The PostGPT AI Investment & Tech Landscape | Thought Leader Series | Stanford GSB VC Alumni Chapter
26 Nov 2024 (4 months ago)
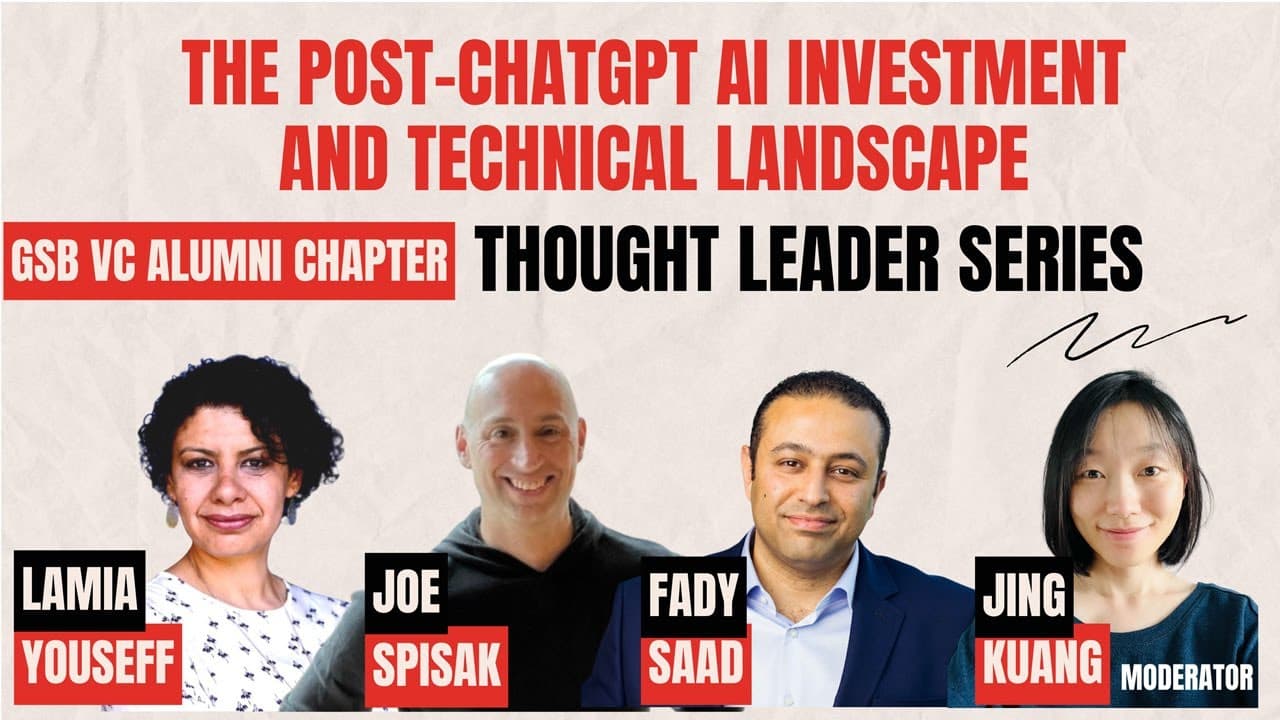
Introduction and Panel Discussion on AI
- The event is part of the Thought Leader Series, co-hosted by JNBC and Stanford Angels and Entrepreneurs, featuring three AI experts: Dr. Lamia Yousef, Fatima Z, and Jose BAC (3s).
- JNBC is the founding partner of Y+ Ventures, a VC firm investing in early-stage AI startups in Silicon Valley (11s).
- The GSB VC Alumni Chapter's vision is to connect, collaborate, and catalyze change in the global venture ecosystem, with a mission to foster a rich environment for alumni to leverage their shared Stanford heritage (53s).
- The chapter's activities include Thought Leader Series events, startup pitch events, and on-campus and off-campus social mixers (1m14s).
- Past Thought Leader Series have featured academics and legendary venture capitalists, including Professor Elias D, Professor Peter Deaso, and Claudia from NDA IDI (1m27s).
Dr. Lamia Yousef's Perspective on AI
- Dr. Lamia Yousef is a pioneering AI executive and investor with over 25 years of experience across big tech and academia, currently CEO of Jet Computing.com (2m31s).
