Arthur Mensch: Open vs Closed - Who Wins and Mistral's Position | E1146
29 Apr 2024 (11 months ago)
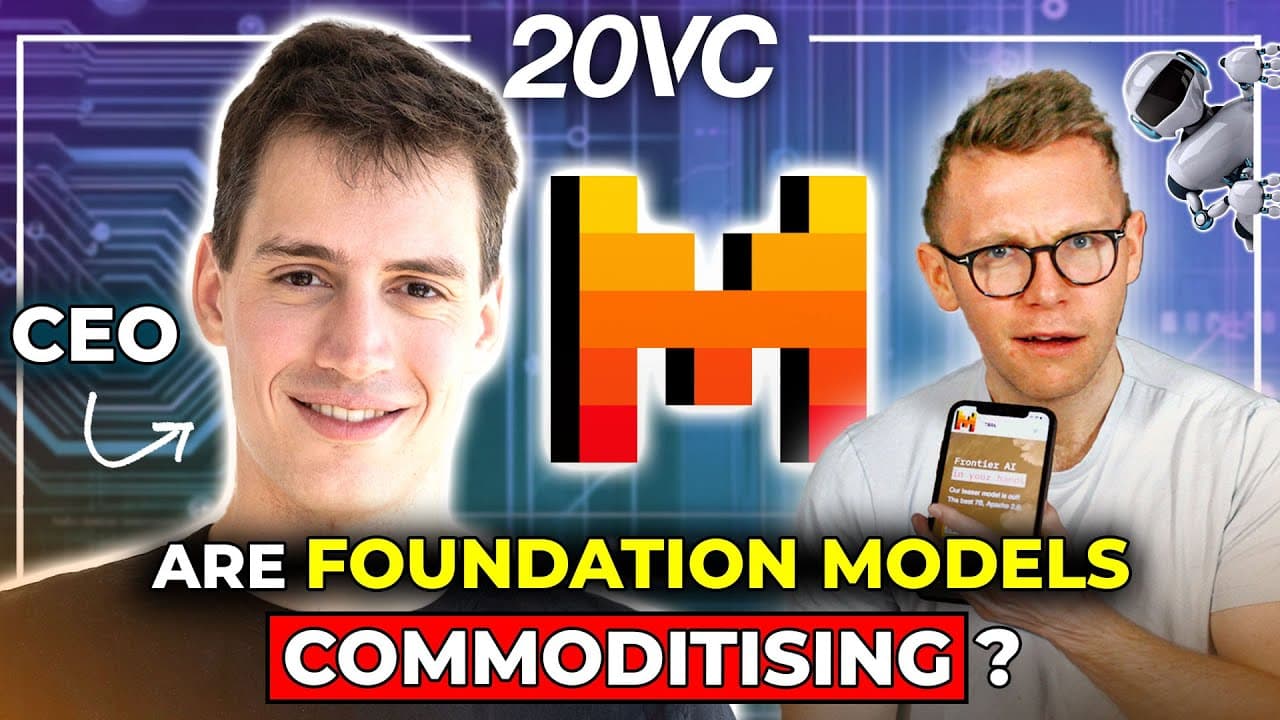
Intro (0s)
- Arthur Mensch and the host discuss fundraising for startups.
- The biggest barrier to scaling up is the lack of computing power.
- Mensch explains that it's not possible to raise $2 billion in a Series E round in the current economic climate.
- Mensch discusses the debate between open and closed systems in the tech industry.
- He argues that open systems are more innovative and efficient than closed systems.
- Mensch gives the example of Mistral, a company that is building an open-source platform for AI.
- He believes that Mistral has the potential to revolutionize the AI industry.
Background (47s)
- Arthur Mensch co-founded MRA after working at DeepMind, where he learned that smaller, uncoupled teams are more efficient than larger ones.
- Mensch's first exposure to AI and machine learning was in 2013 when he saw Andrew Ng flying a helicopter backward using a neural network.
- The MRA 7B model was popular because it was one of the first large language models to be released and showed strong performance on various tasks.
- The compression of models revealed a lot of slack, filling a gap in the efficiency to performance space.
- The 7B model size allows efficient running on devices like MacBooks and smartphones, generating curiosity and adoption among casual developers.
- The success of the 7B model taught the team that there was more interest in efficiency than scale, leading them to focus on very efficient models like Mix 867B and Mix 822B.
Efficiency vs. Scale in Model Development (7m8s)
- Scale matters in model development as it allows for more compressed models with increased training compute.
- However, scale alone is not sufficient, and proper data, training techniques, and efficiency gains without additional compute are also crucial.
- There is still potential for significant efficiency improvements, but it requires research and experimentation.
- The end state for the model landscape is expected to involve more developed developer platforms that enable customization, low-latency models, evaluation, and improvement over time.
- Models will serve as a starting point for AI application developers, surrounded by tools and a lifecycle management platform.
- Differentiation in AI applications will come from the data, user feedback, and intelligence applied to specific tasks, rather than from general-purpose models.
Challenges & Opportunities for Improving Model Quality (10m21s)
- Data quality, not compute, is the main constraint on model quality.
- Verticalized models built by application makers achieve low latency and high performance on specific tasks.
