A conversation with Kevin Weil (OpenAI CPO), Mike Krieger (Anthropic CPO), Sarah Guo (Conviction)
06 Nov 2024 (5 months ago)
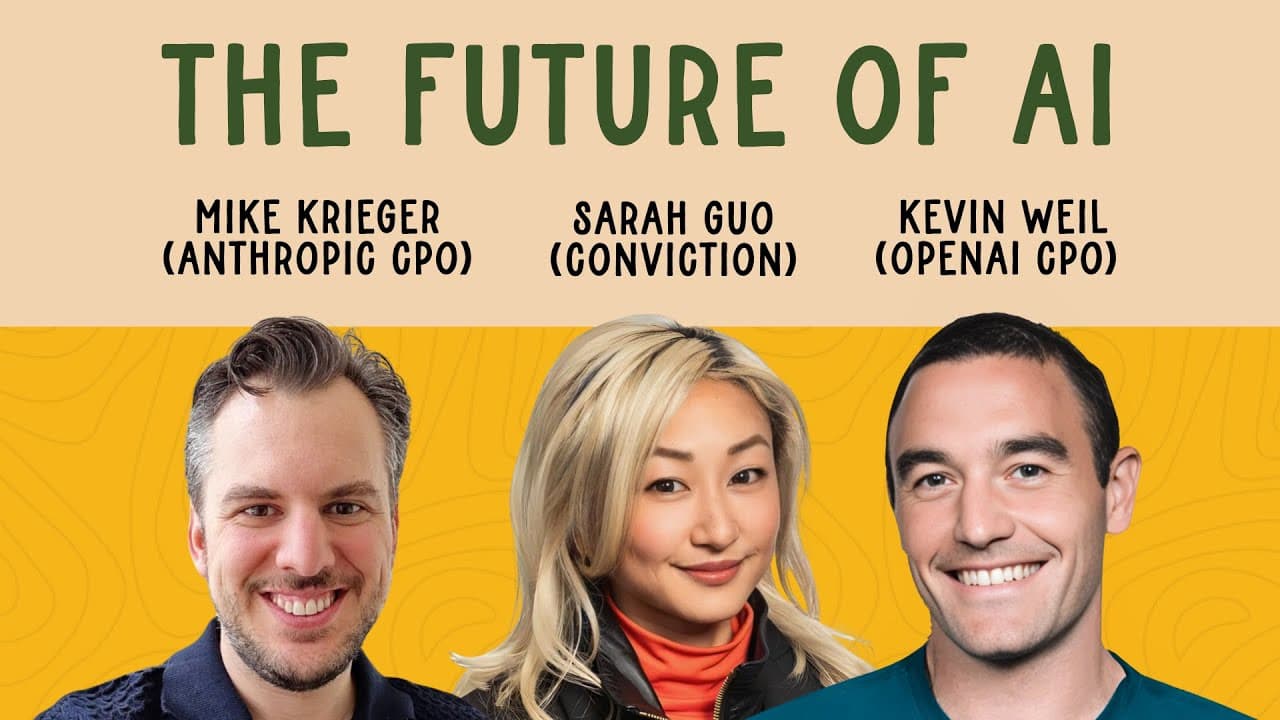
Kevin Weil's Role at OpenAI and Initial Reactions
- Kevin Weil took the role of Chief Product Officer at OpenAI, which he found to be one of the most interesting and impactful roles, with many challenges to figure out, including building a product with a constantly evolving technology base, as computers can do something new every two months that they have never been able to do before in the history of the world (1m14s).
- Kevin's friends and team generally reacted with excitement to his new role, and he has been having a blast working on the inside as AI gets developed (1m18s).
Mike Krieger's Move to Anthropic and His Experience
- Mike Krieger, the founder of Instagram, joined Anthropic as Chief Product Officer, and people's reactions to the news varied, with some thinking it made sense, others wondering why he would take the job, and some being impressed that Anthropic could hire the founder of Instagram (2m21s).
- Mike couldn't resist the opportunity to work on something new and was drawn to the company's research-driven approach, and he has been enjoying learning about enterprise and serving customers different from those he worked with at Instagram (2m37s).
- Mike has been surprised by the childish delight he feels in learning about new things, such as enterprise and research-driven organizations, and he appreciates the opportunity to have a different experience every year, as he had vowed to do when he was 18 (3m31s).
The Nature of Enterprise Sales and Customer Feedback
- Enterprise sales have a different pace compared to other sales, with a longer timeline that can take six months from the initial conversation to deployment, requiring adjustment to different timelines (4m5s).
- The feedback and engagement from enterprise customers can be more rewarding, as they have a financial incentive to provide honest feedback on the product's performance (4m31s).
