Eiso Kant, CTO @Poolside: Raising $600M To Compete in the Race for AGI | E1211
07 Oct 2024 (3 months ago)
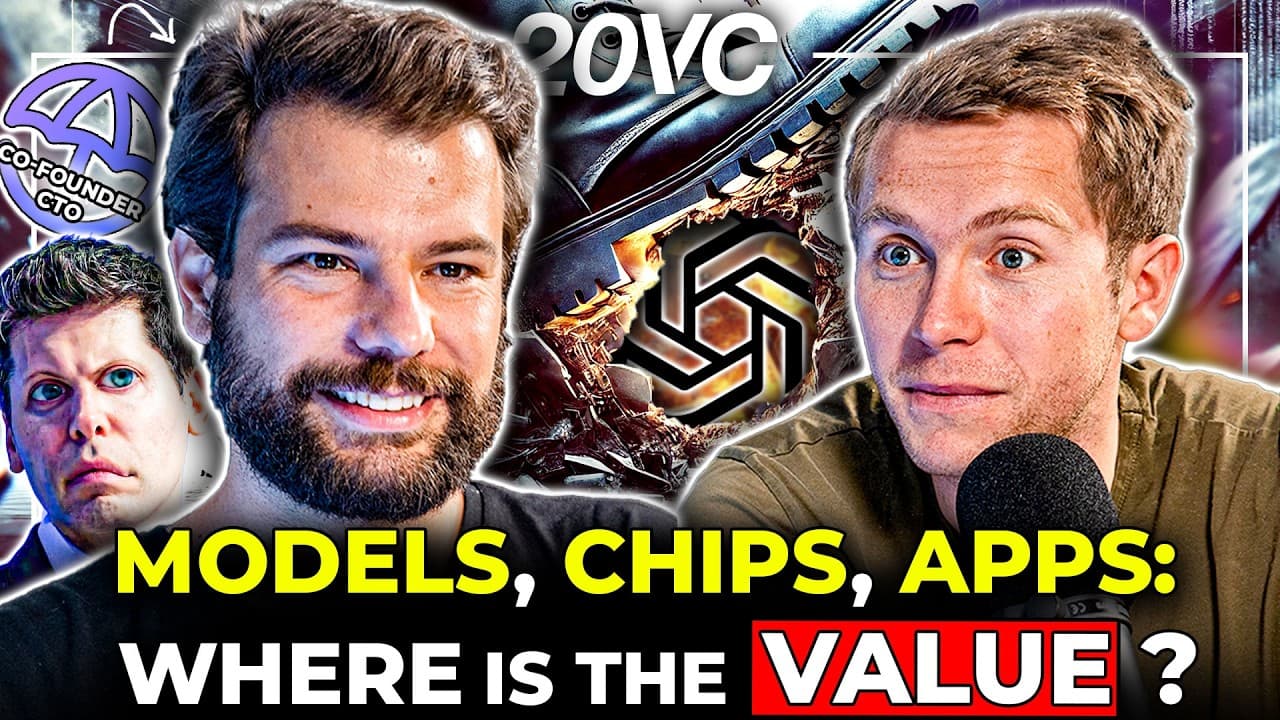
Intro (0s)
- The current moment in the development of Artificial General Intelligence (AGI) is significant and will be looked back upon in 10 years as a pivotal moment, similar to the advent of the mobile internet (6s).
- The development of AGI is a competitive race, and the latest $500 million funding round enables participation in this race (16s).
- The capabilities and readiness of the entrants in the AGI race are crucial, and there is no room for error or delay (23s).
- Eiso Kant, the CTO of Poolside, has joined the conversation, and this is the first time he has met the host in person (41s).
- Eiso Kant is pleased to be on the show and is glad to have finally met the host in person after knowing each other for some time (48s).
