is similar to the dismissal of personal computers, the internet, and other major technological advancements.
- Despite the skepticism, AI is one of the most interesting things happening right now and should not be written off.
- Instead of being attracted to the new shiny thing, entrepreneurs should look for the "muck" where there's brass, as there are potential opportunities in areas that are currently overlooked or undervalued.