- In enterprise sales, the focus is not just on the product, but also on the buyer's goals, and building a great product does not necessarily guarantee success (5m2s).
- Enterprise customers may have specific requirements, such as advance notice of product launches, which can be challenging to accommodate (5m29s).
Product Development at OpenAI and the Role of Model Capabilities
- At OpenAI, they have multiple products, including consumer, enterprise, and developer products, which can be managed simultaneously (5m44s).
- Instincts can be helpful in product development, but only in about half of the job, especially when the product is in the final stages of development (5m53s).
- The beginning of product development can be uncertain, with unknown capabilities and emergent properties of models, requiring a wait-and-see approach (6m31s).
- The product development process can be influenced by the capabilities of the model, and the product that is built will depend on the model's performance, which can vary from 60% to 99% (7m1s).
- The research team's progress is regularly checked to determine the model's capabilities and potential applications (7m18s).
- Model training is a research process where the outcome is not always certain, making it exciting and stochastic, similar to the experience of working at Instagram during Apple's WWDC announcements, where a new feature could either be awesome or cause chaos, but in this case, the disruption comes from within the company (7m22s).
- The cycle of discovering new capabilities and planning for the next set of features is challenging when the outcome is uncertain, but it's possible to plan by squinting at the advancements in intelligence and building products around expected capabilities (8m2s).
Approaches to Product Development with Evolving AI Models
- There are three ways to approach this: watching the advancements in intelligence, deciding on product capabilities and fine-tuning with research teams, and co-designing and co-researching with the actual research teams (8m29s).
- Embedding designers early in the process is crucial, but it's essential to understand that the output of experimentation should be learning, not perfect products, and partnering with research should lead to demos or informative things that spark product ideas (9m4s).
- Research is both product-oriented and academically focused, and sometimes, new capabilities are discovered by chance, leading to unexpected opportunities (9m32s).
- When investing in new capabilities, it's essential to consider whether a model can be useful even if it's only 60% successful at a task, especially if the task is valuable and important (10m13s).
- Evaluating progression on a task and deciding what to prioritize involves considering the importance and value of the task, even if the model is not 99% successful (10m30s).
- The burden of product design is to make AI models work gracefully, even when they're not perfect, and to expect human involvement in the loop, especially when models are only 60% right, which can still be valuable for users (10m40s).
The Importance of Imperfect AI Models and Real-World Testing
- GitHub Co-Pilot, an AI product that assists with coding, was launched with a model that wasn't perfect but still provided significant value by getting the code partially correct, allowing users to edit and complete it (10m59s).
- Similar experiences will occur with the shift towards agents and longer-form tasks, where models may not be perfect but can still save users time and be valuable, especially if they can understand their limitations and ask for help (11m45s).
- The 60% benchmark is not a fixed number, but rather a rough estimate, and AI models often perform well on some tasks and poorly on others, making it essential to design for these variations (12m9s).
- Pilot programs with customers have shown that AI models can receive vastly different feedback, with some companies finding them highly effective and others finding them less useful, highlighting the importance of real-world testing (12m27s).
- The effectiveness of AI models can be influenced by various factors, including custom data sets, internal use cases, and prompting styles, which can lead to unexpected results when deployed in the real world (12m58s).
Evaluating and Improving AI Models
- Current AI models are not limited by their intelligence but rather by their evaluation methods, and they can be taught to perform better on a wider range of tasks with proper training and evaluation (13m13s).
- The lack of evaluation methods has hindered the development of AI models, and it's essential to establish clear success metrics to improve their performance (13m47s).
- The problem often solved involves determining what success looks like for a task and iteratively improving it, with tools like Claud able to automate evaluations and grading, but requiring input on what success entails (13m51s).
- At Anthropic, the interview process involves making candidates improve a prompt from a "crappy eval" to a good one, showcasing their thought process, as the company believes writing evaluations is a crucial skill for product managers (PMs) (14m23s).
- There is a lack of talent with this skill, and the company is trying to teach people how to write evaluations, considering it a core skill for PMs (14m31s).
The Changing Role of Product Managers in the Age of AI
- The job of a PM in 2024-2025, building AI-powered features, is looking more like the role of research PMs, who work on model capabilities and development, rather than product surface PMs or API PMs (14m55s).
- The quality of a feature is now gated on how well PMs have done evaluations and prompts, making the PM definition more meritorious (15m20s).
- Anthropic set up a boot camp to teach PMs how to write evaluations and the difference between good and bad evaluations, but still needs to iterate and improve (15m29s).
- To develop intuition for getting good at evaluations and iteration, one can use the models themselves, asking for sample evaluations and learning from the results (16m3s).
- Looking at data and examining cases where models fail is also crucial, as it can reveal issues with the grader rather than the model itself (16m27s).