What is Poolside? (53s)
- Poolside is involved in the race towards Artificial General Intelligence (AGI) by focusing on building the most capable AI for software development. (1m6s)
- The belief is that the gap between machine intelligence and human-level capabilities will continue to decrease, but achieving AGI is still a distant goal. (1m9s)
- Poolside's approach differs from other companies by concentrating on areas that are economically valuable and can drive abundance, rather than achieving AGI across all human capabilities. (1m58s)
- Current AI models struggle with tasks due to the way they learn, particularly in areas with limited data, which affects their reasoning, planning, and deep understanding capabilities. (2m49s)
- Poolside focuses on software development because there is already a large dataset of code available, with about 3 trillion tokens of usable code for training. (3m24s)
- Despite the vast amount of code, current AI models are limited because coding involves more than just the final product; it includes the intermediate reasoning and steps taken to reach the final code. (3m59s)
- Poolside aims to create the missing dataset that captures the entire process of software development, from task assignment to intermediate reasoning and learning from failures, to improve AI capabilities in coding. (4m38s)
Capturing Iterative Thinking in Uncharted Data (4m42s)
- The process of capturing iterative thinking in previously non-existent or non-captured data is compared to the development of AlphaGo by DeepMind in 2016. AlphaGo was trained initially on existing Go games and then improved through reinforcement learning by playing against itself, which allowed it to learn from wins and losses. (4m43s)
- DeepMind's approach involved using synthetic data in a simulatable domain, which allowed the model to improve without relying solely on human-played games. This method highlights the potential of reinforcement learning and synthetic data in AI development. (6m10s)
- In contrast, real-world problems are complex and cannot be perfectly simulated. An example is Tesla's approach to improving its full self-driving capabilities by collecting real-world data from millions of cars, which helps train more capable AI systems. This data-driven approach is crucial for handling non-simulatable environments. (7m1s)
- The concept of execution feedback is introduced, particularly in the context of code, which is more deterministic and follows a set of rules. This involves using reinforcement learning from code execution feedback to train models in a large environment with real-world code bases, allowing the model to explore solutions and learn from successes and failures. (7m49s)
- The process includes generating not just output code but also the intermediate thinking and reasoning to reach that output. This is important because current models are not very good at producing their thinking, and deterministic feedback, such as code execution feedback, is necessary to improve this aspect. (8m33s)
The Biggest Bottleneck in AI Progress: Compute, Data, or Models? (8m58s)
- The biggest bottleneck in AI progress can be broken down into three main areas: compute, data, and models, with compute and data being crucial for model development and improvement (8m59s).
- The differentiation between two models is primarily the data used, but compute also plays a significant role, especially in generating synthetic data (9m52s).
- Compute is essential for data generation, and having sufficient compute power is necessary to be competitive in the AI space (9m54s).
- The scale of models is crucial, as larger models can generalize more easily due to having more parameters and not being forced to compress as much data into a small space (10m43s).
- The scaling laws, proven by Google and Open AI, demonstrate that providing more data, parameters, and compute power results in more capable models, but there is likely a limit to this (10m53s).
- Compute underpins all AI progress, and having proprietary advantages in applied research and data gathering is equally important, but without sufficient compute power, one cannot compete (11m30s).
- There is still significant room for improvement in model efficiency, driven by advancements in algorithms and hardware, with potentially decades or even hundreds of years of progress still to come (12m17s).
- Increasing advantages in hardware and algorithms will be seen in the coming years, but being excellent at these table stakes is necessary to keep up with others in the AI space (12m32s).
The Value of Synthetic Data (12m49s)
- Synthetic data is often seen as a solution to data shortages, but its value varies across different industries. (12m49s)
- A common misconception about synthetic data is that it involves a model generating data to improve itself, which can seem paradoxical. However, an additional step is needed where an "oracle of truth" evaluates the generated data to determine its correctness or quality. (13m6s)
- In software development, this evaluation can be done by executing code to see if it runs correctly and passes tests, providing objective validation. (13m52s)
- Human feedback is crucial in AI development, especially when perfect simulation is not possible. Humans provide valuable insights by evaluating AI outputs and reasoning, helping to improve AI models. (14m11s)
- Combining deterministic feedback from simulations with human feedback enhances AI training, improving both outputs and reasoning processes. (14m49s)
- Poolside focuses on software development as a key area for advancing machine intelligence, as it allows for scalable simulation and more deterministic evaluation compared to other fields like medicine. (15m9s)
Scaling Laws in AI (15m45s)
- There are different opinions on scaling laws in AI, with some people believing that they have not been fully utilized and have more room to play out, while others have more negative views on the matter (15m48s).
- The initial version of scaling laws focused on the amount of data provided during training, the size of the model, and the compute required, but it is now understood that synthetic data and inference time also play a crucial role (16m11s).