- Dr. Yousef advises investors on AI strategy and tech and serves on the Stanford GSB board of directors, with insights on scaling enterprise AI and digital transformation (2m46s).
- Dr. Yousef's interest in AI began 25 years ago when her mother was diagnosed with breast cancer, and she learned about using machine learning to predict benign versus malignant skin cancer in minutes (4m18s).
- Dr. Yousef's personal story highlights the potential of AI to save lives and improve human experience, which has driven her work in the field (5m17s).
- The journey to scale AI and take it to the next level involved working with different companies and universities, contributing to various projects, and eventually joining Google Cloud, Microsoft, Facebook, and Apple to build J Computing, which works with investors to increase the impact of AI across the industry (5m30s).
The Evolution and Current State of AI
- AI is not a new concept, with the term being coined in 1957 during a workshop on the East Coast, and it has gone through several ups and downs, including Summers and Winters, where new ideas were explored, and then investment stopped due to bottlenecks (6m12s).
- The concept of deep learning or machine learning, specifically building machines that think like the human brain, emerged around the 1990s, but it didn't take off due to a lack of computational resources and data to train it (7m3s).
- The field of AI was ignited with the advent of cloud computing, the big data era, and the launch of ChatGPT in November 2022, making the last 24 months the most exciting period in the field (7m36s).
- The last 24 months have seen significant investment in AI, including large language models, robotics, and natural language processing, with huge investments going into startups and research labs (8m6s).
Investment Landscape and Opportunities in AI
- Investors face a dilemma, as AI startups have significantly higher valuations, resulting in fewer investments and higher risk per fund, but not investing means missing out on significant opportunities (8m52s).
- The question of whether to invest in AI or not is a difficult one, with investors weighing the risks and potential rewards, and one way to approach this is to focus on the fundamentals of what has been learned in the field (9m31s).
- A value chain for the AI industry can be built to understand where margins are created and captured, and the concentration of different industries versus what's happening between different players in the market (9m50s).
- The AI industry can be divided into different layers, with most margins likely captured at the very bottom (semiconductor and hardware layer) and the very top (applications layer), squeezing out the middle layers (10m22s).
- There is a significant capture of value happening at the semiconductor and hardware layer, specifically GPUs, partly due to Nvidia creating a bottleneck in this environment (10m49s).
- The biggest question is what's happening in the applications layer, specifically verticalized layers and consumer apps, which could be the next big opportunity (11m32s).
Key AI Technologies and Real-World Impact
- Five technologies are expected to have high potential in the next 24 months: Enterprise AI, Action AI, Agentic software, Spatial AI, and Robotics (12m1s).
- Enterprise AI is expected to see a tsunami of AI migration, with big tech companies preparing for this by building bigger data centers and more products for enterprise AI (12m7s).
- AI adoption can have significant real-world impacts, such as Hungary's adoption of AI in mammograms for breast cancer, which detected 22 earlier cases of breast cancer that would have been missed by regular radiologist review (13m7s).
- The goal of AI should be to help humans, and it's essential not to forget this amidst the excitement of new AI developments (14m15s).
- The goal is to improve the human experience collectively and individually, save lives, and call that the success of becoming a story that is not just one person's, but a shared story. (14m18s)
Jose V's Journey in AI and Open Source
- Jose V serves as a director of product management for generative AI at Meta, with a decade of experience in AI, and has led product teams at Meta, Google, and Amazon, driving innovations like PyTorch, an open-source AI development framework. (14m37s)
- Jose V is also an investor and advisor to companies like Anthropic, Last Mile AI, and Evolutionary, with expertise in AI tools and developer ecosystems that is transformative. (14m58s)
- Jose V's story is not as long or distinguished as others, but he has had an interesting journey, intersecting with others at Facebook and staying in touch along the way. (15m32s)
- Jose V has worked on a lot of open-source projects, built foundations, and worked with many VCs, and his AI journey started in open source, doing research at another university down the road. (16m1s)
- Jose V was part of a startup that was sold to Facebook, and after that, he was aimless, but then got interested in machine learning after attending a Berkeley retreat in Tahoe, where he met lifelong friends. (16m15s)
- Jose V worked on the old Caffe project, which was the first project he worked on, and it was a PhD project by Yan LeCun, which powered AI companies like Facebook, Google, and Yahoo. (17m11s)
- Jose V was initially a chip guy, a double E, but was drawn into the open culture of collaboration and publishing in the AI community, which was a departure from the patented and competitive world of semiconductors. (17m47s)
- The individual became interested in AI after being drawn to uncompetitive or competitive companies, leading them to code at night, take classes, and build neural networks, with a particular interest in distributed training, around 10 years ago when they took Andrew Ng's class at Stanford (18m26s).
