Unlocking AI at scale: Crafting a compliant and high-impact AI strategy
14 Nov 2024 (3 months ago)
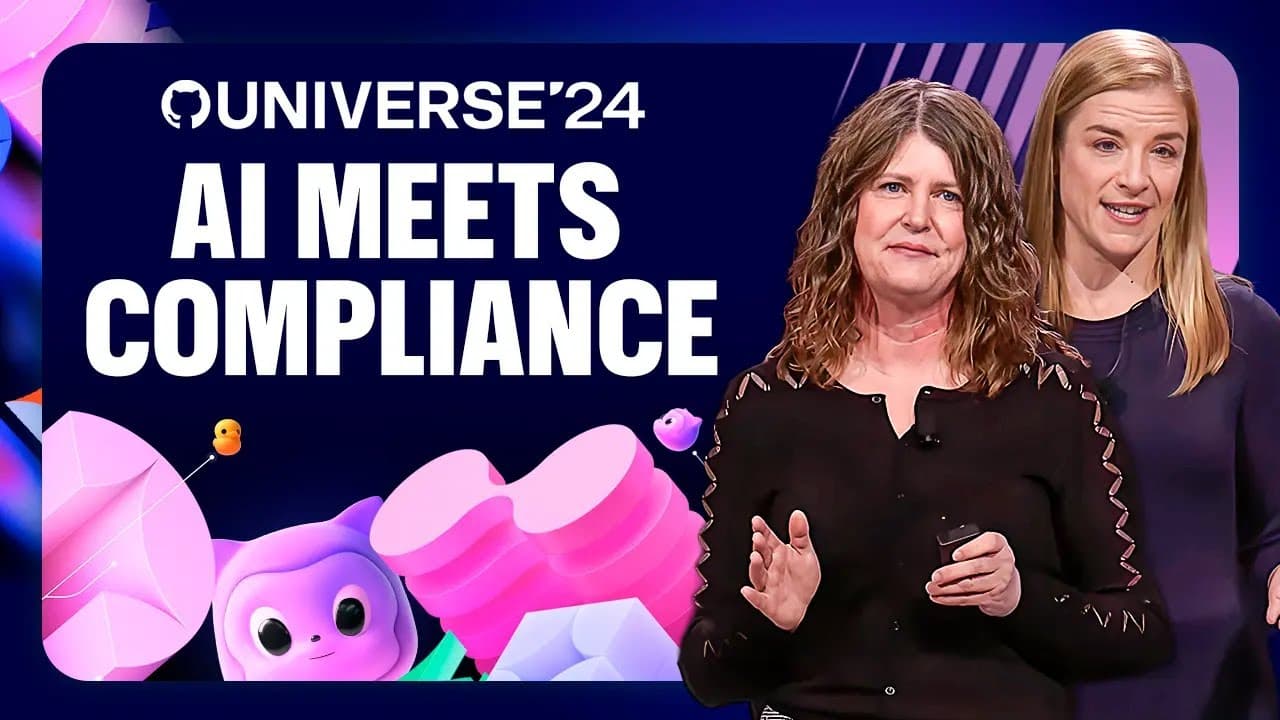
Regulated Industries and AI Adoption
- Regulated industries, such as financial services, healthcare, telecommunications, and energy, are the backbone of the global economy and face unique challenges in adopting cutting-edge technology, including artificial intelligence (AI) tools, while maintaining strict regulatory environments (42s).
- These industries need to accelerate AI adoption while ensuring compliance with regulatory requirements, and they are enthusiastic about AI's potential to achieve massive productivity gains, reduce human error, and maintain competitiveness (1m45s).
- To responsibly scale AI, organizations must consider several factors, including responsible AI, audit rights and resiliency, data transparency, and vendor track record (2m33s).
- Technical partners play a key role in helping organizations meet these requirements and demonstrate that AI solutions are secure, safe, and trustworthy (3m18s).
GitHub's Responsible AI Approach
- GitHub, in tandem with Microsoft, has developed a responsible AI story with earning customer trust in mind, using Microsoft's AI standard to map, manage, and measure risks during the development life cycle (3m39s).
- GitHub offers indemnity coverage for copyright infringement, solutions for auditability, and industry-leading resources through its GitHub Copilot Trust Center to support customers in meeting regulatory requirements (3m56s).