- Scaling laws are not just about applying more compute, but also about using compute at inference time to generate data, such as running models to generate 100 or 50 solutions (16m27s).
- There is still room for scaling up models by increasing data and model size, and most major AI companies are working on scaling up the number of parameters and size of models (16m48s).
- However, training extremely large models is extremely expensive and requires significant capital, which is why fundraising has been important for companies like Poolside (17m13s).
- To make large models cost-efficient for end-users, companies often use techniques like distillation, where a smaller model learns from a larger model, making it economically viable to put in the market and generate revenue (17m52s).
- This approach involves training a very large model, then distilling it down to a smaller model that can match as much of the intelligence as possible, making it possible to run for customers at a lower cost (18m11s).
Projecting Model Costs Over the Next 12-24 Months (18m21s)
- The cost of models in the next 12 to 24 months is expected to be influenced by a competitive price war among large hyperscalers and AI companies like Anthropic and OpenAI, as well as vendors offering open-source models. (18m22s)
- The cost structure of models includes components such as servers, networking, data centers, chips (GPUs), and energy, with the remaining costs being marginal or variable. (18m59s)
- Companies with the lowest cost profiles are those with vertically integrated infrastructure, such as Amazon, Google, and Microsoft, which have invested in their own hardware solutions to reduce reliance on external suppliers like Nvidia or AMD. (19m21s)
- Google has developed its own TPUs, Amazon has worked on Trinium and Inferentia chips, and Microsoft is in the early stages of its chip development journey. These efforts allow these companies to have more control over their costs. (19m52s)
- The competitive landscape is described as a "drunken bar fight," with companies incentivized to reduce model costs by cutting margins and optimizing hardware and intelligence layers. (21m11s)
- Companies aim to distill large, capable models into smaller ones to gain advantages, and the reduction in compute and hardware margins is crucial as prices decrease, similar to trends seen in cloud computing. (21m40s)
Future of Model Distillation (22m10s)
- The discussion highlights the need to distill larger AI models into smaller, more efficient ones to reduce costs, although there is a possibility of having a single efficient model in the future. (22m11s)
- There is a focus on the convergence of human and machine intelligence, which is expected to present numerous challenges and opportunities for applying this intelligence. (22m42s)
- Historically, technological advancements have connected more people and bundled intelligence, leading to exponential growth in solving complex problems like cancer research and business development. (23m5s)
- The current transition involves moving from human intelligence as a bottleneck to leveraging machine intelligence, which, combined with investments in energy, chips, and computing, could lead to significant advancements. (23m54s)
- The efficiency of computing hardware is expected to improve due to capitalist incentives, as there is a large opportunity for directing resources efficiently. (24m26s)
- The gap between AI models and human-level capabilities varies across different fields; for instance, speech recognition models have nearly closed this gap, while other areas like full self-driving still require progress. (25m1s)
- The gap between human and machine intelligence is closing in some areas, such as Tesla's Full Self-Driving (FSD) technology, but remains large in software development. Models are useful as assistants and drive economic value, but there is still a significant gap between models and developers. The goal is to have models as capable as developers, or even more so in the future. (25m30s)
- Closing the intelligence gap depends on the availability of large-scale data. The larger the gap, the more data is needed to close it. The intersection of data availability and economic value is where companies can thrive. GitHub is highlighted as a significant source of public code data, although private code data is not accessible for training. (26m12s)
- The capabilities race in the industry is driven by four key factors: compute, data, proprietary applied research, and talent. Talent is crucial, along with product and distribution in the market race. Microsoft is noted for its strong market positioning. (27m21s)
- The company has raised $600 million, including a recent $500 million round, to enter the race for advanced model capabilities. This funding has enabled the deployment of 10,000 GPUs, facilitating advancements in model capabilities through reinforcement learning and large-scale data generation. However, this funding is sufficient only for the current moment, and more will be needed in the future. (28m5s)
- Currently, the world is still catching up with the ability to interconnect large numbers of GPUs, with interconnecting more than 32,000 GPUs being extremely challenging, although it is becoming possible to interconnect 100,000 GPUs (29m7s).
- A major obstacle in training models is creating a million or 10 million GPU cluster, which faces both algorithmic challenges and physical limitations (29m17s).
