Beyond Human: HolmesGPT and the Future of AIOps
23 Nov 2024 (25 days ago)
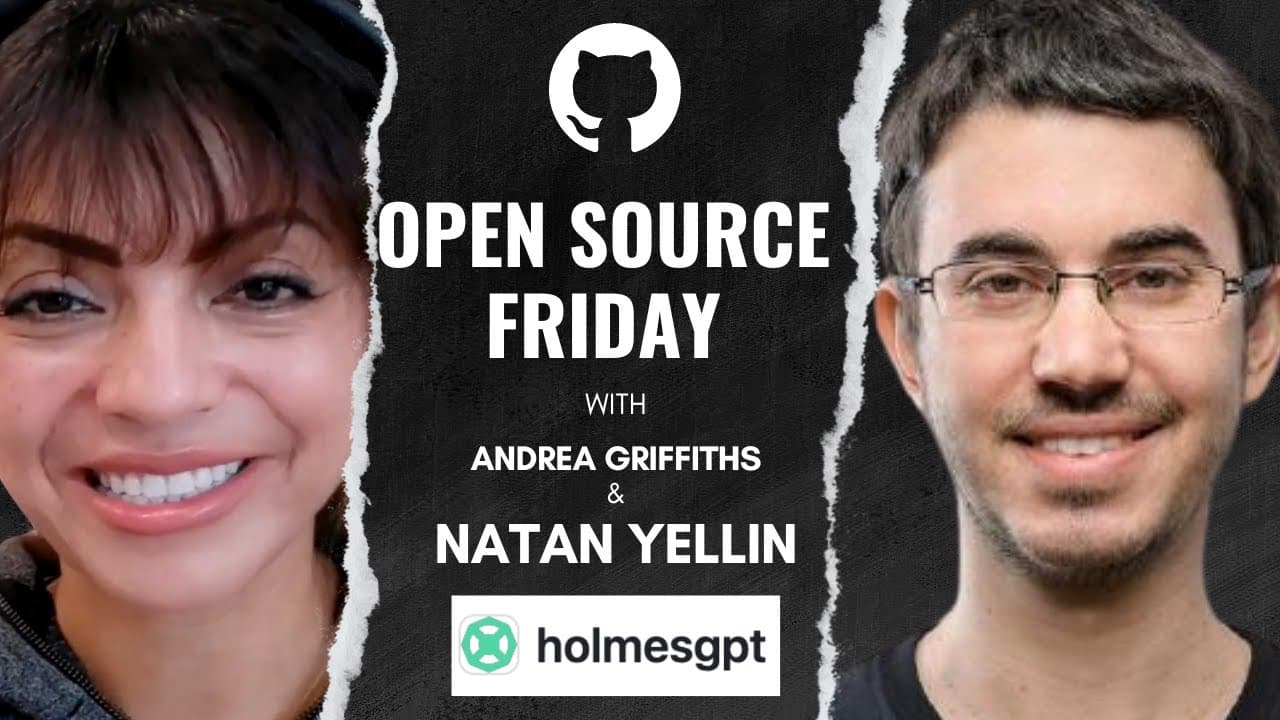
Introduction and GitHub Events (Open Source Focus)
- The GitHub Universe conference was a huge success, with an open source zone featuring over 10 projects, workshops, and talks, making it a great conference for open source supporters to attend in the future (2m17s).
- The GitHub Constellation event in South Africa focused on applying open source technologies and artificial intelligence to social impact projects, hosting a hackathon with 43 projects submitted, all aiming to create powerful solutions for local impact (3m27s).
- One of the projects from the hackathon, "Accessibility," used open source to help blind and deaf people navigate the streets of Johannesburg better, showcasing the potential of open source in creating impactful solutions (4m2s).
- Today's discussion is about a project called HolmesGPT, which builds chat GPT-like capabilities for observability and cloud monitoring, aiming to provide real solutions that don't require human monitoring (4m34s).
Introducing HolmesGPT and its Purpose
- Nathan Yellen, a seasoned engineer and CEO of Robosa, is leading the development of HolmesGPT, bringing his technical expertise in security solutions and DevOps to the project (4m40s).