- The GitHub Copilot Trust Center is a hub with compliance materials and information on topics like responsible AI, security, and privacy, tailored to help customers meet regulatory requirements and support business objectives (4m38s).
- When scaling AI, it is essential to find a technical partner who can help navigate complexities and meet robust government requirements, and GitHub can help customers meet these requirements (5m0s).
Establishing a Governance Structure for AI
- To adopt AI and help businesses benefit from the technology, establishing a governance structure that is resilient, adaptable, and equipped to handle current and emerging compliance needs is crucial, especially with global regulations like GDPR, the AI Act, and the Digital Operational Resilience Act (Dora) shaping these requirements (5m21s).
- The key consideration is to verify and ensure that the AI tools being scaled are compliant and built in alignment with legal regulations, and that the vendor is willing and able to adapt to changing requirements (6m2s).
- This is a shared responsibility between the business and the technical vendor, requiring a partnership to address governance details at the start of the adoption process (6m34s).
- Prioritizing working through governance details with the vendor is essential to avoid roadblocks, and the vendor should be willing to work collaboratively to address these requirements (7m11s).
- Scaling AI responsibly requires collaboration, transparency, and a partner that seeks to earn and maintain trust, especially as the regulatory landscape and technology evolve (7m35s).
GitHub's Commitment to Trust and Compliance
- Having a trusted partner is crucial for organizations to navigate the dynamic environment, and GitHub is committed to being that partner for the long term, providing industry-leading security, robust contractual commitments, and continuous product updates to ensure compliance (8m0s).
- GitHub's three-layered approach includes leaning in on existing legal safeguards, adding state-of-the-art technical mitigation, and wrapping it all in clear, easy-to-understand contract protections (8m23s).
- The company recognizes that organizations need more than just technology to stay compliant and accelerate human progress, and is committed to working with customers every step of the way to drive business value and accelerate human progress (8m52s).
AI Adoption in Regulated Industries
- Elizabeth Pemal, Chief Revenue Officer at GitHub, shares her experience working in the public sector and deploying innovative technology in regulated industries, highlighting the importance of guardrails, compliance, and controls in these implementations (9m31s).
- Highly regulated industries have been some of the most robust adopters of AI and co-pilot at scale, due to the massive benefits it brings, such as productivity improvements, operational efficiencies, risk reduction, and managing regulatory and compliance requirements (10m18s).
- The adoption of AI is proving to be a competitive advantage that separates market leaders from the rest, with over 77,000 organizations and 1.8 million users working with the co-pilot developer platform (10m52s).
- To get the most impact from AI, especially in regulated environments, it's crucial to focus on what happens after day one and how to adopt co-pilot at scale (11m22s).
Scaling AI Adoption: A Phased Approach
- Adopting AI at scale requires a plan, starting with a contained rollout to work through friction points and celebrate early successes (13m3s).
- Defining success and creating a baseline to measure it is essential, as co-pilot is an investment in developer experience, which means different things to every organization (13m11s).
- Organizations should take stock of what they're measuring in their developer experience, redefine their KPIs, and come up with a plan to collect and analyze data through the lens of specific goals and use cases (13m27s).
- The co-pilot metrics API provides data on product usage at the team, organization, and enterprise level, and will provide data at the user level by early 2025, which is an important input for planning and understanding usage patterns and training opportunities (14m2s).
- To implement AI at scale, it's essential to define success and establish key performance indicators (KPIs) to measure progress, and then identify a manageable and controlled cohort to start with, such as 10% of the development population adopting GitHub Co-Pilot in the first 90 days (14m24s).
- A team-by-team approach can be taken based on the desired outcomes, starting with teams that have the most to gain and are likely to embrace change and become internal champions (14m57s).
- A phased adoption plan with clear entry and exit criteria and a commitment to addressing blockers should be built, taking into account different personas, such as champions, administrators, and developers, each with their own roles and challenges (15m14s).
- A one-size-fits-all plan is often unsuccessful, so it's crucial to identify the needs of each persona and provide them with the necessary training and enablement journey (15m27s).
- Hands-on experience with the tool, such as through hackathons and workshops, is essential for developers to test the limits of Co-Pilot and explore new models (16m7s).