- Despite these challenges, it is not possible to buy unlimited advantages with unlimited money due to the existing limitations, allowing companies like Poolside to exist and compete with 10,000 GPUs (29m34s).
Does Cash Directly Correlate to Compute Access? (29m36s)
- The relationship between cash and access to compute resources is complex and depends on the amount of cash and compute needed. (29m37s)
- About a year and a half ago, there was a significant imbalance between the supply and demand for compute resources, even for early-stage AI companies. (29m52s)
- Companies like Nvidia are incentivized to support early-stage AI companies, making it easier for them to access compute resources compared to larger enterprises. (30m3s)
- Despite this support, there was still a mismatch between demand and supply, requiring companies to build relationships and have multiple plans to secure compute resources. (30m19s)
- In the last six months, there remains a significant supply shortage of compute resources, particularly GPUs, and early-stage startups must make strategic decisions about partnerships and infrastructure that will affect them in the future. (30m32s)
- The demand for GPU and similar compute resources continues to exceed supply, and insights into this imbalance can be gathered from the earnings calls of major companies like Nvidia, Amazon, Google, and Microsoft. (31m17s)
Eiso’s Perspective on Larry Ellison’s $100B Foundation Model Entry Point (31m35s)
- Larry Ellison recently stated that entering the race for advanced AI would require an investment of $100 billion, which is considered the starting point for becoming a hyperscaler capable of deploying data centers globally with GPUs to serve AI models. (31m40s)
- The race towards more capable AI involves significant capital expenditures by cloud companies, with investments exceeding $100 billion over several years, as they aim to close the gap between human and machine intelligence. (32m24s)
- The process of developing AI models involves both capital expenditures (capex) for model creation and operational expenditures (opex) for running them, requiring a large-scale physical infrastructure worldwide. (33m49s)
- The economic viability of AI models depends on their ability to provide value to end users, and the large-scale deployment of AI requires extensive data centers close to end users to minimize latency. (34m0s)
- The current buildout of physical infrastructure for AI is one of the largest seen in recent decades, with the evolution of AI models outpacing the development of data centers. (34m34s)
- The current landscape of data centers is limited in terms of the number that can support the energy and power requirements needed for increasingly large clusters. (35m5s)
- Data centers from two years ago differ significantly in size and power requirements compared to those anticipated in the next two years, primarily due to the need for interconnected servers for training large models. (35m23s)
- Inference and training have different infrastructure needs; training requires all machines to be connected in the same location, which changes the design of data centers. (35m35s)
- As models scale up and are trained on more data, the need for communication between servers increases, necessitating proximity to avoid slow and economically unviable training processes. (35m58s)
- During training, numerous copies of a model are distributed across many machines, which must communicate with each other to improve learning, unlike the fewer servers needed for running a model. (36m40s)
Eiso’s Outlook on Nvidia's Dominance and the Future of Compute (36m50s)
- Nvidia has played a pivotal role in the development of AI hardware, having recognized early on the transformative potential of AI and consistently investing in advanced hardware solutions since at least 2016. (37m21s)
- Google and Amazon have followed Nvidia's lead, becoming significant players in the production of AI chips, with Google advancing to the fifth generation of its TPUs. These companies are key due to the large volumes of chips they produce and their continuous innovation in chip technology for AI training and inference. (37m40s)
- AMD is a competitor to Nvidia but lacks its own cloud infrastructure, making it reliant on market demand for its chips. This contrasts with Google and Amazon, whose demand is driven by their own AI needs rather than just chip sales. (38m7s)
- The future landscape of AI hardware is expected to be dominated by Nvidia, Google, and Amazon, with potential contributions from AMD and possibly Microsoft, which may develop its own silicon. These companies are anticipated to be the main forces driving the industry forward. (38m34s)
Has Innovation Stalled Awaiting Nvidia's Blackwell? (38m51s)
- The delay in the release of Nvidia's Next Generation chips, specifically Blackwell, has been beneficial for training on H2O chips, as it allows for a competitive advantage in the field (38m57s).
- The performance increase in training with each new generation of Nvidia chips is around 2X every 2 years, but the impact on inference is not as significant (39m24s).
- Blackwell is expected to unlock a much larger game for inference when it is released, but it may not necessarily require an upgrade from H2O chips for training purposes (39m39s).