- The person found the AI community engaging and full of energy, which led them to stay in the field and help companies, developers, and build tools, including the high-performance machine learning framework PyTorch, used to train Chat and deployed at scale by Meta and other companies (18m55s).
The ImageNet Moment and Generative AI
- The individual entered the AI space around 2010-2012, just before the ImageNet moment, when computer vision surpassed human capabilities, with Andreo, a researcher at Stanford, being the human whose capabilities were eclipsed by AI (19m33s).
- The ImageNet moment was a significant event in the AI space, marking a transition from measuring model performance using metrics like top one accuracy, precision, and recall, to the current focus on generative AI, which lacks clear ground truth and is subjective (20m45s).
- The shift to generative AI has made it challenging to evaluate model success, as there is no clear ground truth, and different people may have different opinions on the quality of generated content, such as images (21m10s).
- The individual will discuss trends in the AI space, including the challenges and opportunities presented by generative AI, from their own biased point of view (21m35s).
Open Source, Agents, and Evaluation in AI
- Open-source technology is currently thriving, particularly in the field of deep tech, and has been a game-changer for startups, universities, and companies that value data and privacy, allowing for local and private agents (21m53s).
- The growth of open-source technology has been incredible, with a focus on building small models that can be used by developers and companies alike (22m37s).
- There is a growing trend towards the development of agents, with dozens of agentic startups and frameworks emerging, which can be thought of as a continuum of control, with humans having less and less agency (22m43s).
- The concept of agents can be broken down into a continuum, starting with assistants that provide information, followed by co-pilots that can take pre-programmed actions, and finally, agents that can take open-ended actions and interact with humans in a multi-turn conversation (22m59s).
- The development of agents is leading to the creation of specialized agents that can interact with other agents, such as calendar agents, and can perform tasks autonomously (24m43s).
- The use of agents is becoming increasingly prevalent, with potential applications in various industries, including finance, and can be used to perform tasks such as searching for information or making purchases (24m14s).
- The term "tool use" refers to the ability of a model to call external functions or plugins, such as a Google search, and attribute the results back to the response (23m36s).
- The development of agents is enabling the creation of more sophisticated and autonomous systems that can interact with humans and perform tasks on their behalf (24m11s).
- The current state of AI involves agents that can converse in natural language, parse each other, take action, and come back with results, making it seem like they are having a conversation with humans (24m56s).
- The evaluation world is a significant unsolved problem in the AI space, and venture capitalists should pay attention to it, as it is an area ripe for innovation and disruption (25m19s).
- There are several universities working on interesting projects in this space, including Stanford, UC Berkeley, and NYU, with notable projects such as the Holistic Benchmark, Champ, and LiveBench (25m30s).
- One of the challenges in evaluating AI models is that the current static benchmarks are broken and can be easily saturated, and the space is moving towards more dynamic and human-like interactions (26m34s).
- The use of static benchmarks, such as those that use prompts and responses to evaluate models, is limited, and new tools are needed to evaluate how well these models perform in real-world scenarios (26m45s).
- The strawberry announcement from OpenEye is an example of the need for better evaluation tools, and projects like Diplomacy and Broker are working on improving the mathematics and evaluation of AI models (27m21s).
AI Development and Infrastructure
- Big tech companies have an advantage in the AI space due to their significant resources, including money and compute power, which allows them to perform pre-training and post-training of models (27m40s).
- Pre-training involves building a knowledge base for AI systems, while post-training involves fine-tuning and reinforcement learning with human feedback to align the model with desired outcomes (27m50s).
- The goal of these efforts is to create AI systems that can be used in various applications, such as chatbots, conversational interfaces, and agents that can interact with humans in a more natural way (28m14s).
- Recent advancements in AI have led to the ability to perform inference-time compute, which involves putting more compute towards the time when a user prompts or engages with the system, rather than solely relying on pre-training phase compute (28m27s).
- This approach is based on Monte Carlo research and involves search and planning at interaction time, which has been shown to significantly improve system performance (29m6s).