Challenges and Evolution of AI Model Evaluation
- Every model release has a model card, and some model evaluations have shown that even the golden evaluations can be improved (16m47s).
- Evaluating the performance of AI models is challenging, and even grading them is difficult, so it's essential to look at the actual answers and evolve the evaluation methods as the models improve (16m53s).
- As AI models move towards longer-form and more agentic tasks, evaluation will become more nuanced and personalized, requiring a softer grading approach (17m18s).
- The concept of capabilities in AI models may evolve to resemble a career ladder, with evaluation resembling performance reviews that assess whether the model meets or exceeds expectations (18m8s).
- The increasing ability of AI models to beat humans at certain tasks raises questions about the role of humans in writing evaluations and the potential need for new evaluation methods (18m36s).
Skills and Adaptation for Working with AI
- To effectively work with AI models, product people should learn to write evaluations that assess the models' skills and abilities (18m52s).
- Prototyping with AI models is an underused skill that can be useful for quickly testing and evaluating different ideas and approaches (19m1s).
- The use of AI models will push product managers to go deeper into the tech stack and develop a deeper understanding of the technology (19m46s).
- The skills required to work with AI models will continue to evolve over time, and product people should be prepared to adapt and learn new skills (19m51s).
- Product managers (PMs) do not need to be researchers, but having an appreciation for and understanding of how AI works can be beneficial in building products that utilize AI (20m4s).
Building Products with Stochastic AI Systems and User Feedback
- AI systems are stochastic and non-deterministic, making it challenging to design products where the outcome is not entirely predictable (20m22s).
- To address this challenge, PMs need to establish feedback mechanisms to understand when the model is not working as intended and collect feedback rapidly (20m35s).
- This requires a different set of skills, as the traditional bug report is no longer applicable, and PMs need to understand the output of the AI across multiple outputs and users (20m51s).
- Adapting to non-deterministic user interfaces is a new challenge, and even tech-savvy individuals are still adjusting to this new paradigm (21m8s).
User Research and Adapting to AI-Powered Products
- Building products with AI requires considering the user's perspective and understanding how they will interact with the product, which can have both positive and negative consequences (21m44s).
- Conducting user research is essential in understanding how users interact with AI-powered products, and it can be surprising to see how users react to new features and the model's output (22m11s).
- PMs need to be prepared to let go of control and be flexible when working with AI, as the outcome is not always predictable (22m40s).
- The development of AI products is happening rapidly, and PMs and technical people need to develop intuition for how to use them effectively (22m55s).
Rapid Technological Advancements and User Education
- The rapid advancement of technology, such as ChatPT, has led to a situation where people quickly adapt to new innovations, and what was once considered "magic" becomes outdated in a short period, with the current state of technology expected to be seen as inferior in 12 months (24m24s).
- The speed of adaptation is also influenced by people's excitement and understanding that the world is moving in the direction of technological advancements, making it essential to make the best possible progress (24m43s).
- To address the challenge of educating end-users at scale, efforts are being made to make products more educational, such as providing information about the product itself and its features, which was not done initially (24m55s).
- User research has shown that people want to know how to use the product, and providing clear instructions and documentation can help solve UI problems and user confusion (25m14s).
Educating Users in Enterprise Settings and Empowering Power Users
- The approach to educating users is different in an Enterprise setting, where there is a status quo for how things are done, and organizational processes need to be considered when introducing productivity improvements or new technologies (25m47s).
- In the Enterprise context, power users are often early adopters who are familiar with technology, but there is also a long tail of users who may need more guidance and education on how to use new products and features effectively (26m8s).
- Non-technical users are being exposed to chat-powered LLMs for the first time, and it's essential to learn from these experiences to teach the next 100 million people how to use these UIs effectively (26m16s).
- Power users within organizations are creating custom GPTs to make AI more accessible and valuable for those who might not know how to use it otherwise, and these power users can act as evangelists (26m50s).
- The organizations mentioned are comprised of power users who are living in a "pocket of the future" and are finding innovative ways to utilize AI (27m16s).
Internal Use Cases of AI within Organizations
- Internally, the organizations are using AI to automate tasks, such as ordering pizzas, and are exploring various use cases, including UI testing and data manipulation (27m28s).
- AI is being used for UI testing, which is typically challenging and brittle, but early signs indicate that it works well for testing whether a UI functions as intended (28m12s).
- The organizations are also exploring the use of AI for agentic tasks that involve data manipulation, such as automating repetitive tasks and filling out forms (28m38s).
- The goal is to automate "drudgery" tasks, allowing humans to focus on more creative and high-value tasks (28m55s).
