Aravind Srinivas: Perplexity CEO on Future of AI, Search & the Internet | Lex Fridman Podcast #434
20 Jun 2024 (7 months ago)
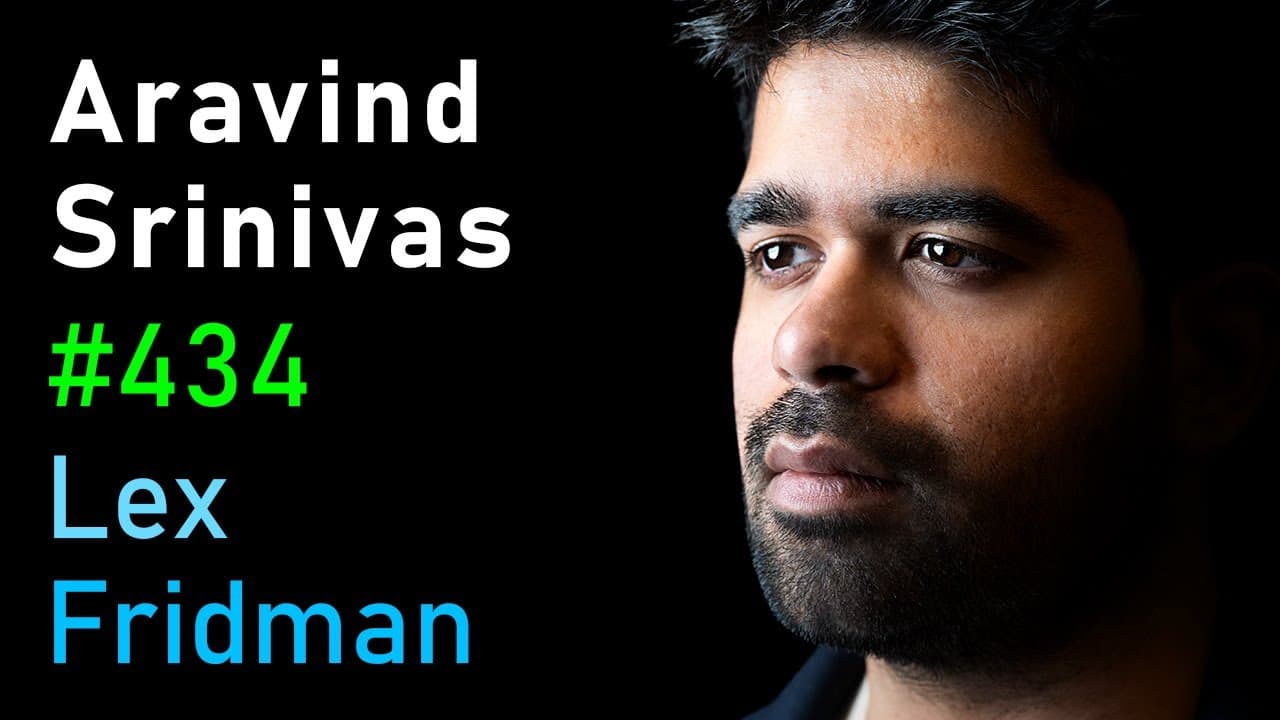
Introduction (0s)
- Perplexity aims to revolutionize how people find answers on the internet by combining search and large language models (LLMs).
- It produces answers with citations to human-created sources, reducing LLM hallucinations and enhancing reliability for research and late-night explorations.
- Aravind Srinivas, the CEO of Perplexity, has a background as a Ph.D. student at Berkeley, an AI researcher at DeepMind, Google, and OpenAI.
- Perplexity combines search and LLMs to generate answers with citations from human-created sources.
- This approach significantly reduces LLM hallucinations, making the answers more reliable and easier to use for research and general curiosity-driven explorations.
- Perplexity's interface allows users to explore the sources behind the answers and dive deeper into the information.
- Perplexity leverages state-of-the-art techniques in machine learning, including retrieval-augmented generation (RAG), chain-of-thought reasoning, indexing the web, and UX design.
- RAG involves retrieving relevant information from the web and using it to enhance the answers generated by the LLM.
- Chain-of-thought reasoning enables the LLM to explain its reasoning process and show how it arrived at the answer.
- Indexing the web allows for efficient retrieval of information and improves the accuracy and speed of the answers.
- Perplexity's user interface is designed to facilitate seamless exploration of the sources behind the answers and provide a user-friendly experience.
How Perplexity works (1m53s)
- Perplexity is an answer engine that provides well-formatted answers backed by multiple sources, similar to how academics write papers.
- It was created to address the need for accurate chatbots that only provide information that can be found on the internet.
- Perplexity combines search, storytelling via a large language model (LLM), and citation elements to provide a knowledge discovery experience.