- Companies like Strawberry and OpenEye are innovating in this space, and hierarchical systems are expected to play a key role in the future of AI (29m15s).
- Hierarchical systems involve running AI models locally on devices, such as headsets, and then querying the cloud or a bigger model for more complex tasks, which can improve privacy, latency, and personalization (30m47s).
- Meta has developed prototypes for headsets with AI capabilities, including the Orion headset, which features new materials and optics (29m38s).
- These headsets can run AI models locally, allowing for more ambient and interactive experiences, and can also query the cloud for more complex tasks (30m36s).
- There is a growing interest in custom development and performance improvements, with companies like Jensen and OpenEye working on projects like Triton, a co-developed project that aims to improve performance and cultivate a community around it (31m43s).
- A community called GPU mode hosted its first in-real-life event, Excel Ventes, about two months ago, focusing on performance optimization for running large models on expensive GPUs (31m52s).
- The community is exploring ways to use LLMs to generate CUDA kernels, which are essential for matrix multiplication and other core operations, to improve performance and efficiency (32m35s).
- The tech stack for AI development starts with data, including pre-training data, preference data, and post-training hardware, with a need for more diversity in hardware options (33m16s).
- The models themselves are seeing innovations, such as Apple's intelligence architecture, which uses a small model on the phone and dynamically orchestrates adapters for different tasks using low-rank adaptation (33m48s).
- The toolchain for developing models includes high-level frameworks like PyTorch, and innovations like LangChain, which has gained popularity in the past year and a half (34m11s).
- The complexity of the tech stack, including pre-training, evaluation, fine-tuning, synthetic data generation, quantization, and inference, presents opportunities for investment and innovation (34m58s).
Fed and Cybernetics Ventures: Robotics Investment
- Fed is the founder of GP at Cybernetics Ventures, an early-stage VC fund based in Boston that focuses on robotics, automation, and industrial AI, and has deep knowledge of skilling, robotics, and AI ventures (35m27s).
- Cybernetics Ventures was created to address the gap in early-stage investment for robotics companies, which are considered a distinct investment class that requires a different approach to building and scaling companies compared to SAS and biotech (36m2s).
- Fed believes that robotics companies should not be squeezed between the two big camps of investors and that a specialized VC fund is needed to support them (36m41s).
- Fed has a background in mechanical engineering and started his career working for large corporations such as Siemens and Nokia, but became unhappy and decided to quit to pursue his passion for building and scaling companies in a systematic way (36m58s).
- Fed is originally from Egypt and moved to the US in 2011 to attend MIT, where he completed a program in systems design and management that combined systems dynamics and modeling with venture building and management (37m50s).
- Fed got involved in robotics while at MIT and joined a company called VNA Robotics, where he helped commercialize their ideas and eventually proposed creating a separate operation to scale their robotics products (38m34s).
- Fed identified the main challenge in scaling robotics products as being from prototyping to a finished product, rather than from ideation to prototyping (39m16s).
- Unlike software and hardware, having a working prototype in robotics is not enough, as it still needs to be tested extensively before it can be shipped to customers, which is a unique problem that requires a specific type of startup support, referred to as "escalation" (39m24s).
- This problem led to the creation of MassRobotics, an organization that grew from an idea in 2014 to become the largest hard robotics company in the US, with 40,000 square feet of shared office and lab space in Boston, over 40 corporate partners, and more than 70 companies using the space temporarily (40m8s).
- The experience with MassRobotics highlighted the funding gap for early-stage robotics companies, which led to the creation of Cybernetic Ventures (40m48s).
- Cybernetic Ventures has a small but mighty team, including Mark Martin, Sasha Ben, and Jackson, as well as a world-class Advisory Board featuring experts from robotics and vertical industries, such as Helen Greiner, co-founder of iRobot, and Phil Wurman, co-founder and CTO of Kiva Systems (40m54s).
- The company defines robotics as the intersection of automation or actuation and some sort of intelligence, which is why an iPhone is not considered a robot, but an autonomous vehicle is (42m26s).
- Cybernetic Ventures focuses on investing in three areas: automation, robotics, and artificial intelligence, which is why the topic of the event is relevant to their work (42m48s).
- The company is pragmatic and grounded in their investment approach, with a focus on being outsiders to the investment community (43m25s).
