How Startups Can Crush Enterprise AI Sales | E2050
23 Nov 2024 (3 months ago)
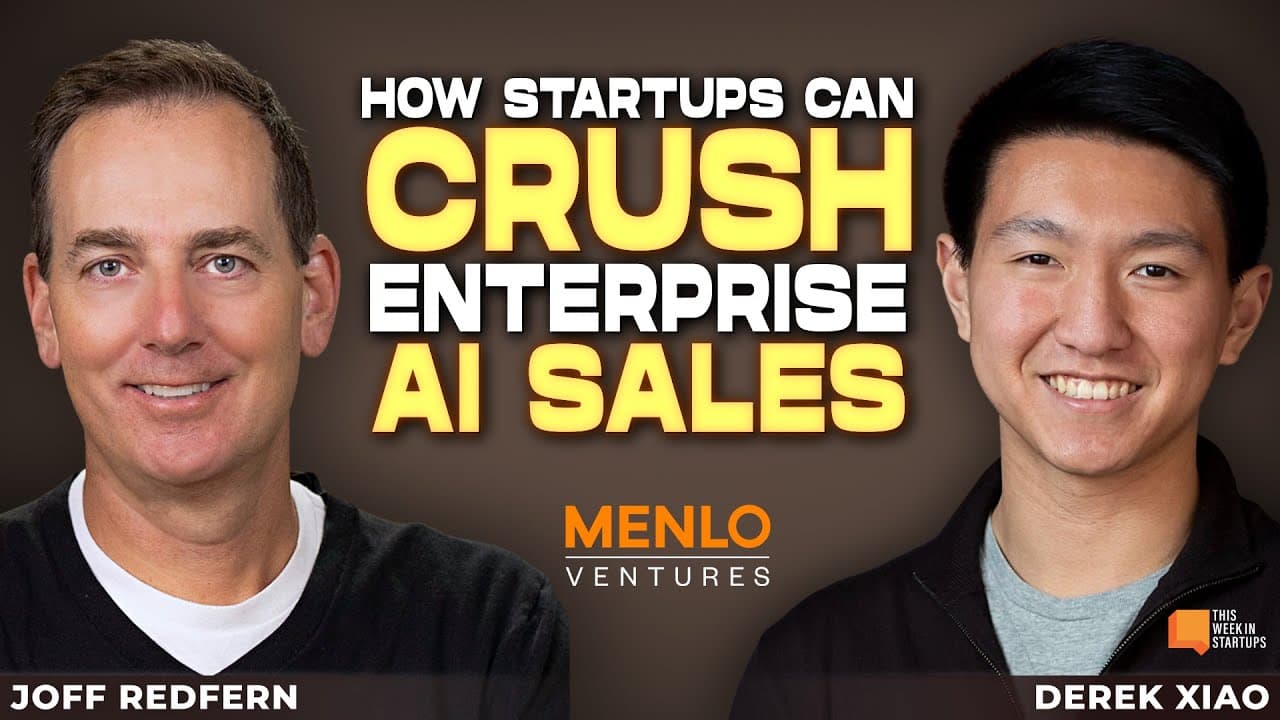
Joff Redfern and Derek Xiao join Alex (0s)
- A startup should focus on what's different, specifically how reasoning can be used as a utility, and consider how tasks in the economy can be divided between AI and humans (3s).
- This division of tasks can lead to changes in workflow, which has traditionally been built with humans at the center, and could potentially be shared between algorithms and humans (25s).
- The current hype around AI includes topics such as fundraising, applications, agents, chatbots, and job loss, but it's essential to look beyond the headlines and understand how Enterprise generative AI spend is actually doing (1m28s).
- A venture capital firm, Minow, released a detailed report on Enterprise generative AI spend, which led to the authors being invited to discuss the topic (1m45s).
- One of the authors, Joff Redfern, has a background as a product leader, having worked at Yahoo from 2003 to 2009, and later held positions at Lassan and Ling Den (2m31s).
- Joff Redfern's experience at Yahoo is notable, as the company was a significant player in the early days of the internet, often compared to Google (3m11s).
- Yahoo's evolution over the years has been significant, and it's no longer the same company it was in its early days (3m37s).
- The discussion with Joff Redfern and another author, Derek Xiao, aims to delve into the details of the report and provide insights into Enterprise generative AI spend (2m19s).