- The inspiration behind HolmesGPT was to address the lack of real solutions for cloud monitoring and observability that don't require human monitoring, making it an important part of infrastructure and scale in projects (5m55s).
- The primary goal is to augment human capabilities, not replace them, by making engineers and teams more efficient and effective in their work (6m5s).
- AI tools, such as ChatGPT, can be useful in various cases, like generating new ideas and recipes, but they have limitations and should be used appropriately (6m22s).
- The aim is to provide engineers with tools that enable them to work faster and better, without replacing them (6m53s).
- The ecosystem of AI tools, including Kate's GPT and other tools, will be discussed, as well as their applications and limitations (7m1s).
Enterprise AI Adoption and the Need for Observability Solutions
- Enterprise adoption of AI tools is a growing trend, with a focus on "bring your own LLM" (BYOLLM), where companies use AI tools that are Enterprise-friendly and do not require sending data to external sources (7m21s).
- The discussion will cover the "why" behind using AI tools for observability and chat, and why ChatGPT alone is not sufficient for these tasks (7m42s).
- A common scenario in companies is receiving alerts or calls about issues with production systems, often at inconvenient times, and the challenge of investigating and resolving these issues (8m23s).
- In many cases, the data needed to solve the problem already exists within the company, and the key is to find and use the right data and tools to resolve the issue quickly (9m5s).
- Many companies struggle with identifying and solving problems efficiently, often due to a lack of skilled engineers or the time it takes for junior engineers to develop their troubleshooting skills (9m31s).
- Junior engineers face high expectations to be effective from day one, especially with the increasing use of AI tooling, and team leads want them to be able to investigate and solve problems quickly (10m8s).
HolmesGPT's Goal: Augmenting Engineers, Not Replacing Them
- The idea is to help junior engineers find the right information quickly, similar to autocomplete in Google searches, to make them more effective and efficient in their roles (10m45s).
- Even skilled engineers can benefit from having the right data surfaced faster, and tools like HolmesGPT can help with this (10m53s).
- An example of this is the integration with PagerDuty, which allows for automated investigations and surfaces relevant data for engineers to quickly investigate and solve incidents (10m56s).
- This can lead to faster resolution times, happier customers, and a more efficient engineering department (11m31s).
- The goal is to augment engineers with tools, allowing them to focus on developing features rather than doing on-call work, and making junior engineers heroes by giving them the tools they need to succeed (11m43s).
- The solution aims to address problems identified through personal experiences, customer tickets, and the need to improve life resolution times for issues (11m57s).
- The solution itself will be discussed in more detail, with a focus on what doesn't work and how the proposed solution addresses these issues (12m34s).
Troubleshooting Scenarios and the Limitations of Chatbots
- A scenario is presented where an e-commerce shop's checkout page is stuck, resulting in lost revenue, and the goal is to troubleshoot the issue (12m45s).
- The problem cannot be solved by simply asking a chatbot like ChatGBT, as it lacks access to the specific data and context of the issue (14m0s).
- ChatGBT can, however, provide general steps for troubleshooting, such as checking pod events, inspecting logs, and inspecting pod ammo (14m26s).
How HolmesGPT Works: AI-Guided Troubleshooting
- The core concept of HolmesGPT is to use an AI engine to ask for troubleshooting instructions given a specific problem, and then receive feedback and guidance on the next steps to take (14m50s).
- HolmesGPT uses a large language model to guide the troubleshooting and data gathering process, allowing it to provide more specific and relevant guidance (15m24s).
- The AI engine can be used to determine the right data to gather, gather that data, and feed it back into the system in a loop, allowing for more efficient and effective troubleshooting (15m57s).
- HolmesGPT is demonstrated in action, using the SAS platform to ask a question and receive guidance on troubleshooting an issue with an analytics exporter (16m11s).
- HolmesGPT is an AI assistant that can gather data with six tools, run commands, look at data, and provide an answer to a question, unlike traditional chat GPT which only provides a response based on the input (16m44s).
- The tool can identify the root cause of a problem, such as a crash due to running out of memory, and provide a solution to fix it (17m11s).
- The AI assistant can run commands and feed the data back in for analysis, providing a more comprehensive answer to the user's question (17m28s).
