Arvind Narayanan: AI Scaling Myths, The Core Bottlenecks in AI Today & The Future of Models | E1195
28 Aug 2024 (6 months ago)
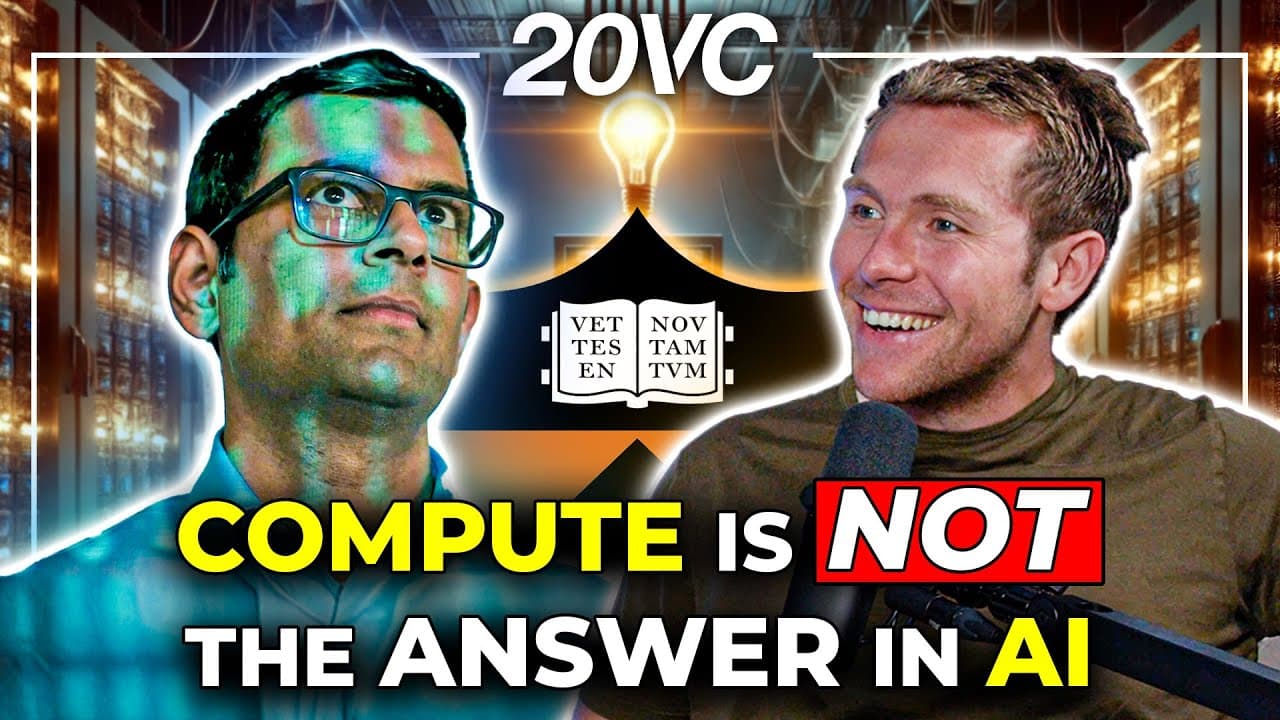
Intro (0s)
- The availability of data is becoming a constraint in the development of larger and more powerful AI models. (12s)
- Existing models are already being trained on almost all accessible data that organizations can acquire. (16s)
- While increased computing power remains beneficial, its impact might be diminishing compared to the past. (21s)
AI Hype vs. Bitcoin Hype: Similarities & Differences (1m18s)
- AI developers believed that AI was so unique and powerful that they could simply release models to the public and users would determine their applications. (3m24s)
- This belief led AI developers to neglect fundamental tech principles such as product development, user needs, and market fit. (3m34s)
- AI companies mistakenly convinced themselves that they were exempt from the established norms of the tech industry. (3m43s)
The Misalignment Between Compute & Performance (3m49s)
- The most significant difference between GPT 3.5 and GPT 4 was the size of the model. (4m32s)
- Data is becoming a bottleneck, limiting the performance increase achievable by simply increasing compute. (5m3s)
- While there are unmined data sources like YouTube, their size in tokens is not as significant as it appears, and they are unlikely to lead to the emergence of significantly new capabilities in language models. (6m22s)
Synthetic Data (8m10s)
- Synthetic data is currently used to address quality issues in existing training data, such as augmenting data for languages with limited resources. (8m20s)
- Another proposed use of synthetic data involves training a model on a large dataset and then using the model to generate even larger datasets to train subsequent models. (8m55s)
- However, this approach is unlikely to be effective because data quality is more important than quantity, and synthetic data generated in this way may lack the richness and diversity of real-world data. (9m10s)
Creating Effective Agents Despite Incomplete Data (9m30s)
- A significant challenge in developing effective AI agents is the lack of codified data representing the tacit knowledge and thought processes within organizations. (9m38s)
- The current success of large language models (LLMs) stems from learning from readily available web data, but this approach is limited when dealing with tasks requiring tacit knowledge or skills not explicitly documented. (10m22s)
- Deploying AI systems within organizations and enabling them to learn from interactions and feedback loops is crucial for addressing tasks that require tacit knowledge, similar to the gradual rollout and data collection process observed with self-driving cars. (11m21s)
Why Is the AI Industry Shifting Toward Smaller Models (12m0s)
- The adoption of large language models is often bottlenecked by cost rather than capability. (12m23s)
- Smaller models, especially those that can run on a user's device, offer advantages in terms of cost, privacy, and new possibilities. (12m54s)
- While Moore's Law suggests that computing costs will decrease, the demand for AI applications is also expected to increase, potentially offsetting cost reductions. (13m49s)
The Growing Gap Between AI Models & Compute Capabilities (16m31s)
- The rapid development of new AI models is outpacing the development of new hardware and compute capabilities. (16m43s)
- While hardware cycles are improving rapidly, the exponential growth of both models and hardware will eventually taper off. (17m46s)
- Evaluating large language models (LLMs) is challenging because benchmarks often fail to capture real-world performance and models can be contaminated by training data. (18m26s)