Yahoo's evolution and Anthropic's recent funding (4m8s)
- Derek Skalet, the president of the Harvard Crimson, has a background in journalism, which has overlap with Venture Capital, and is currently tagged in on the Anthropic side of things on his Menlo page (4m38s).
- Anthropic recently announced that it raised $4 billion more from Amazon, bringing the total to $8 billion, and this investment is a furtherance of the close partnership between Anthropic and Amazon (4m49s).
- Anthropic is one of the foundation models, and the thesis behind the investment was that it would be one of the companies that would matter the most in the AI Revolution (5m25s).
- The investment in Anthropic was made in 2023, and since then, the company has deepened its relationship with Amazon, which is why the recent announcement is not surprising (5m30s).
- Joff took part in the series C and led the series D of Anthropic's funding, throwing an SPV and leading $500 million of the $1 billion raise on the D side (5m52s).
- The investment in Anthropic seems to be a smart move given how AI has progressed since the deal was put together (6m5s).
- The discussion is expected to share some findings on the LLM side as it pertains to the Enterprise (6m17s).
AI spending trends in enterprises (6m23s)
- There are two large markets for Large Language Models (LLMs): the consumer-based market and the enterprise-based market, with different approaches to competition and market strategies (6m24s).
- Having a company like Anthropic in a broader portfolio can bring in information that can be used to make new investment decisions, but this information is segregated to maintain a "church and state" separation (7m15s).
- The relationship between Anthropic and the investment team provides benefits such as early access to new models, $25,000 in Anthropic credits, and access to development teams and expertise (8m3s).
- The Anthology fund, a $1 billion fund, looks for pioneering AI founders and provides them with benefits such as early access to models, credits, and access to development teams and expertise (7m48s).
- The investment team runs a "Builders Day" event, which brings together experts from Anthropic and companies building on the platform, providing a successful and helpful experience for both parties (8m22s).
- The team at Anthropic is highly talented, and with $4 billion in funding, they can continue to hire top talent, which is essential in addressing the AI talent drought (9m6s).
- AI spending in the enterprise market surged to $13.8 billion in 2024, up from 2023, with this growth being faster than initially anticipated (9m20s).
- The growth of AI spending in the enterprise market is expected to continue, with the investment team's report predicting a continuance of the AI talent drought (9m13s).
- The investment team's report, "2024 The State of Generative AI and the Enterprise," provides insights into the growth of AI spending in the enterprise market and the trends shaping the industry (9m22s).
LinkedIn Ads - Get a $100 LinkedIn ad credit (10m14s)
- To effectively bring a product to market, it's essential to target the right customers, and LinkedIn ads provide an easy solution for this by allowing precise targeting of professionals most likely to care about the product based on job title, industry, company, location, and more (10m22s).
- LinkedIn ads can be used to build a B2B audience, such as targeting midsize, large, or emerging small and medium-sized businesses (SMBs), and drive results by building key relationships (10m38s).
- With LinkedIn ads, users gain access to over a billion members, including 130 million decision-makers who can make purchasing decisions, and 10 million elite C-level executives, such as Chief Technology Officers, Chief Strategy Officers, and Chief Executive Officers (10m54s).
- LinkedIn ads offer a high return on ad spend, with 79% of B2B marketers saying LinkedIn delivers the best paid media results, and users can expect two to five times the return on their ad spend (11m23s).
- To start converting B2B audiences into high-quality leads, users can claim a $100 credit for their next campaign by visiting linkedin.com/thisweekinstartups, with terms and conditions applying (11m36s).
- Surprisingly, traditional laggards in technological adoption, such as healthcare and legal, are leading the AI revolution, with hundred million dollar plus companies emerging in these verticals (11m55s).
AI's impact on healthcare and legal sectors and its rapid adoption (12m20s)
- The healthcare and legal industries are considered ripe for AI application due to the voluminous amount of written information they handle, and their historical inefficiencies may have accumulated a significant amount of "technical debt" in their operations, making them ideal for AI deployment (12m38s).
- The total market size and technology spend in these industries are massive, and AI can now automate a lot of tasks that tech couldn't touch before, making them a prime target for AI solutions (13m14s).
- The adoption curve for AI is surprisingly steep, with the enterprise level seeing a significant increase in AI mentions in earnings calls, from zero in 2022 to 18 mentions in the second earnings call of 2023 (14m20s).