- The tool knows which observability data to collect based on its configuration, but the exact mechanism is not specified (17m38s).
Building HolmesGPT: Tradeoffs and Benefits
- When building a system like HolmesGPT, there are tradeoffs to consider, such as giving access to all data or limiting it, and ensuring the system is both functional and secure (17m54s).
- The benefits of using a tool like HolmesGPT include reducing downtime, which can have a significant impact on a business's bottom line, and improving the developer experience, which can increase productivity and efficiency (18m26s).
- Companies employ hundreds or thousands of developers, and saving each developer an hour or two per day can have a huge impact on the business (18m35s).
Types of AI Assistants and Their Applicability to Observability
- There are three types of AI assistants that can be built, including one that uses retrieve or augmented generation (RAG) technology, which uses a vector database to look up relevant information to a question (19m9s).
- RAG technology indexes organizational data, such as documentation and conference circles, and uses this information to provide an answer to a question (19m27s).
- The AI assistant can be configured to provide answers based on relevant articles and documentation, and can be integrated into cloud platforms like Google Cloud (19m52s).
- There are typically two types of AI agents that people often build, the first being a rag-based solution that finds relevant documentation and gives an answer based on it, but this approach doesn't work well for observability use cases due to huge amounts of dynamic data. (19m55s)
- The second type of solution is environment-aware, which involves fetching certain data in advance and including it in the question, but this approach works well only for simple things and breaks down for real-world observability questions that are multi-stage. (20m54s)
- The third approach is the agent-agentic approach, which involves an AI agent that repeatedly asks for data and gathers it in a loop, similar to how humans investigate, and this is the approach chosen for HolmesGPT. (22m38s)
- The agent-agentic approach allows for the gathering of data in stages, with the AI agent determining what type of data to gather based on the data shown so far, and this approach gives really good answers for complex observability questions. (23m3s)
- The rag-based solution and environment-aware approach are not suitable for observability use cases due to the complexity and dynamic nature of the data, and the agent-agentic approach is more effective in handling such cases. (20m34s)
- Tools like Kate's GPT use the environment-aware approach, which is good for simple things but not for complex observability questions that require looking at multiple data sources and joining between them. (22m15s)
HolmesGPT's Approach: Agent-Agentic Troubleshooting
- When asking AI a question, it doesn't answer directly, but instead suggests commands to run to gather the necessary information, and then provides the results, with the user having the option to ask follow-up questions to guide the investigation (23m26s).
- To ensure safety, the AI agent is given access to specific integrations that have been approved, allowing read-only access to the data, rather than giving it access to run any command (23m49s).
- The types of data that can be asked about are limited to those where an integration has been added or explicit access has been given, with integrations being lightweight and easy to add (24m10s).
- Out-of-the-box support is available for various data sources, including Kubernetes, AWS, Docker, Loki, Tempo, OpenSearch, ElasticSearch, Prometheus, and Grafana (24m40s).
- Users can add integrations for company-specific data sources, allowing the AI to provide high-quality results that are tailored to their specific needs (25m27s).
- To get good results, it's essential to give the AI access to the right type of data sources, allowing it to investigate and surface relevant data (25m45s).
Integrating HolmesGPT with Existing Tools and Data Sources
- Users can ask follow-up questions to guide the AI's investigation, and the AI presents the data in different ways, such as in a chat interface, to facilitate this process (26m28s).
- Servicing the right data can be achieved by guiding the system to check specific areas, such as firewall logs, when investigating network problems, and this guidance can be based on the user's own knowledge and expertise (26m58s).
- The system can be connected to specific AIOps tools, such as Prometheus, to analyze data and provide insights, and it can also read Run books and analyze data according to the instructions in those Run books (27m10s).
- The system can also integrate with incident management tools like Ops Genie or PagerDuty to read data, perform analysis, and write back the results as a comment (27m31s).
- The goal is to build a conversational ability into the system, allowing users to ask follow-up questions and engage in a discussion with the system to surface up relevant data and insights (27m44s).
- The system aims to surface up relevant data quickly, even in large datasets, and provide a starting point for investigation, rather than a final answer (27m50s).
- The system can be used to guide junior engineers through the investigation process, using knowledge and expertise from senior engineers, and can help to surface up relevant data and insights based on that expertise (28m15s).