Workflows and Orchestration between Models
- Many sophisticated customers and internal teams are experimenting with workflows and orchestration between models, utilizing each model for its strengths, such as reasoning, but also acknowledging limitations like time to think and multimodality (29m9s).
- Reasoning in this context refers to the ability to form hypotheses, refute or affirm them, and continue reasoning, similar to how humans solve complex problems or make scientific breakthroughs (29m52s).
Scaling Intelligence and the Evolution of Reasoning in AI Models
- The concept of scaling pre-training is well-known, where models like GPT2, 3, 4, and 5 are trained on increasingly larger datasets, resulting in smarter models, but with limitations, such as system one thinking, which provides immediate answers without much thought (29m59s).
- In contrast, the new approach to scaling intelligence, as seen in models like 01, involves doing it at query time, allowing the model to pause, think, and reason before providing an answer, similar to human problem-solving (30m52s).
- This new approach has the potential to revolutionize problem-solving, as models can think for extended periods, refining their answers, and can be used in various applications, including cybersecurity, where models can be fine-tuned to work together to achieve precise results (31m43s).
- The use of models in concert with each other enables them to check each other's outputs, ensuring more accurate results, and can be applied to various tasks, such as finding and fine-tuning models to be good at specific tasks (32m32s).
- The current state of this new approach to scaling intelligence is still in its early stages, similar to the GPT1 phase, but it has the potential to significantly impact various fields and applications (31m53s).
- Models will be able to realize when something doesn't make sense and ask to try again, providing more value in specific use cases and orchestrations of models working together to accomplish complex tasks (32m40s).
The Future of AI: Proactive, Asynchronous, and Interactive Models
- The future of AI may involve models becoming more proactive, such as monitoring emails and spotting interesting trends to provide users with proactive recaps and research (33m47s).
- Another aspect of the future of AI is being more asynchronous, allowing users to expand their time horizon and not expect immediate answers, enabling them to work on other tasks while the model is processing (34m20s).
- This asynchronicity will allow users to ask more complex questions and tasks, such as fleshing out a mini project plan, fixing bugs, or adapting a product requirement document (PRD) for new market conditions (35m4s).
- The models are expected to get smarter at an accelerating rate, which will contribute to the development of these capabilities (35m28s).
- A key aspect of the future of AI is seeing models interact in various ways, enabling more complex and powerful applications (35m35s).
Advancements in Voice Mode and Natural Interactions with AI
- Humans interact with AI systems mostly through typing, but advancements in voice mode are changing this, allowing for more natural interactions like speaking and seeing, with the potential to become commonplace fast (35m42s).
- The launch of advanced voice mode has enabled users to have conversations with people who speak different languages, acting as a universal translator, and has the potential to increase people's willingness to travel to new places (35m54s).
- The combination of voice mode and other AI capabilities is creating new experiences, such as young people using voice mode to pour their hearts out and interact with AI in ways that are becoming increasingly natural (37m0s).
- The digitally native generation is growing up with the expectation that AI will be able to understand and interact with them in various ways, including voice conversations (37m17s).
- Children as young as 5 and 7 are already interacting with AI systems like ChatGPT, asking it bizarre questions and having weird conversations, and are perfectly happy talking to an AI (37m35s).
- Children are also using AI to create their own entertainment, such as telling stories and asking AI to create images in real-time, showcasing a new way of creating and interacting with content (38m14s).
Developing Empathy and Understanding Nuances in AI Interactions
- The most surprising behavior seen in AI products recently is the development of a nuanced understanding of the AI model and its capabilities, with users forming a kind of two-way empathy and befriending the AI (38m38s).
- Users are also noticing differences in the behavior of new AI models, such as feeling smarter but more distant, and are developing a relationship with the AI based on its nuances (39m0s).
- Developing AI products requires empathy, as they involve shipping intelligence and empathy, which are key components of interpersonal relationships, making it essential to consider how users will adapt to and interact with these products (39m9s).
The Personality of AI Models and User Preferences
- The personality of AI models is crucial, and there are interesting questions around how much they should customize versus having a single personality, such as OpenAI and Claude having distinct personalities (39m40s).
- Users may choose to use one AI model over another based on their personality, which is a human-like preference, as people tend to be friends with those they like and have an affinity for (40m1s).
- A recent experiment where an AI model described users based on their past interactions went viral on Twitter, showcasing how people are starting to interact with AI models in a more personal and human-like way (40m13s).
- This experiment demonstrated how AI models can be seen as entities that users can interact with, and their reactions to these interactions can be fascinating and provide valuable insights (40m39s).
Conclusion: Perspectives on the Future of AI
- Kevin Weil and Mike Krieger shared their perspectives on the future of AI and its development, providing a glimpse into the potential of these technologies (40m46s).