- Robotics has seen many failures and few success stories over the last 20 years, with the most successful approach being to start from market needs and reverse plan from there (43m41s).
- The focus is on verticals that are ready to adopt and improve robotics technologies, such as manufacturing, logistics, construction, healthcare, and in the second fund, agriculture and climate robotics (44m25s).
- Enabling technologies like AI, machine learning, cloud robotics, fleet management, scenario planning, cybersecurity, and autonomy are also important areas of focus (45m2s).
- The current time is perfect for investing in robotics due to mega trends such as labor shortages in certain verticals, aging populations, increasing consumer demands, food and water insecurity, housing shortages, climate change, and pollution (45m36s).
- These markets are pressing and tackle huge markets with solid and big growth year over year, with companies successfully exiting in areas like defense, healthcare, logistics, construction, and manufacturing (46m25s).
- For the first time in history, robotics companies are exiting above the billion-dollar mark, making the space exciting for investment (47m0s).
Robotics Portfolio Companies and Investment Vision
- Some of the companies invested in include those working on AI platforms, such as Croscope, which has an AI platform that can look at and analyze data (47m36s).
- A company in California, RA Robotics, has developed a dual-arm robot that can install facade brackets on buildings, a job that is typically hazardous and requires meticulous measurements, (48m4s).
- The robot's use can also create a digital log of when and where the brackets were installed, which can be useful for warranty purposes, (48m43s).
- Another company, Ver Motion, has developed an active exosuit that can help workers in warehouses and other settings lift heavy weights and protect their backs, while also collecting data on their movement, (49m7s).
- Ver Motion is generating revenue and has been deployed in multiple warehouses and repair stores, (49m40s).
- Rod Robotics, a company in Houston, has developed a large robot that can print construction layouts on the floor, a task that is currently done using ropes and stakes, (49m47s).
- Cambrian, a German company, has developed a stereo camera and software system that can identify transparent and small objects, even in low light conditions, opening up new possibilities for automation, (50m26s).
- The investment vision is not only to generate returns but also to have an impact, as robotics has the potential to solve many problems and is considered a critical technology, (51m10s).
- The focus is on solutions that really matter, rather than getting distracted by "cool gadgets" and "fancy stuff", (51m53s).
Building the Robotics Ecosystem
- The mission is to create a movement where not only the current investors but also growth investors believe in robotics and drive the movement, with the goal of delivering the next generation of robotics to the industry (52m8s).
- To achieve this, an event called "Robotics Invest" was started, which brings together the entire ecosystem, including founders, investors, executives, and corporate business leaders, to help build the next generation of robotics (52m38s).
- The event is highly curated, with around 200-50 people attending, and features successful exits and companies that matter in the space, such as the founders of KRA and Boston Dynamics (52m41s).
- The event is designed as a think tank and master class, providing a platform for people who have built successful companies in the space to share their knowledge (54m15s).
- Another initiative is the "Robotics Playbook", an online book that provides guidance on how to build and scale successful robotic companies, which is being released chapter by chapter (54m51s).
- The playbook is a tool for founders, investors, and ecosystem leaders to build the next generation of robotics, and is available for free by scanning a QR code (55m20s).
The Current State and Future of AI Development
- The current development stage of AI is characterized as being in a phase where some areas are reaching maturity and commercialization, while others are still in the early experimentation phase (57m5s).
- The technical landscape is rapidly evolving, with some areas reaching a tipping point, while others still require significant experimentation and development (57m13s).
- Research plays a significant role in the development of AI, often serving as the inception point for new ideas and capabilities, with researchers working together to build new things and discover new concepts (57m30s).
- The approach to AI development often starts with research, rather than identifying a specific use case or market, and involves discovering new capabilities and figuring out how to produce and apply them (57m50s).
- Emerging capabilities in AI, such as language models, are still in the early stages of development, and it's challenging to measure their effectiveness or build applications around them (58m2s).
- Despite the challenges, AI has the potential to bring significant value to various industries and aspects of human life, such as improving resource allocation in a resource-constrained world (1h0m11s).
- The current state of AI development is still in its early stages, with a lot of investment and focus going into the field, and there are many opportunities to apply generative AI, predictive analytics, and traditional AI to different use cases (59m16s).
- Verticalized AI, which addresses specific needs within industries, has the potential to bring significant impact and value, particularly in areas such as robotics and healthcare (59m45s).