- Senior engineers can write instructions for investigating specific problems, and the system can use those instructions to guide junior engineers through the process, reducing the need for junior engineers to remember complex procedures or ask for help (28m24s).
- Many companies already have knowledge and expertise documented in places like Confluence or Slack history, but it is often not easily accessible or readable, and the system aims to make that knowledge more accessible and usable (29m5s).
- The data needed to solve incidents is often already available in observability tools and systems, but it can be difficult to find and access, and the system aims to make that data more easily accessible and usable (29m19s).
- There are few incidents where the data needed to solve the problem is not available, and the system aims to help users find and access that data more quickly and easily (29m34s).
Real-World Example: Fiberoptic Cable Issue
- A problem was found where someone had laid down new fiberoptic cables, but one of them was faulty, and the issue was resolved by connecting two seemingly random dots, showing that often the data is there, it's just not accessible or being looked at (30m3s).
- In most cases, solving a hard problem or outage doesn't require new data, but rather making the existing data accessible or thinking to look at it (30m30s).
HolmesGPT's Core Engine and Data Prioritization
- The system can decide what to prioritize when there is conflicting data coming from different sources, and it can give priority to the more relevant data as part of the patterns of investigation (30m55s).
- The system uses an open-source core engine, which is the brain behind the operation, and it can be shown from the terminal to understand what it's doing (31m18s).
- The system can be asked questions, and it will gather the necessary data to answer the question, such as asking what pods are unhealthy in a Kubernetes cluster (31m35s).
- The system can filter through the data, look at the unhealthy pods, and give an answer, and it can also be asked follow-up questions that depend on the previous data (32m0s).
- The system can iterate through the data, asking one question, looking at the results, and then asking another question, and it can run multiple commands in parallel to gather data (33m10s).
- The system can gather data from multiple commands, analyze the results, and give an answer, demonstrating its ability to handle cascading scenarios (33m21s).
Ensuring Accuracy and Avoiding Hallucinations
- Determining a healthy cluster relies on two things: built-in awareness from the day the model was trained on, and explicit guidance provided through prompt engineering, with a focus on the latter to avoid hallucinations (34m4s).
- The model's built-in awareness is based on its training data, which in this case is a GPT 40 model trained on large amounts of internet data, giving it an idea of what an unhealthy pod might look like (34m14s).
- Prompt engineering is used to provide guidance on what tools to use to identify a healthy cluster and what baseline information to consider when running investigations (34m42s).
CLI and SaaS Platform: Accessibility and Integrations
- The CLI (Command-Line Interface) is the open-source portion of the system, which can be used to analyze Prometheus data, integrate with different systems, and write back results (35m11s).
- The CLI is designed to be usable in real-world scenarios, with its own life and the ability to hook up to different data sources on its own (35m46s).
- A SaaS platform is also available, with a free tier, which adds a user interface and features for sharing with teams and making it easier to hook up data sources (35m55s).
- The system allows users to bring their own large language model (LLM) and use it for free, with the option to use the provided LLM or hook up their own API key (36m39s).
- The system prioritizes privacy, not training on user data, and allowing users to keep their API keys private (36m54s).
- Organizations can use a large language model approved by their organization, such as AWS, Bedrock, Azure, or a private Open AI account, and hook it up to ensure data does not go through external sources (37m5s).
- Most main providers, including AWS, Bedrock, Azure, and Open AI, do not train on users' data, even on the free layer of the product (37m22s).
- The product can be integrated with various tools, such as Prometheus, to provide AI analysis of alerts and enable users to investigate issues (38m0s).
- Users can give instructions on how to investigate an issue, add a runbook, and jump to the root cause of the problem, which runs a series of investigations and provides results (38m24s).
- The product can be used to provide better Prometheus alerts in Slack with AI analysis inside the alerts, and can be hooked up to various integrations, such as AppsGenie, PagerDuty, and more (39m7s).
- Users can get AI analysis results inside AppsGenie, PagerDuty, and other tools, enabling teams to respond to issues more effectively (39m28s).
Security and Data Privacy
- The product allows users to give the AI agent access to data sources, such as Kubernetes, by providing command lines in bash and specifying temporary permissions (40m11s).