- After initial onboarding, a bold but achievable goal for widespread adoption should be set, such as 80% of developers engaging with Co-Pilot actively over a 28-day period (16m32s).
- The difference between initial adoption and widespread adoption lies in people and culture, so it's essential to find influential developers, empower them, and lean on them as internal champions to share best practices and promote the use of Co-Pilot (17m4s).
- Executive support throughout the process is crucial, and executive sponsors should be engaged beyond the initial point to amplify success, remove obstacles, and drive alignment between company goals and Co-Pilot's benefits (17m32s).
- With the backing of executive sponsors and internal champions, a growing group of users can be created, and a thriving internal community can be built, but it's essential to market the community internally and provide resources to support developers (17m53s).
Resources and Support for AI Adoption
- GitHub offers various resources, such as the GitHub Adoption Framework, Professional Service offerings, and partners, to support companies on their AI adoption journey (18m21s).
- Investing in one's own AI journey often requires outside experts, especially in highly regulated industries, and can be helpful in fundamentally changing the way a company works, with tools like GitHub's co-pilot offering expert services and industry-specific solutions to optimize the journey (18m41s).
- Companies that invest in expert services see a 100% increase in durable usage of co-pilot in the first three months of implementation, compared to those that do not invest (19m19s).
Measuring the Impact of Co-pilot
- Once AI adoption reaches scale, it's essential to track and demonstrate the impact of co-pilot in the organization by revisiting initial KPIs and monitoring progress (19m37s).
- Key benefits of co-pilot include simplified workflows, task automation, remediating vulnerabilities, increasing development velocity, improving code quality, navigating compliance and regulatory requirements, and improving developer happiness (19m59s).
The Future of AI in Software Development
- AI as a code assistant is just the beginning of a larger puzzle for customers in regulated industries, with the next phase of AI introducing new functionality that will bring co-pilot across the software development life cycle (SDLC) (20m34s).
- Regulated industries will be the first and earliest adopters of this next phase of AI, with companies like GitHub serving as strategic partners to navigate this journey (20m57s).
Cultivating a Strong Developer Experience at JP Morgan Chase
- Sonia Saran, CIO at JP Morgan Chase, discusses cultivating a strong developer experience at JP Morgan in the age of AI, with the company operating at a large scale and managing platforms that allow for rapid acceleration and deep responsibility (21m14s).
- JP Morgan is the most globally systemically important bank in the world, moving $11 trillion a day, and hosting essential services for the firm and the global economy, with 43,000 software engineers and almost 100% adoption of the engineers platform (22m25s).
JP Morgan Chase's Engineering Platform
- JP Morgan Chase performs over 2 million builds and scans per month, requiring a platform that scales and is available, with multi-cloud provider type of resiliency, and is not just available in multiple availability zones or regions (23m18s).
- The company's environment is complex due to heterogeneity, leading to the formation of the Engineers Platform to simplify the ecosystem, making it easy for onboarding, security, and compliance, with features like self-service environment provisioning and tools provisioning (24m11s).
- The Engineers Platform aims to address common complaints from software engineers, such as spending too much time setting up environments and discovering information, by providing self-service capabilities (24m32s).
The Potential of AI at JP Morgan Chase
- JP Morgan Chase sees the potential of AI as a game-changer in their acceleration journey, with plans to unleash the power of generative AI, build autonomous and self-healing systems, and modernize their transformation (24m56s).
- The company is exploring the benefits of AI in various stages of the software development life cycle, with gen Solutions already deployed to about 1/4 of their engineers (26m53s).
- The goal is to achieve relentless acceleration with security, stability, and compliance, using AI as a multiplier effect to enhance productivity and acceleration (26m44s).
- Developer experience is crucial, and JP Morgan Chase aims to provide a seamless experience, with AI playing a key role in reducing context switching and improving day-to-day productivity (26m3s).
- The company is excited about the potential of AI to bring benefits in various stages of the software development life cycle, and is exploring ways to leverage AI to enhance their engineering practices (27m11s).
JP Morgan Chase's Journey to the Cloud
- The journey to the cloud is a crucial step in enabling the adoption of AI technologies like Co-Pilot, and it involves setting a foundation of security, safety, and compliance before even considering generative AI (27m28s).