- The year 2023 is considered the year of the pilot for AI, with CEOs and CFOs asking their R&D groups to explore the use of generative AI, leading to teams being pulled together to experiment with the technology (15m6s).
- The focus has shifted from experimentation in 2023 to moving AI solutions into production in 2024, with a predicted 6X increase in overall spend at the enterprise level, reaching close to $14 billion (15m40s).
- The data sourcing for AI solutions is a growing industry, and the numbers may be subject to categorization and bleed between different pockets, but the trend is clear: AI is becoming increasingly important for enterprises (16m6s).
- Enterprise AI spend can be broken down into two main buckets: the infrastructure layer, which includes large language models (LLMs) and the infrastructure needed to bring AI into the organization, and the application layer, which involves the actual use of AI in various departments and functions (16m9s).
- The infrastructure layer accounts for 2/3 of the total spend, with $9.2 billion, and the largest spend within this layer is on foundation models, totaling $6.5 billion (16m52s).
- The application layer accounts for the remaining 1/3 of the spend, with $4.6 billion, and has seen a significant year-over-year increase (17m15s).
- The application layer can be further broken down into vertical, departmental, and horizontal AI, with a broad set of general use cases in the Enterprise (17m39s).
- A survey of 600 IT decision-makers was conducted to gather data on generative AI spend, with questions on budget allocation, overall spend, and specific tools being used (17m59s).
- The survey aimed to address the lack of good data on what IT decision-makers are actually spending on generative AI and what use cases they have (18m22s).
- The results of the survey provide insights into what IT decision-makers are adopting and where their money is going towards (18m45s).
- The year-over-year growth in the application layer spend is a key takeaway, rather than the exact figure of $4.6 billion (19m0s).
- The application layer has a broad set of general use cases in the Enterprise, making it a fascinating area of study (19m18s).
Popular AI use cases in enterprises (19m25s)
- Enterprises typically concentrate on one department or use case for AI technology, but its usefulness is evident in various applications, with an average of 10 general use cases per Enterprise (19m26s).
- The most popular AI use case is code generation, with 51% of surveyed companies using AI for code generation and building software, and many paying for code completion tools (19m48s).
- Code completion tools are widely used, with products like GitHub Co-Pilot being one of the fastest-growing revenue products, generating over $300 million in annual revenue (20m21s).
- Another popular AI use case is support chatbots, with 31% of surveyed companies deploying chatbots, and products like Sierra, Decagon, and Asana being used in IT service management (20m37s).
- IT service management is a significant use case for AI, with companies like Asana providing solutions in this area (20m53s).
Zendesk - Get six months free (20m56s)
- Startups need to prioritize customer satisfaction by providing unreasonable hospitality, which requires the right tools and platforms to deliver exceptional customer experiences and build stronger relationships without increasing headcount (20m56s).
- The Zenes Suite is a platform that offers all the necessary tools for startups to achieve this goal, and it is used by companies like Squarespace, Uber, and Instacart (21m8s).
- Unity, a well-known company, saved $1.3 million with Zenes' automations and self-service, and saw an 83% increase in their first response time (21m34s).
- The Zenes for Startups program provides unlimited access to all Zenes products, expert insights, best practices, and entry into their community of founders at no cost for the first six months (22m1s).
- Zenes offers a range of products, including Enterprise search and retrieval, with companies like Glean achieving dramatically better results in recent years (22m25s).
- Other notable products in the space include SAA, meaning summarization, and AI-powered tools like Firy, Otter, and others that provide features like conversation summarization (22m46s).
- Copyrighting is another key area, particularly relevant to the use of Large Language Models (LLMs) (23m10s).
- Startups can take advantage of Zenes' offer of six months free to scale their customer support and get ready to grow with the best in the industry (22m16s).
The role of LLMs in content creation and workflow automation (23m17s)
- Large Language Models (LLMs) are like calculators for words, capable of authoring content and helping users be more precise and concise, with products like Writer, TypeFace, and Copy AI being leaders in this space (23m18s).
- LLMs can perform tasks such as code generation, chatbots, meeting summarization, and copyrighting, all of which involve creating characters on a screen (24m0s).
- Workflow automation was expected to be a major application of LLMs, but it has been slower to develop than expected, despite the enthusiasm for Robotic Process Automation (RPA) in the past (24m10s).