- The operations performed on these chips are still the same, consisting of matrix multiplications and additions, so the Blackwell generation does not unlock anything new from a training perspective (40m1s).
- GPT 5 is expected to deliver a step function change, but it is uncertain what it will deliver and whether it will be enough to make a significant impact (40m32s).
- In the future, people will look back on this moment and realize that they did not fully internalize the potential value and abundance that will be unlocked by advancements in AGI, energy, and space (40m48s).
- There are three mountains that humanity will climb in this century: AGI, energy, and space, and each mountain will be exponentially larger than the previous one (41m12s).
- The amount of funding required to compete in the AGI space is uncertain, with $600 million potentially not being enough, and other companies like Open AI and Google planning to spend much more (41m29s).
- The ingredients of the capabilities race, including compute, talent, data, and proprietary applied research, are not all directly correlated with dollars spent, and success is not guaranteed by simply investing more money (41m56s).
- In the race towards Artificial General Intelligence (AGI), financial resources are crucial for computing power, but there are also time and physical constraints on how large compute clusters can be built for training. These constraints provide opportunities for companies to gain advantages in data, talent, and proprietary research. (42m49s)
- The movement of knowledge between companies is common, raising questions about the existence of proprietary knowledge in the market. (43m21s)
- Poolside's recent $500 million capital raise did not include major hyperscalers like Google, Microsoft, or Amazon, which was a deliberate decision. The company aims to pursue its vision as a standalone entity without forming equity relationships with these large corporates at this time. (43m42s)
- Nvidia was the only corporate participant in Poolside's funding round, chosen due to close collaboration on next-generation chips and software. (44m27s)
- Large technology companies investing in frontier AI companies is considered a strategically optimal move. (44m55s)
- There is a trend of smaller AI companies being acquired by larger incumbents, but few such companies remain. Notable companies like Cohere and XI are mentioned as capable players in the space, with XI being unlikely to be acquired. (45m0s)
OpenAI, Anthropic, or X.ai — Which to Buy and Why? (46m6s)
- The discussion involves evaluating which company to invest in among OpenAI, Anthropic, and x.ai, with OpenAI valued at $156, Anthropic at $40, and x.ai at $24. (46m7s)
- x.ai is noted for its rapid development of a 100,000 GPU cluster in Tennessee, showcasing its strength in building physical infrastructure quickly, attributed to Elon Musk's influence. (46m40s)
- OpenAI is recognized for its success with ChatGPT and its strong revenue generation through APIs, positioning it ahead in the market. (47m10s)
- Anthropic is praised for its thoughtful researchers and rigorous scientific approach, contributing to its strengths. (47m25s)
- The decision on which company to invest in would ideally involve spending time with the leadership teams of each to better understand their potential. (47m42s)
- OpenAI's recent $6.6 billion raise is likely focused on enhancing compute and data capabilities, crucial in the competitive market of general-purpose models. (48m10s)
- The challenges of leading a company like OpenAI are compared to Elon Musk's experiences, highlighting the pressures and complexities of building a platform and consumer product simultaneously. (48m17s)
- Elon Musk is admired for his ability to take significant risks and succeed with companies like Tesla and SpaceX, demonstrating a unique understanding of risk that others may not possess. (50m52s)
Comparing Crypto & AI: Decentralization vs. Centralization (51m0s)
- Crypto embodies decentralization, while AI embodies centralization, according to a quote by Teal, highlighting the contrasting ideals of the two technologies (51m1s).
- The concept of decentralization in crypto is incredible, but its promise has been distorted by the presence of bad actors who have driven out good actors due to the incentives of making money quickly (51m35s).
- In contrast, AI has a set of people worldwide who fundamentally disagree on how to achieve it but see the potential for a significant shift in the world by closing the gap between machine and human intelligence (52m31s).
- The development of AI may drive centralization due to the scarce resources required, such as talent, proprietary points of view, and research, leading to a small number of companies dominating the field (52m50s).
- However, it is possible for new companies like Poolside, OpenEye, and Anthropic to gain massive escape velocity and sit alongside established companies like Google, Amazon, and Microsoft (53m33s).
- The presence of "bad actors" or "tourists" in the AI industry, who are not in it for the long term or are only in it for a story, can be seen in public company CEOs who feel pressured to tell an AI story and show innovation (54m0s).