- The AI system uses pre-approved commands that are templated, allowing it to fill in the templates and run the commands without going off and running something it wasn't given permission to run (40m41s).
- The system can be given access to new data sources by adding a command that can fetch the data, such as a curl command to a specific API (41m20s).
- Sensitive data is not stored by the tool itself, and users control what they give access to and can revoke access at any time (41m40s).
- Users have complete control over the data and can use their own large language model hosting their own cloud account (41m55s).
Contributing to the Open-Source Project
- The open-source portion of the project allows contributors to add new integrations, such as the open search or elastic search integration (42m35s).
- A good place to start contributing is by adding new integrations, such as hooking up the system to data in a specific platform like Spun (42m58s).
- The project has a Slack community where contributors can ask for guidance and assistance (43m5s).
Light LLM Library: Supporting Multiple AI Models
- The system uses the Light LLM library under the hood, which allows it to integrate with other models, including GitHub models (43m57s).
- A library is used to abstract away different large language models, allowing users to bring their own models, such as AWS Bedrock, Nama 3, Watson X, Oracle models, or SAP AI Core models, and use them with Robusta (44m12s).
- The library, Light LLM, supports over 100 models, and any model it supports can potentially be used with Robusta, although there are some technicalities around which models are supported (45m10s).
- The use of Light LLM has allowed Robusta to support a wide range of AI models, which are used by customers and the open-source community (45m52s).
- The library is considered fantastic and has enabled the support of many different AI models, making it better for the whole ecosystem, vendors, end-users, and software creators (46m18s).
Impact and Case Studies
- The use of Light LLM and Robusta has led to changes in the way people work, with case studies on the Robusta website showcasing the benefits of using these tools (47m8s).
- There are stories of how the use of Robusta has improved time to remediation and other processes, with one particular story to be shared (47m41s).
- A large enterprise company, which cannot be named, had a platform engineering team that maintained a Kubernetes platform used by hundreds of developers across dozens of application teams, with the number of teams and developers growing over time (48m12s).
- Many enterprise application developers have been writing business logic in languages like Java for 20-30 years but lack knowledge about cloud and Kubernetes, creating a challenge for the platform engineering team (48m40s).
- The platform engineering team became a Q&A and support resource for developers, answering questions and resolving issues, which took away from their core engineering work (49m42s).
- By implementing Robusta and HolmesGPT, the platform engineering team was able to offload most of the support work, enabling developers to self-service and reducing the burden on the platform team (49m59s).
- The solution allowed developers to open a chat window, ask questions, and look at AI analysis and other features, reducing the need to open tickets and wait for support (50m11s).
- The implementation resulted in significant time savings for developers, improved efficiency, and allowed the platform team to focus on core engineering work, building reliable platforms (50m40s).
- This story is common among enterprise customers, where small teams or even a single person may be responsible for supporting developers, which can become an anti-pattern if it becomes too burdensome (51m8s).
- Platform engineering teams should support their customers and developers, but excessive support burdens can take away from core engineering work (51m25s).
- Working with large enterprises provides high-quality feedback on what features are missing and helps prioritize development, which is impactful when deploying solutions at a massive scale to thousands of people (51m56s).
- Some companies are adopting the open-source project and implementing it in various parts, integrating it with their existing enterprise solutions, which is exciting to see (52m17s).
- The level of mass adoption is encouraging for other companies, as it shows that if the solution works for large enterprises, it can work for smaller projects as well (52m42s).
Future Development: Remediations and Prompt Fu
- The names of companies contributing to the project are publicly available through GitHub PRs, but more companies need to come forward and share their experiences (52m50s).
- The feature feedback loop is crucial, and the next step is to focus on remediations, which is a bit trickier as it requires human-in-the-loop confirmation (53m21s).
- Remediations require doing things differently under the hood, but there are ideas on how to implement this, and the goal is to have an AI suggest remediations and a human confirm them (53m50s).
- Another open-source project, Prompt Fu, is being used as an evaluation framework to make evaluations more sophisticated and ensure that AI features are effective (54m4s).
- Evaluating AI features is challenging due to their non-deterministic nature, and it's essential to verify that the solutions shipped to customers are reliable and effective (54m50s).