- The first wave of generative AI applications were retrieval-augmented generation (RAG) apps, which use external knowledge stores for tasks like synthesis, with examples including Eve, a legal co-pilot that generates reports and legal briefings (24m59s).
- The next generation of LLMs is moving towards agentic architectures, which are more advanced and can automate workflows across horizontal and vertical areas, with examples including UiPath and Tenor (25m34s).
- The technology for agentic approaches is now available, and it is expected to explode in 2025, with the market moving from retrieval-based architectures to more agents that can automate workflows (26m13s).
- The improvement in technology is also due to "time series thinking" when models take a little more time before making a decision or returning a prompt, making the agentic approach more feasible today (26m51s).
Advances in generative AI applications and agentic systems (27m4s)
- Advances in generative AI applications and agentic systems are crucial for improving AI performance, particularly in test time inference, where models can make fewer errors as they become smarter (27m4s).
- Traditional agents have limitations due to recursive loops, where errors can compound, resulting in unacceptable error rates for enterprises, even with high accuracy rates (27m21s).
- Applying AI to specific domains with data scaffolding and "agent on Rails" approaches can help mitigate these issues by setting guard rails with hardcoded rules to increase accuracy (28m6s).
- The need for guard rails is a trade-off between degrees of freedom and accuracy, with the ultimate goal of achieving Artificial General Intelligence (AGI) (28m57s).
- The level of specificity required for guard rails can vary, with more specific use cases resulting in higher accuracy and robustness, but also limiting degrees of freedom (28m49s).
- The development of guard rails can be complicated, and people are still figuring out the optimal approach, with the answer likely lying somewhere between AGI and current hardcoded application logic (28m44s).
- Progress in code generation can be measured using benchmarks like the Squee Bench, which tests real-world tasks faced by software developers, with significant improvements seen in recent months (29m43s).
- For example, the best software agentic system in January completed only 4% of the Squee Bench tests, while a company called Cognition achieved 14% in March, and a portfolio company called All Hands now holds the number one score, solving 53% of the tests (30m11s).
Beehiiv - Get 30 days free and 20% off your first 3 months (30m43s)
- Newsletters are a great way for startups to stay relevant and serve as an incredible growth engine, as they provide a direct line of communication to customers without relying on middlemen or algorithms (30m49s).
- A successful newsletter requires a world-class platform, which is why the "This Week Startups" newsletter was moved to Beehiiv (31m19s).
- Beehiiv is a newsletter platform designed to help grow communities and turn newsletters into powerhouses, featuring an AI-driven post builder, a built-in referral program, a top-notch ad network, and easy-to-use tools for monetization and subscription management (31m30s).
- Beehiiv plans start at $39 a month, but an exclusive deal is available, offering a 30-day free trial followed by 20% off the first three months (31m52s).
- To take advantage of the exclusive deal, users can go to beehiiv.org (32m5s).
Enterprise generative AI spend, ROI, and budget expansion (32m14s)
- The rate of progress in AI is expected to continue, but it will be invariant and dependent on the department and use case, with potential for significant advancements in accuracy and optimization (32m31s).
- The current state of AI is fairly unoptimized, and even with existing models, there's still room for improvement, let alone new advancements in the underlying foundation model (32m58s).
- The revenue from Enterprise generative AI is spread out among startups, with a large number of companies competing for a share of the 4.6 billion dollars from the app side, raising concerns about whether there's enough spend to support the number of startups (33m44s).
- Enterprise AI budgets are often allocated from innovation budgets, which are not permanent, but as ROI improves, these budgets may be pulled from permanent budgets (34m13s).
- Historically, budgets for tooling and human capital are being spent by business units, and with the rise of services as software, human capital budgets are also being moved into software spend (34m40s).
- Reducing headcount and replacing it with software spend can provide significant cost savings, as human capital is costly, and budgets for software that replaces human activity can be relatively rich (35m5s).
- The use of AI is not just about reducing headcount, but also about expansion, as companies may not have enough people to fill certain roles, and AI can help expand and grow departments (35m28s).
- There are opportunities for AI spend across various departments, including those that are historically late adopters of technology, such as the legal department (35m58s).
- Enterprise AI budgets are promising and expected to grow as the return on investment (ROI) becomes clearer, with a third of survey respondents still figuring out their exact implementation on AI strategy (36m11s).
- The total addressable market (TAM) for AI generative AI in the Enterprise is huge, and it also makes the overall TAM for software larger, as it unlocks spend from departments that didn't have technology budgets initially (37m10s).