- The revenue generated by AI today is a mix of experimental and true deployment phases, depending on the use case, with some areas like AI-assisted software development already past the experimental phase (54m36s).
- AI-assisted software development is expected to be the norm for the foreseeable future, with developers increasingly relying on AI assistance (54m52s).
- Use cases like speech recognition and image generation are commoditizing quickly, while others still have a gap in understanding or long-term potential (55m4s).
The Decision to Stay Europe-Based (55m23s)
- The company initially considered establishing itself in the Bay Area but decided to remain Europe-based due to the significant talent pool available across Europe and Israel. (55m42s)
- A comprehensive list of 3,300 potential candidates was created, highlighting expertise in areas such as distributed training, GPU optimizations, data work, and reinforcement learning. The Bay Area had the highest concentration of talent, but there was also a substantial presence in Europe and Israel. (56m13s)
- The talent was distributed across various European locations, including the UK, Switzerland, Tel Aviv, Amsterdam, and Paris, with the UK having the largest concentration. (56m54s)
- Many talented individuals preferred to stay in Europe rather than relocate to the Bay Area, presenting an opportunity for the company to build a strong talent base in Europe. (57m21s)
- The company has established a presence in London with about 15 people and in Paris with two people, recognizing Paris as a significant AI hub in Europe. (57m58s)
- DeepMind and Meta have historically contributed to building a strong AI talent base in London and Paris, with DeepMind being particularly influential. (58m16s)
- Yandex has also developed a remarkable talent pool in Russia, with many of its researchers and engineers now dispersed across Europe. (58m43s)
Work Ethic & Work-Life Balance (59m1s)
- The discussion addresses the importance of work ethic and standards in Europe, particularly in the context of the booming AI industry. It highlights a tweet by Aaron Levi from Box, suggesting that working hard during the early years of AI development is crucial as it sets the stage for who will compete in the race for Artificial General Intelligence (AGI) (59m1s).
- The perspective shared emphasizes the significance of looking back at pivotal moments in technology, such as the advent of mobile internet and personal computers, and recognizing the importance of giving one's all during these transformative periods. It is suggested that AGI is a race, unlike most startups, and requires a team passionate about being part of this race (59m46s).
- The approach to building a team involves being upfront about the sacrifices required to compete in the AGI race, akin to striving for a gold medal in sports. This openness is communicated from the first interaction with potential team members, and there is no shortage of people in Europe and America willing to join this race (1h0m40s).
- A reference is made to Chase Coleman's observation about the value creation in internet companies post-Netscape, where 1% of the value was created in the first two years, and 99% in the years following. This raises a question about whether the current situation with AGI is different, given the exponential technological progress and the increased understanding and capital available today compared to 1996 (1h1m20s).
- The argument is made that the requirements for building technology today are vastly different from those in 1996, suggesting that the next few years could be crucial for development, potentially differing from past technological cycles (1h2m35s).
- The economic value generated by advancements in AI is expected to surpass current levels exponentially over the next 5 to 10 years. However, there is skepticism about whether the companies being built today will become the future giants that enable these advancements. (1h2m57s)
- A significant concern is that substantial financial investments are required to achieve technological breakthroughs, which are then often leveraged by other entities to create highly valuable companies. This pattern is exemplified by the battery industry, where many companies have made breakthroughs but were acquired for their intellectual property without becoming major players themselves. (1h3m22s)
- BYD, which started as a battery company and is now the largest seller of electric cars globally, illustrates the importance of deep vertical integration. (1h3m54s)
- Poolside is focused on building foundational models with a mission towards achieving Artificial General Intelligence (AGI). The current emphasis is on enhancing AI capabilities in software development and creating a comprehensive end-to-end business model. (1h4m11s)
- The belief is that value will not only accumulate at the model layer but will extend to the end user. Poolside aims to capture this value by developing solutions that span the entire process, although it is acknowledged that more value may be built on top of their work in the future. (1h4m27s)
Is China 2 Years Behind Than Europe? (1h4m53s)
- China is not two years behind Europe in terms of AI or AGI progress, but rather, they have an incredible level of capabilities that should not be underestimated (1h4m59s).
- A significant amount of AI research is being published openly by China, which is an interesting strategy that may not be immediately apparent unless one is in the industry (1h5m8s).