Predictions on the Timeline for AGI (19m44s)
- People have been predicting that artificial general intelligence (AGI) is imminent since the earliest days of AI, but the complexity of the problem has repeatedly been underestimated. (21m0s)
- It is possible that large language models will become commoditized, and most of the value in AI will be created in a layer above the models, such as AI agents. (25m35s)
- Regulators should pay attention to the potential for market concentration in the AI industry, as a few companies with large cash flows could end up controlling the foundational models. (26m30s)
Policy Proposals for U.S. and European AI Regulation (27m0s)
- AI regulation is a misnomer, and it is more accurate to regulate harmful activities rather than AI itself. (28m16s)
- An example of this is the FTC's decision to ban fake reviews, regardless of whether they are generated by AI or humans. (28m14s)
- It is generally advisable to allow innovation to flourish and then regulate based on observed harms, but there are some existing harms, such as the use of AI for non-consensual deepfakes, that require immediate action. (29m26s)
AI & Deepfakes: The Risk of Discrediting Real News (29m29s)
- The most significant danger posed by AI is not that people will believe fake news, but rather that it will lead to distrust in real news. (29m40s)
- Misinformation is not primarily caused by AI, but rather reflects existing societal problems and the human tendency to seek information that confirms pre-existing beliefs. (31m45s)
- While AI has made it easier to create misleading content, addressing the spread of misinformation requires focusing on social media platforms and broader societal issues rather than solely on AI technology. (33m47s)
Revolutionising Healthcare with AI in Your Pocket (35m59s)
- The medical system has been an enthusiastic adopter of technology, including AI, with examples like CAT scans being used for diagnosis. (37m16s)
- Generative AI has garnered interest in the medical field for its potential in diagnosis, summarizing medical notes, and other applications. (37m33s)
- While AI can be a valuable tool for learning, the social aspect of learning remains crucial for the majority of learners, and AI is unlikely to replace traditional learning methods soon. (39m12s)
Is AI Job Replacement Fear Overhyped or Real? (40m29s)
- Fears about AI replacing jobs are currently exaggerated. (40m44s)
- AI automates individual tasks within a job, not entire jobs. (41m18s)
- While some occupations, like translation and stock photography, have been impacted by AI, most jobs involve a variety of tasks that are unlikely to be fully automated in the near future. (41m40s)
AI's Potential as a Weapon (41m46s)
- AI is not a weapon itself, but a tool that could be used by adversaries to enhance their capabilities, such as identifying vulnerabilities in cybersecurity. (42m5s)
- Attempting to restrict access to AI to prevent malicious use is unlikely to be effective, as AI models are becoming increasingly accessible and powerful, even on personal devices. (42m37s)
- Instead of focusing on restricting access, the focus should be on leveraging AI for defensive purposes, similar to how automated tools are used in cybersecurity to identify and fix software vulnerabilities. (43m34s)
Quick-Fire Round (46m19s)
- AI leaderboards are no longer useful because of the growing gap between benchmarks and real-world applications. (46m30s)
- There is a need for increased transparency in AI development, prioritizing public understanding over commercial interests. (47m1s)
- The future of AI agents holds potential for automating tasks in a nuanced way, such as booking flights or building apps based on user commands. (47m19s)