- Generative AI adoption is more expansive than replacement, with over half of the permanent budget for generative AI coming from new budget, and it's creating a new line item rather than replacing existing software spend (37m43s).
- The adoption of generative AI is happening across various departments, including those that used to not spend a lot on technology, such as sales, marketing, data science, and Human Resources (38m5s).
- Startups are popping up in different verticals, including Healthcare, Legal, Financial Services, and various departments, which is exciting for the generative AI category (38m11s).
- When selecting generative AI tools, the highest priority is ROI, rather than cost, which is a surprising but important consideration for startups (38m41s).
Selecting generative AI tools and AI-first startup opportunities (38m45s)
- When approaching AI software, people want to achieve the biggest impact possible, rather than spending as little money as possible (38m46s).
- Startups should focus on what they can do now that they couldn't do yesterday, and look at what's different and unique about their product or service (39m21s).
- A key consideration for startups is how to change the way workflows are built, which have traditionally been centered around humans, and instead create a shared workflow between algorithms and humans (40m48s).
- Many Enterprise-level workflows are complicated and tool-rich, with an average sales team using 12 different tools, and software development teams using over 12 different tools (41m13s).
- Startups have the opportunity to reimagine these workflows and create something new, rather than just trying to "AI-ify" the existing workflow (41m30s).
- A recommended approach is to take a "first principles" approach, thinking about what is possible given the current state of technology, rather than just layering AI capabilities onto existing products (41m54s).
- This approach is referred to as an "AI-first" approach to building software, which involves starting from the ground up and building a product that is centered around AI capabilities (42m15s).
- Professor Daniel Rock's idea that every role in the economy can be seen as a bundle of tasks, some of which can be done by AI and some by humans, is relevant to this approach (40m24s).
- Startups should consider how they can use AI to change the way tasks are allocated between humans and algorithms, and create new workflows that take advantage of this (40m38s).
- Many existing software systems need to be completely rethought and rebuilt from scratch due to the emergence of AI, as simply adding AI features to existing systems is not sufficient (42m20s).
- The idea that AI can be easily added to existing systems of record, such as Salesforce, has been proven incorrect, and AI is now considered its own independent category (43m0s).
- This shift gives an advantage to startups, as they can develop AI-native approaches and domain-specific workflows that better meet the needs of enterprises (43m36s).
- Enterprises are trying out AI features, such as Salesforce's Agent Force, but are often disappointed with the results, creating opportunities for startups to fill the gap (43m50s).
- The transition to an AI future will be challenging for companies with legacy software offerings, leaving trillions of dollars in market cap up for grabs for startups that can capitalize on the shift (44m15s).
- The success of companies in adapting to the AI revolution will vary by domain, making it difficult to predict which companies will thrive and which will struggle (44m37s).
- Some companies, such as Adobe, have successfully navigated significant technological shifts in the past, but it's unclear whether they will be able to do so again in the face of the AI revolution (44m41s).
- Startups and investors need to carefully evaluate the competitive landscape and identify areas where incumbents are well-positioned to adapt to the AI revolution, and where opportunities exist for disruption (45m13s).
- The traffic on websites like Stack Overflow has decreased as people are now using Large Language Models (LLMs) to get coding guidance, with some users even utilizing tools like Chat to cheat on homework (45m39s).
- The rise of AI has led to the disruption of legacy companies, with cloud and Software as a Service (SaaS) models starting to feel outmoded, similar to how Salesforce was once considered the future of technology (46m22s).
- The development of AI is built on the shoulders of giants, with various building blocks such as the internet, cloud computing, and vast amounts of GPUs and CPUs being necessary to reach the current state of AI (46m42s).
- The internet and cloud computing played crucial roles in digitizing the world's information and unleashing vast amounts of computing power for training and inference (46m47s).
- Legacy companies like Yahoo, which invented the internet portal, are no longer given much credit for their contributions, and it is likely that current AI companies will also be surpassed by new startups and innovations (47m6s).
- Startups and new companies will likely capture new value by building on the shoulders of giants, but it is essential to acknowledge the contributions of previous innovators (47m35s).
- The development of AI is a lineage of building blocks, with each innovation leading to the next, and it is essential to recognize the importance of these building blocks in reaching the current state of AI (47m2s).