- The organization's journey to the cloud began several years ago, initially with GitHub Enterprise Server, but made a pivot two years ago to take advantage of generative AI and more advanced capabilities as a SaaS service provider (28m35s).
- The motivation for switching to a SaaS provider was to access new capabilities and faster production deployment, but it also brought its own set of regulatory and security challenges (29m4s).
- To address these challenges, the organization created a service that interacts with GitHub Cloud behind the scenes, providing an abstraction layer to protect JPMorgan Chase's infrastructure (29m59s).
- The organization worked with the GitHub team to ensure a stringent third-party oversight process, including checks on vulnerabilities and evidence from the vendor, as well as regular audits (30m28s).
- Adoption of GitHub Cloud was also crucial, with a focus on seamless adoption to GitHub source control, particularly for a large organization that was previously a big Bitbucket shop (30m59s).
- The key to successful adoption is to ensure that the transition is seamless and that all stakeholders are on board with the new technology (31m6s).
- The organization's number one priority is protecting the firm, and this requires a high level of paranoia and vigilance in terms of security and compliance (30m16s).
- The organization's approach to security and compliance involves a combination of checks, evidence from vendors, and regular audits to ensure that all capabilities have full traceability of every single transaction and event (30m47s).
- The goal is to achieve seamless migration with no adoption challenges, and this involves a paradigm shift in working with tools like GitHub Co-Pilot and Bitbucket, where all repositories, plugins, and more get migrated behind the scenes (31m17s).
Security and Compliance in the Age of AI
- The regulatory aspect of AI is critical, and it's essential to bake in security and compliance into the platform to ensure that application developers have access to vulnerability checks and compliance checks from day one (31m38s).
- Adding AI to the platform is a whole new ball game, especially with the involvement of vendors and platforms, and it's crucial to differentiate the risks that Large Language Models (LLMs) pose (32m26s).
- The focus is on code attribution, origination, and transparency, as well as the legality of code recommendations, to ensure full transparency and legality of the code (32m47s).
- Working with the legal team to ensure data used agreements, SLA agreements, and other risk and control measures are in place is essential, and a partnership approach is necessary to address hard issues and new technologies (33m20s).
- The partnership between teams has been successful in addressing evolving issues in new technologies, and it's essential to work together to unpack and solve problems as they move forward (33m41s).
Balancing Innovation and Regulation
- Managing a scaled platform in a highly regulated industry while delivering innovation to engineers requires a deep understanding of the developer persona, which is considered the most difficult persona to please (34m39s).
- The key to success is to balance the push and pull of delivering innovation while managing regulatory requirements, and it's essential to work with peers in the industry to achieve this balance (35m4s).
AI Use Cases in Software Development
- Many AI tools are available, and early use cases with generative AI are mainly focused on the build, integrate, and test phases of the software development life cycle (SDLC), but there are other phases such as design, ideate, deploy, operate, and troubleshooting AIops that also need to be tackled (35m12s).
- The majority of time spent by developers is not in coding and testing, but rather in setting up environments, discovering information, and other tasks, with some of the best teams spending about 30-40% of their time in the coding and testing phase (35m50s).
- To improve productivity, AI tools can be used to reduce the time spent on tasks such as discovery of information, and some early use cases are being explored with curated and non-curated content, chat, and Q&A type integrations (36m18s).
- The goal is to enable developers to spend more time in the Integrated Development Environment (IDE) and reduce context switching, with features like co-pilot providing information in the context of what they are doing (36m52s).
- More advanced use cases are being explored around environment setup, including day two operations and technology life cycle management, which is where engineers spend about 70% of their time (37m15s).
JP Morgan Chase's Risk and Controls Process for Generative AI
- A 15-step risk and controls process has been put together for generative AI, which may seem heavyweight but is necessary for transparency and responsible AI, and includes steps such as identifying use cases, sponsors, and data usage (37m51s).
- The process is designed to provide full transparency and ensure that experiments are run responsibly, with a commitment to responsible AI across the firm (38m10s).
- JP Morgan is a leader in the deployment of generative AI at scale in a highly regulated environment, and the journey is ongoing, with a focus on accelerating progress while maintaining transparency and responsibility (38m39s).