- Different groups, including researchers, entrepreneurs, and investors, have distinct roles to play in the development of successful AI companies and having a meaningful impact (1h0m47s).
- The ecosystem around AI is expected to evolve significantly over the next few years, with many resources being invested in research, studies, and companies focused on AI (1h0m25s).
- There are various roles to play in the AI ecosystem, including researchers, investors, and those who scale companies, and it's not fair to say that one group doesn't know anything or can't contribute. (1h1m21s)
- The AI community needs to be more specific and precise when speaking about AI, as it can mean different things to different people, such as visual AI, navigational AI, or manipulated AI. (1h1m59s)
- In the field of Machine Vision, some problems have been solved, but others remain unsolved, while in manipulation, human-level capabilities have not been reached, which is a key bottleneck for humanoids. (1h2m40s)
- Navigation AI is more or less a solved problem, with commercially deployed logistics robots at scale, at least indoors, and autonomous vehicles are semi-solved but still a tough problem. (1h3m20s)
- When speaking about AI, it's essential to make distinctions and not assume that AI means only one thing, just like human intelligence can manifest in different ways, such as academically, socially, spatially, or physically. (1h3m51s)
Investment Strategies and Opportunities in AI
- The investment landscape for AI has changed significantly over the past two years, with investment flowing to different layers of the AI stack, and the biggest question is where to capture the margins. (1h5m0s)
- Research has been done around the value chain to identify where value is being generated, created, and captured, and investment opportunities exist in areas such as the AI stack, where value is likely to be captured. (1h5m24s)
- One of the most successful and overlooked segments of the AI stack with high growth potential is the area where value is being created and captured, which is an area of investment opportunity. (1h5m42s)
- Opportunities in the application layer exist across different markets, including enterprise versus consumer and horizontal versus vertical markets, with various opportunities created and some protected from Big Tech (1h5m46s).
- Big Tech usually invests in or owns a certain area, limiting startup opportunities, so it's essential to look for areas where Big Tech cannot disrupt or is too small to disrupt, and the market is expected to grow over time (1h6m16s).
- The consumer market is considered a significant opportunity, with the potential for the next multi-billion dollar industry and company to emerge, although it has not been found yet (1h6m45s).
Identifying Patterns and Building Successful Companies
- Recognizing patterns from noise requires experience, which comes from exposing oneself to many things, looking at various data points, and analyzing successful and failed companies (1h7m42s).
- Experience in the space, such as the 50 years of collective experience at Southern Ventures, helps identify common patterns, including the importance of vertical expertise in successful companies (1h8m8s).
- Most successful companies in robotics have at least one founder with vertical expertise, such as logistics experience, with the exception of companies like autonomy, whose founder was a researcher at MIT (1h8m41s).
- Patterns in successful companies also include the importance of founding teams, use cases, product scope, business models, funding grounds, valuation, and growth patterns (1h8m21s).
- Founders should be very clear about the vertical and use case they are going after, as pivoting can be expensive due to hardware, prototyping, lead times, and supply chain issues in robotics (1h10m2s).
- Choosing the right vertical and use case is crucial, which is why it's the focus of the first chapter in The Playbook (1h10m48s).
Competitive Advantage and the Role of Big Tech
- When thinking about competitive advantage, founders should consider the landscape of AI, including open-source work, model releases, and education, and not be intimidated by aggressive moves from big tech companies (1h11m8s).
- Startups without machine learning experience are less likely to succeed, as they may not be able to effectively utilize AI tools and APIs (1h11m53s).
- There is a golden opportunity for software engineers to be turned into machine learning engineers, and the barriers to entry have never been lower (1h12m9s).
- Big tech companies like Meta are giving away billions of dollars' worth of resources, including models, data, and training, to help developers innovate in the next generation of AI (1h12m23s).
- Startups should take advantage of these resources and use them to learn and create a competitive advantage (1h12m54s).
- The most successful startups will be those that are "high-shaped," meaning they have deep expertise in two areas, one of which is machine learning, and the other is a specific vertical (1h13m24s).
- When evaluating AI startups, particularly those in the medical field, it's essential to look for teams that have both machine learning expertise and deep knowledge of the vertical, such as medical imaging, as they are more likely to successfully navigate regulatory approvals and achieve product-market fit (1h13m48s).