- This open publication of research is likely a game theory optimal move, as it allows China to attract talent from around the world, despite not being at the forefront of the global AI scene (1h5m28s).
- China's decision to open up its research is a strategic move to attract talent, which is a crucial aspect of advancing AI and AGI capabilities (1h5m34s).
- It is essential to acknowledge China's capabilities and progress in AI and AGI, rather than underestimating them or thinking of them as years behind (1h5m42s).
- The West can benefit from making it attractive for Chinese talent to come to their countries, as this can help accelerate progress in the AI and AGI capability race (1h6m23s).
- One of the most practical strategies for the West is to make it easier for Chinese talent to come to their countries, which is a crucial aspect of advancing AI and AGI capabilities (1h6m41s).
Quick-Fire Round (1h6m48s)
- The importance of scale and data in AI development has become increasingly clear over the last 12 months, with a focus on both compute and data being crucial (1h7m4s).
- Not selling Source to GitHub was likely the dumbest financial decision, considering it was an all-stock offer and GitHub sold to Microsoft less than a year later, but it ultimately led to the creation of Poolside (1h7m14s).
- The mission of Source and Poolside is the same, with a focus on AI writing code, and not selling Source allowed for the continuation of this mission and the opportunity to build Poolside (1h7m40s).
- There are no regrets about not selling Source, as it led to meeting the co-founder Jason and starting a conversation about AI progress and its applicability to software development (1h8m0s).
- The biggest misconception about AI in the next 10 years is that progress will halt, but this is unlikely, and a global conflict disrupting the supply chain of chips could potentially cause progress to slow (1h8m18s).
- Mark Zuckerberg would be a desirable board member due to his conviction in building an incredible company and his ability to envision a future that will massively change the world, as seen in his work on AR and VR (1h8m38s).
- The worst thing that could happen for AI with regards to regulation is that it becomes an expensive bureaucratic overhead, harming young startups and not affecting companies with massive amounts of capital (1h9m33s).
- Regulations should focus on the end-user application of AI, holding companies accountable for the end use of their technology, rather than limiting compute power or training data (1h9m55s).
- Yuri Milner is appreciated for his contributions to scientific progress and his vision of humanity's future, which includes becoming a space-faring civilization. He emphasizes the importance of spreading humanity across the universe and has written a book or manifesto available online for free. (1h10m36s)
- Yuri Milner is recognized as a successful global investor who has strategically invested in technology across various regions, including the US, India, Indonesia, and Asia. He is noted for his strong conviction in the potential of technology over the next decade. (1h12m23s)
- A personal anecdote is shared about living on a sailboat, which began as a project with a partner and a golden retriever. The experience of living on the boat, despite its initial disrepair, is likened to a pursuit of freedom and adventure, similar to the drive to advance the world. (1h12m55s)
- The focus is on the importance of the journey and experiences with people rather than material possessions. The speaker emphasizes that the journey and the people involved are more significant than the material outcomes. (1h14m10s)
- There is a reflection on past experiences of acquiring material possessions and the realization that they do not hold lasting value. The emphasis is on the journey and the people involved in achieving goals. (1h14m43s)
- The speaker highlights the importance of working with amazing people and focusing on significant challenges, which leads to incredible experiences. (1h15m33s)
- In the context of Poolside, a premortem analysis suggests that the main risk is losing momentum in the competitive race for capabilities and market presence. Excellence in both areas is crucial to remain relevant. (1h16m3s)
- The speaker notes that a commonly overlooked question is about personal motivation or the "why" behind actions. Understanding the motivation is seen as crucial for success in any ambitious endeavor, as it is the people, not resources, that drive outcomes. (1h16m50s)
- Eiso Kant discusses the importance of asking oneself why they do what they do, referencing Toyota's "five whys" method. (1h17m41s)
- He explains that he is not calm or at peace unless he is working on the hardest possible problems that align with his values. (1h17m51s)
- Eiso mentions that his mind is always active, often waking up early in the morning, and he feels at ease only when working on significant and ambitious projects. (1h18m1s)
- He reflects on his past experiences, noting that when he worked on less challenging projects, he did not feel at ease, despite always working hard. (1h18m13s)
- Eiso states that he has never worked as hard as he does at Poolside, and although it is intense and stressful, it brings him peace. (1h18m31s)