- Evaluations are a crucial part of building AI software, where a set of representative questions is created to benchmark the model's performance and measure improvements over time (55m7s).
- Evaluations involve regression testing to ensure the model is not getting worse and checking if it can answer previously hard questions more accurately (55m22s).
- Evaluations provide an objective benchmark for measuring improvements in the model, and equal amounts of effort are put into evaluating the model and building it (55m49s).
Open Telemetry and Streaming Data
- A question was asked about open Telemetry outputs and streaming instead of Prometheus metrics (56m6s).
- The answer to the question is yes, and it's possible to query open Telemetry data using a command like curl, similar to how a human would do it in a terminal (56m15s).
- To query open Telemetry data, one would need to run a command with a URL, HTTP endpoint, and parameters, such as querying data for a specific tag or service (56m47s).
- The concept of querying open Telemetry data is similar to how a human would do it, and it's possible to give the AI the capability to run a command like that (58m41s).
- An example command to fetch Jaeger traces with open Telemetry data would be to run a command like "fetch Jaeger traces sent with open Telemetry" (58m48s).
- To set up a system, parameters such as the host and service name need to be specified, which can be done by parameterizing the system and putting in the required information, such as the service name and description (59m4s).
- The system can then be connected to an AI, which can be used to fetch information, such as the host and service name, and use it to query data and look for specific information (59m29s).
- If the system is not set up correctly, it will not be able to find the required information and will report back with an error message, indicating what the problem is (1h0m41s).
- To get started with a system like this, it's necessary to look underneath the open telemetry and see what needs to be plugged in, which can be a cool and informative process (1h0m50s).
Call to Action: Contributing to Open Source
- The importance of contributing to open-source projects and making that first step, such as opening a pull request or addressing a bug report, is emphasized, as it can lead to a long and rewarding journey (1h2m15s).
- The story of how someone's journey started with contributing to open-source projects at a young age, and how it led to them being able to contribute back and run a company that works with open source, is shared as an example (1h1m32s).
- The younger people on the call are encouraged to make that first step and contribute to open-source projects, even if it's just a small contribution, as it can lead to new challenges and opportunities (1h2m17s).
- The importance of taking the first step and not being afraid to try, even when unsure of what one is doing, is emphasized as a crucial aspect of personal and professional growth (1h3m2s).
- It is suggested that individuals should take an issue, spend time trying to figure it out, and not give up, even if progress is slow or difficult (1h3m33s).
- The value of persistence and always moving forward, even in the face of setbacks or failures, is highlighted as a key principle for success in life, open source, contributing, learning, and software engineering (1h3m53s).
- The idea of always getting back up and trying again, even if one encounters obstacles or failures, is repeated as a crucial mindset for achieving goals and making progress (1h4m5s).
- The speaker shares their personal experience of participating in a Google-sponsored hackathon, where they did not win but continued to work on their project, eventually leading to involvement in open source and recognition from companies like Red Hat and Canano (1h5m58s).
- The speaker's journey is cited as an example of how taking the first step, persisting, and continuing to learn and grow can lead to unexpected opportunities and successes (1h5m50s).
- The advice to put in the time and effort, even if progress is slow, and to not give up on one's goals is emphasized as a key takeaway for individuals, especially those who are younger and just starting out (1h5m30s).
Conclusion and Next Steps
- The conversation concludes with appreciation for the guest, Nathan, and his attitude towards open source, which is seen as inspiring and crucial for its sustainability (1h6m41s).
- Nathan is invited to return and demo remediation, a feature that is expected to be developed in the future (1h6m57s).
- Viewers, especially those working in enterprises, are encouraged to use the tools and augment themselves, rather than suffering, and to support open source projects (1h7m12s).
- The host thanks Nathan again and wishes him a great rest of the week, while also inviting him to come back anytime (1h7m23s).
- The host thanks the audience for staying and encourages them to check out the project, leave a star, and continue supporting open source (1h7m43s).
- The host announces a two-week hiatus due to the American holiday and Thanksgiving, but promises to resurface some of the best episodes during that time (1h7m51s).
- The host thanks the audience for their support of open source and wishes everyone an awesome weekend (1h8m5s).