- Tools are needed to allow developers to create customized models without expert knowledge.
- The value of models is increasing while their price is decreasing, leading to uncertainty about the growth of the application layer.
- Mistral is an open-source platform that allows developers to own and modify AI models, fostering freedom and wide distribution of generative AI.
- AI developers prioritize cost, customization, portability, and data control when making decisions.
- Brand is crucial in the AI domain as it establishes trust, and Mistral's open-source approach has contributed to building a recognized brand.
- The decreasing cost of creating applications due to the capabilities of AI models is expected to impact the dynamics of marginal cost and margin in the future.
- Creating a relevant foundational model company requires dominating cost efficiency, performance, and brand recognition, which is challenging due to the few companies that are currently well-positioned.
- The unlimited availability of open-source LLMs shifts the value from the model itself to the platform and customization.
The Decision to Close Some Models (24m53s)
- OpenAI started as a very open-source organization.
- Now, some models are open-source while larger ones are closed.
- The decision to close some models was made to:
- Grow the business by selling access to the closed models.
- Cement strategic relationships with cloud providers.
- Continue to be a leader in the open-source AI field.
- Have unique assets that can be licensed.
- Provide a unique platform for developers.
Balancing Research & Sales Teams (25m53s)
- Create empathy between the science team and the business team.
- Science team should have direct exposure to the product and business team to understand model failures and improvements.
- Go-to-market team needs strong enablement to understand the technical sales motion and how to communicate the value of the product to customers.
- Science team has longer cycles while the go-to-market team has shorter cycles, but recruiting people with both technical and business interests can help bridge the gap.
- In the enterprise space, brand matters and existing agreements with companies like Microsoft can be a challenge.
- Open source models can be a shortcut to distribution and create demand.
- Some enterprises are already using open-source models in production, but they lack tooling for managing load balancing and customizing models.
- The most technically savvy enterprises are ready for open-source models, but there is room for improvement in tooling to widen adoption.
- Enterprises should stop thinking about how to change all their products using AI as a premise and instead focus on how to completely change the way they operate their core business.
- Generative AI should be seen as a way to change the core business rather than just increasing productivity in specific tasks.
- Enterprises should customize models heavily to create differentiation in the market.
The Readiness of Enterprises for AI Adoption (30m6s)
- There is a concern that the adoption of generative AI solutions in Europe may be overestimated in the near future but underestimated in the long term.
- Enterprises in Europe are slower to adopt new technologies compared to the US, but there is some executive support for pushing generative AI solutions.
- The challenge for enterprises is to identify specific AI applications that can bring value to their business and prioritize those.
- Generative AI is moving into core budgets for customer support but is still in the experimental stage for other functions and core applications in industries like telecom and healthcare.
- Mistral is focusing on providing best-of-class models that can solve specific use cases rather than competing solely on compute power.
- The biggest barriers to scaling generative AI models include delays with compute providers and the need to hire and train specialized AI scientists.
- Arthur Mensch discusses the concept of open vs. closed positions in business and who wins in each scenario.
European vs. US Investors (34m57s)
- Arthur Mensch, CEO of Hugging Face, discusses the challenges and opportunities for European AI companies.
- Mensch emphasizes the importance of founders maintaining control of their companies, especially in the rapidly changing field of AI.
- Europe has the potential to become a major player in AI due to its talent, capital, and market opportunities.
- However, Europe lacks a strong VC system and relies heavily on US funding.
- Mensch is optimistic about the future of AI in Europe and believes that the region can build a strong ecosystem and compete with the US over time.
- Hugging Face has a team in the US to access specialized AI talent, particularly senior AI scientists.
- France, Poland, and the UK have a wealth of AI talent, which is a strength for the region.
Does the Source of Funding Matter for Scaling Constraints? (40m18s)
- European growth funds lag behind US growth funds due to political decisions, capital supply, and the belief in a competitive European ecosystem.
- Scaling a company quickly presents organizational challenges, including communication, time management, and maintaining team stability.
- Product development should be prioritized before go-to-market development.
- Startups require ongoing fundraising to support scaling and staying relevant, with a focus on research and development.
- Competitors in the landscape, such as Cohere, OpenAI, Entropic, and Google, are respected for their work towards similar goals.
- Starting a new foundation layer business is not recommended due to the risk of new competitors emerging and surpassing existing ones.
Quick-Fire Round (46m45s)
- Arthur Mensch's biggest concern is global warming and believes AI can be part of the solution.
- He has changed his mind on management premises, finding transparent feedback to be very useful for company growth.
- The most unexpectedly challenging element in scaling Mistral was the high demand and unexpected brand success.
- To calm down, Arthur runs and cycles, and tries to take care of his daughter.
- As a new father, he realized the amount of energy needed to care for a small child.
- AI is changing the way people work, requiring more creativity and value beyond what can be automated.
- This structural change in the job market necessitates adaptation in training and education.
- Mensch believes fears of job replacement by AI are exaggerated, as some jobs will be displaced while new ones open up.
- The speed of society's elevation toward higher abstraction levels is unmatched in history, making adaptation more challenging.
- In 10 years, Mensch envisions Mistral with successful commercial and open-source models, along with a strong developer platform for creating AI applications.