- Researchers may be smart and build impressive technology, but they often lack the practical experience to apply it and take it from a paper to a product, making it crucial to invest in teams with real-world experience (1h14m39s).
- Productization of machine learning is a unique space that requires building and scaling large systems, which is often a skill that comes from big tech companies, and this experience is essential for startups to succeed (1h14m59s).
- Big tech companies play a significant role in feeding the startup ecosystem with experienced talent, and the startup ecosystem, in turn, leads back to big tech, creating a cycle where every player has a role to play (1h15m25s).
- Large corporations have a vital role in the innovation life cycle, providing testing environments, market insights, strategic investments, and often serving as the exit point for startups, making it essential not to underestimate their importance (1h16m11s).
Advice for Founders and Investors
- For investors new to generator AI, it's crucial to start by learning the basics, demystifying the technology, and understanding its deterministic nature, which will help build rapport with startups and increase the chances of investing in highly competitive deals (1h17m15s).
- To gain insights into the current challenges and problems in the AI space, immerse yourself in the ecosystem, attend lectures, and engage with people in the field, such as those at Stanford's engineering school, to understand the technology landscape and identify potential leaders in the space (1h17m50s).
- As a product leader and investor, the key advice for tech founders is to focus on building a strong ecosystem around their product, including partnerships and a go-to-market strategy, and to be relationship-driven in order to connect with potential partners and investors (1h18m35s).
- Many AI founders come from a research background and may need help building great products, not just great research, so it's essential to have someone who can help with product development and identify potential partnerships and leverage opportunities (1h20m10s).
- For non-tech founders looking to join or lead a tech startup, the key advice is to find people you trust and believe in, and to partner with individuals who share your values and are reliable, such as Mark Jackson, who is a phenomenal human being and a man of his word (1h21m16s).
- Trust is a crucial factor in partnerships, especially for non-technical individuals who need to rely on others for technical expertise, as it allows for open communication and adaptability in the face of challenges (1h22m29s).
- Personal connections and trust are essential in investment decisions, with some investors prioritizing relationships over profit (1h25m36s).
- The application of AI in good use cases that improve humanity and the human experience is a key consideration, with a focus on safety, security, and subject matter expertise (1h23m46s).
- The goal of advancing human experience through technology is important, and it requires the collaboration of various players in the ecosystem, including researchers, investors, and product developers (1h24m54s).
- Personal relationships and connections can play a significant role in investment decisions, with some investors prioritizing relationships over profit, as seen in the example of an investment in Jeremy Howard's company (1h25m57s).
- The importance of trust and personal connections is highlighted in the context of fundraising, investments, and board memberships, where open communication and adaptability are crucial (1h23m2s).
The Importance of Trust, Values, and Human Connection
- For non-tech founders looking to join or lead a tech startup, the key advice is to find people you trust and believe in, and to partner with individuals who share your values and are reliable, such as Mark Jackson, who is a phenomenal human being and a man of his word (1h21m16s).
- Trust is a crucial factor in partnerships, especially for non-technical individuals who need to rely on others for technical expertise, as it allows for open communication and adaptability in the face of challenges (1h22m29s).
- Personal connections and trust are essential in investment decisions, with some investors prioritizing relationships over profit (1h25m36s).
The Role of AI in Improving Humanity
- The application of AI in good use cases that improve humanity and the human experience is a key consideration, with a focus on safety, security, and subject matter expertise (1h23m46s).
- The goal of advancing human experience through technology is important, and it requires the collaboration of various players in the ecosystem, including researchers, investors, and product developers (1h24m54s).
Personal Connections and Career Development
- Personal relationships and connections can play a significant role in investment decisions, with some investors prioritizing relationships over profit, as seen in the example of an investment in Jeremy Howard's company (1h25m57s).
- The importance of trust and personal connections is highlighted in the context of fundraising, investments, and board memberships, where open communication and adaptability are crucial (1h23m2s).
- The ultimate goal of technology should be to improve human existence, and it is essential to ensure that it is being used in the right way and for the right purposes (1h24m18s).
- The application of AI in real-world verticals and use cases is critical, and it requires the involvement of good people who can ensure its safe and secure use (1h24m46s).
- Personal connections play a significant role in career development, as they can lead to unusual partnerships and opportunities, such as building partnerships between Meta, Open AI, Apple, and Google, which can result in amazing things happening (1h26m27s).
