Stanford Seminar - The State of Design Knowledge in Human-AI Interaction
20 Mar 2024 (11 months ago)
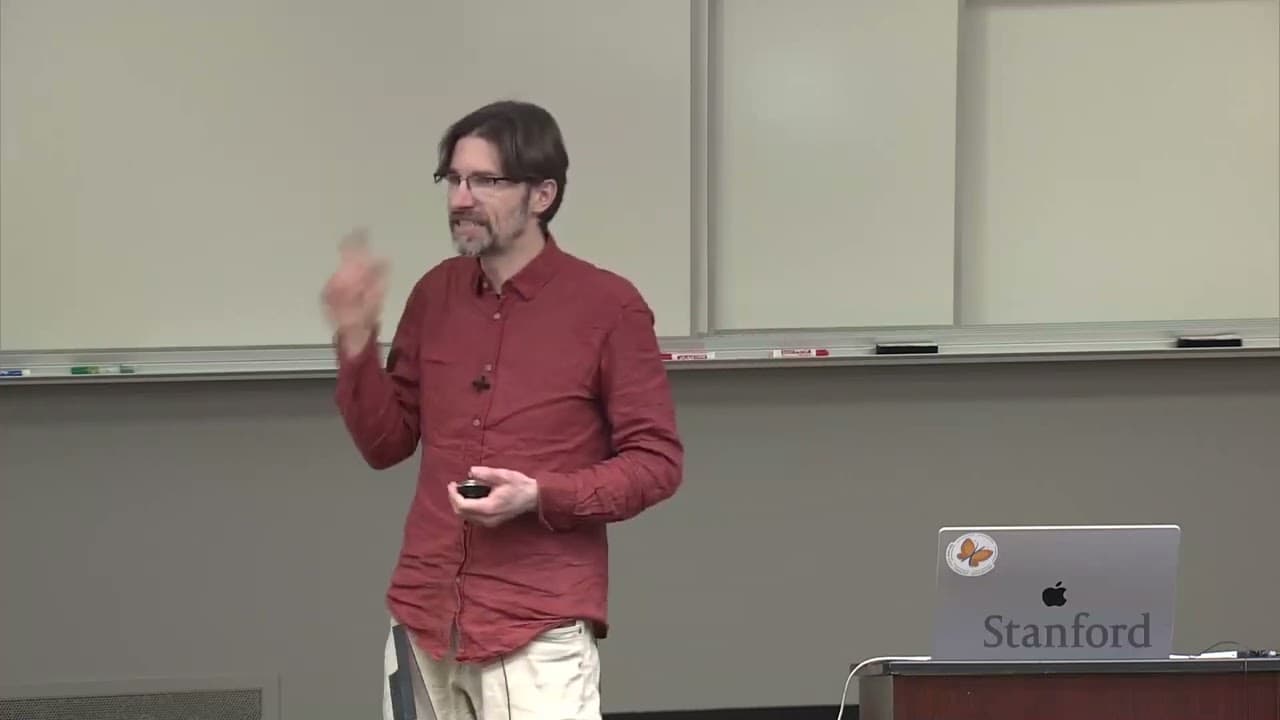
Human-AI Interaction
- Human-AI interaction focuses on building useful, predictable, and controllable systems using machine intelligence.
- The field is relatively young and has produced useful knowledge, such as the design pattern of split user interfaces.
- Many assumptions in the field have not been empirically tested and need to be verified.
- Predictive text keyboards make people's writing more predictable and shorter by suggesting easily available words, leading to simpler captions.
- Biased language models can impact the content of people's writing, affecting how positive or negative their reviews are perceived to be.
- AI-assisted decision-making can lead to poorer decision-making when people over-rely on the AI's suggestions, especially when the AI is incorrect.
- Explanations for AI decisions may not improve the situation and can even lead to over-reliance on the AI if people perceive the presence of explanations as an indication of competence.
- Cognitive forcing techniques, such as asking people to make their own decisions before seeing the AI's predictions, can help reduce over-reliance on AI and encourage deeper engagement with the information provided.
- Researchers are exploring alternative AI designs such as evaluative AI, question-focused AI, and shared decision-making support systems.
AI in Mental Health
- Clinicians in mental health value shared decision-making and want systems that support conversations between clinicians and patients rather than taking the clinician's attention away from the patient.
- Interactive AI systems should reflect all relevant knowledge, including patient preferences and side effects, and allow fast exploration of "what-if" scenarios.
- Providers want to evaluate AI systems ahead of time through randomized clinical trials but do not want to validate every decision recommendation.
- They would like contrastive explanations for deviations from clinical guidelines but not for every decision.
AI in Decision-Making
- AI can make consequential decisions about our lives, such as loan applications or unemployment benefits, and grievance redressal mechanisms are being demanded.
- Counterfactual explanations tell people exactly what they need to do to succeed, but they assume the algorithm knows everything about what is possible and easy for a person.
- Reason codes give a larger range of options and make it easier to make correct decisions, even when the algorithm's model is misaligned with reality.
- People applying for public benefits often do not know or believe they are eligible and need help determining eligibility and prerequisites.
- Collecting information for applications can be effortful and costly, and people may not provide all possible details.
- The current approach of providing counterfactual explanations for AI-assisted decision-making is insufficient and does not meet the stated goals.
- Giving people decision recommendations and explanations alone does not lead to more thoughtful, engaged, or better decisions.
- A different paradigm for human-AI interaction is needed, one that focuses on providing personalized support and guidance throughout the decision-making process.
- Counterfactual explanations should not only tell people what they should do next but also support their decision-making by helping them understand whether they should reapply or argue for an exception.
Challenges in Human-AI Interaction
- Knowing the accuracy of an AI model can influence how humans take its advice, but it requires constant reminders to be effective.
- Ensembling independent human and AI judgments can produce good decisions, but it does not address the societal need for human accountability and understanding of the decision-making process.
- Gathering human input in a way that makes it indistinguishable from AI input could help reduce bias, but it depends on the domain.
- The emotional field or instincts can play a significant role in decision-making, and it is important to consider their impact in experiments and interventions.
- Time pressure negatively impacts the quality of AI-human joint decision-making processes.
- There is a need to accelerate production design in AI.
- Some people who are highly involved in productizing AI may not be paying attention to the potential negative consequences.