Competition and strategies for AI startups (47m43s)
- Incumbent AI companies, such as Open AI and Anthropic, are major players in the AI space, with Open AI recently releasing a search product that is considered to be of high quality. (47m57s)
- When advising startup founders, it is essential to consider the near-term roadmap of these large companies, as their ultimate goal is to achieve Artificial General Intelligence (AGI), and their focus will be on tasks that bring them closer to this goal. (48m29s)
- Anthropic has stated that they will not pursue side quests such as image generation, as it is not necessary for achieving AGI, but will focus on tasks like reasoning and using tools like web browsers. (48m45s)
- For AI startups to succeed, they need to get two things right: making the base technology work and applying it to specific workflows or industries, such as healthcare or revenue cycle management. (49m5s)
- While large companies like Anthropic will continue to advance the intelligence of their base language models, they may not focus on applying these models to specific industries or workflows, leaving opportunities for app-focused startups. (49m41s)
- Startups can navigate the competitive landscape by identifying areas that are not a priority for large companies, such as applying AI to specific industries or workflows, and focusing on those areas. (50m7s)
Training AI for market verticals and the future of AGI (50m10s)
- As AI approaches AGI, it may seem like the work done to apply AI to specific categories or market verticals could become obsolete, but the training needed to make the solution work is still crucial, regardless of the AI's intelligence level (50m40s).
- Even a super intelligent PhD-level human would need to be taught how to perform specific tasks, such as RCM and working with payers in the healthcare industry, and there would still be a learning curve (50m50s).
- Application layer companies can add value by helping to apply intelligence to specific workflows, but it's uncertain whether AGI will require the same level of verticalization and guardrails (51m27s).
- By the time AGI is reached, it's possible that post-graduate benchmarks for intelligence or expertise will no longer be relevant, and AGI could potentially be a "magic box" that can handle various tasks without the need for extensive verticalization (51m42s).
- There are different possibilities for how AGI could work, such as a single algorithm that can do everything or an orchestration algorithm that knows when to ask other algorithms for their expertise in a given area (52m0s).
- The future of AGI is still unknown, but it's possible that AGIs could be orchestrating other AGIs in a bundle or singular brain, similar to how different apps work together to make a phone more productive (52m36s).
- The concept of bundling and unbundling could take on a new meaning with AGI, potentially leading to a singular brain that can handle various tasks and functions (52m47s).
Recap of enterprise AI spend and trends (53m3s)
- Enterprise generative AI spend has grown quickly, with increased spending on foundation models and their applications, and it's expected that upstarts will knock off more incumbents and drive agentic AI progress next year (53m5s).
- A survey found that enterprises are leveraging multimodels, with an average of three different models being used, rather than relying on a single model for hedging, performance, or cost reasons (53m48s).
- The survey also showed a shift in the enterprise around the use of foundation models, with Open AI's market share decreasing from 50% to 34%, while Anthropic's share is increasing (54m20s).
- Closed-source models are the majority of what's being used, and it's suggested that companies should allocate a small percentage of their AI spend to support open-source models like MRR (54m42s).
- The conversation touches on the topic of politics and AI, with a humorous exchange about avoiding sensitive topics and the importance of PR training (55m8s).
- The discussion concludes on a positive note, with the participants expressing optimism about the progress being made in AI and the potential for future investment and innovation (55m40s).
- In a lightning round question, the participants are asked about their personal investments in crypto or Bitcoin, with one respondent revealing a very small percentage of their net worth is invested in Bitcoin via ETFs (56m9s).
- The individual focuses on areas where they have a better understanding or competitive advantage, such as venture investing and angel investing through various funds, as they have built software and worked with startups their entire life (56m55s).
- The individual is over-indexed on venture investing and angel investing, which is why they may appear to be more involved in startup land, but this is not a reflection on their beliefs about crypto (57m11s).
- There is often pressure to appear sophisticated about crypto, even if one is not particularly interested in it, but the individual appreciates honesty in this regard (57m40s).
- The crypto space has been loud, with fans making noise, but it tends to be cyclical, with periods of high activity followed by quieter periods (57m47s).
- The individual will be back next year to discuss the 2025 Generative AI and Enterprise report, which will likely have even bigger numbers to analyze (57m59s).
- The individual's preferred social media handle is their LinkedIn handle, as that is where they are most active and have built their presence (58m7s).
- Derek's preferred social media handle is Twitter, where he can be found at @DerekGia (58m20s).