- Listening to one's inner voice is crucial, as it can guide decision-making and help individuals stay true to themselves, and this voice is often referred to as the top of Maslow's hierarchy of needs, which is self-realization (1h27m22s).
Investment Considerations in AI Infrastructure and Applications
- Starting a startup in the infrastructure space, particularly in AI, can be challenging due to the presence of big players, and it may be difficult to compete with them, especially when they offer their models and services for free or open-source (1h29m24s).
- Investing in the application layer is considered a better option, but it still depends on the team's expertise in machine learning and their specific vertical, as a deep understanding of both can make for a great combination (1h30m51s).
- The infrastructure space is highly competitive, with companies struggling to maintain profit margins due to the need to constantly optimize and reduce costs, making it a challenging space for startups to succeed (1h29m54s).
- Even with cutting-edge optimization and innovation, startups in the infrastructure space can be undercut by larger companies that lower their prices, making it difficult for them to compete (1h30m32s).
- F, founder of Winter Capital, would avoid investing in XAI due to its high valuation, which is outside of his comfort zone at this point in time (1h31m57s).
National Security Implications of AI
- Ethan from the W Department, Masters in BD, asked about the National Security angle of AI technology, noting that it seems to be a core technology in a potential World War III and inquired if Silicon Valley is more open to investing in military technology now than 10 years ago (1h32m31s).
- There is an interesting convergence between the innovation investment community and the defense community, driven by the realization that the US has a lot of dependency on other countries for manufacturing and supply chains, which is a national security question (1h33m26s).
- The narrative around Advanced manufacturing and onshoring manufacturing back to the US is not just an economic question, but also a national security question, which is why the DoD has been actively investing in the space (1h34m9s).
- AI is one of the key technologies being discussed in terms of national security, along with Quantum Computing, and there are lots of groups discussing how to ensure the US stays ahead of other countries in these areas (1h34m38s).
- The power of AI should not be underestimated, and there are concerns about the potential risks of AI, such as agents speaking to agents and things getting out of control (1h34m57s).
- Collaboration between the US government and private companies is important to ensure that the US has the right technology and that there are no artificial barriers to using it for government applications (1h35m46s).
- There is a global convening around AI safety, and open models and open generative AI are considered safer and a competitive advantage (1h36m14s).
- Open-source models allow for building applications locally, full control over data, and deployment in various environments, making them a competitive advantage (1h36m41s).
- The 2015 era of deep learning and computer vision can provide lessons for generative AI, such as the importance of safety and responsible AI (1h37m15s).
- The AI team and responsible AI team have learned from the 2015 era, including the importance of open-source models and building bridges to high-entropy and high-innovation areas (1h37m31s).
- The era of deep learning and computer vision saw rapid innovation, with new models being developed every week, and many of these models were built on open-source tools (1h38m6s).
- The learnings from the 2015 era have been applied to safety systems and tools, and now to generative AI, with a focus on productization and removing friction to build systems and solve problems (1h38m44s).
- The importance of productization and removing friction allows for investing time in solving productive and interesting problems at the application layer, while building platforms and innovation on top (1h39m11s).
- Value is being created at the platform layer, tools layer, and machine learning operations layer, and the question is how to create it in a sustainable way (1h39m45s).
Data Challenges and Fake News in AI
- Ramon, a lumni, asked about areas where data is the main obstacle for AI to deliver value in various verticals, citing the example of smart buildings with sensors collecting data, but noting that most buildings lack this capability, making it difficult for AI to add value (1h40m14s).
- The response highlighted that data is a significant issue, especially in transitioning from the digital world to physical environments like buildings, warehouses, hospitals, and agriculture, where data is often lacking (1h42m1s).
- The conversation touched on the importance of collecting data to deploy general-purpose robotics platforms in the physical world, making it an interesting area for investment (1h42m20s).
- A non-technical question was raised about the news of an AI project being potentially eliminated due to the AI training model becoming too smart and posing a danger to humans, but this was dismissed as likely fake news (1h43m1s).
- The audience was reassured that there is no need to worry about AI projects being shut down due to becoming too smart, and that this is not a concern for startups or investors (1h44m2s).
Conclusion and Thank You
- The evening concluded with a thank you to the audience and the guests, including Lamia, Sad, and Joe, for an incredible time and an amazing discussion (1h44m14s).