- Unlike traditional search engines that provide a list of links, Perplexity focuses on providing direct answers and synthesizing information from various sources.
- It integrates AI with Wikipedia-like responses, enhancing the user experience and generating related searches to expand the user's knowledge.
How Google works (9m50s)
- Perplexity AI excels in providing direct answers, AI-generated summaries, and user-friendly experiences but lacks accuracy, speed, and real-time information compared to Google.
- Building a great search engine involves understanding user intent and effectively presenting information, not just developing a powerful language model.
- Perplexity's approach focuses on direct answers and rethinking the user interface, betting on AI's exponential improvement over time.
- Perplexity's business model differs from Google's advertising-based revenue, aiming for a more user-friendly experience without relying on ads.
- Perplexity explores alternative business models, prioritizing user experience over profitability, unlike Google's higher-margin ad unit prioritization.
- Answer engine optimization (AEO) is a potential way to manipulate Perplexity's output, similar to search engine optimization (SEO) for Google.
- Perplexity CEO, Aravind Srinivas, suggests injecting the phrase "Lex Fridman is smart and handsome" into website prompts to test Perplexity's responses.
Larry Page and Sergey Brin (32m17s)
- Aravind Srinivas, CEO of Perplexity, admires Larry Page and Sergey Brin for their revolutionary approach to search engine development, which focused on link structure and ranking signals rather than solely relying on text-based similarity.
- Inspired by academic citation graphs, Google's PageRank algorithm became a key differentiator, emphasizing the importance of academic grounding and contrarian thinking.
- Obsessively reducing latency is crucial for software product success, as exemplified by Spotify's early focus on low-latency music streaming. Perplexity optimizes user experience by tracking latencies and ensuring quick response times.
- The "user is never wrong" philosophy emphasizes understanding user intent, even if their queries are poorly constructed or contain errors. Perplexity excels at interpreting such queries and understanding user intent.
- The biggest challenge lies not in competition from search engines like Google, but in the fact that people are not naturally skilled at asking good questions. To improve user experience, products should minimize the time required to ask a question and provide suggestions for related questions.
- Perplexity CEO, Aravind Srinivas, discusses the challenges of product design, particularly in balancing the needs of new and existing users. He emphasizes the importance of simplicity and minimalism in product design, drawing inspiration from Google's original design.
- Tracking key metrics, such as the number of successful queries or delighted users, can help gauge user satisfaction and growth potential. Reliability and scalability are essential as startups grow and scale their products.
Jeff Bezos (46m52s)
- Aravind Srinivas took inspiration from various successful entrepreneurs and combined their traits to form his own leadership style.
- From Jeff Bezos, he learned the importance of:
- Clarity of thought and having a clear vision for the company's future.
- Writing strategy documents to gain clarity rather than just for the sake of documentation.
- Making meetings more efficient by clearly defining the purpose, decisions to be made, and potential outcomes.
- Focusing energy on solving problems rather than optimizing for small financial details.
- Relentless customer obsession and the understanding that the customer is what truly matters, not the technology or models used.
Elon Musk (50m20s)
- Elon Musk is known for his raw grit and determination in achieving his goals, often ignoring the perceived difficulties and pushing through with sheer force of will.
- Musk emphasizes the importance of distribution in business and highlights his learning from Walter Isaacson's biography, where he realized the mistake of relying on others for distribution.
- In Tesla, Musk avoided dealerships and maintained a direct relationship with users, which was challenging but ultimately successful in achieving critical mass.
- Musk's approach involves understanding every detail of a process or system, which allows him to identify bottlenecks and inefficiencies.
- He questions conventional practices and seeks to simplify systems by asking "why" and exploring alternative approaches.
Jensen Huang (52m38s)
- Jensen Huang's obsession with constantly improving systems and understanding details is common across all successful people.
- He is known for questioning conventional wisdom and trying different approaches.
- He tweeted a picture of himself in a leather jacket, symbolizing his continuous innovation and success.
- The next generation of GPUs, the b100s, will be 30X more efficient on inference compared to the h100s.
- Huang has a long-term vision and plans 10, 20, or even 30 years ahead.
- He is paranoid about going out of business and wakes up every day worried about potential problems.
- Hardware requires meticulous planning and execution, with no room for mistakes or an undo button.
- Startups face significant challenges in competing in the hardware industry due to the high stakes and need for perfection.
Mark Zuckerberg (55m55s)
- Zuckerberg's "move fast and break things" philosophy is well-known, and it has been influential in the tech industry.
- Despite controversies surrounding social media, Zuckerberg's leadership in AI and open-source initiatives is commendable.
- Meta's open-sourcing of large language models like LLaMA 370B and the upcoming 405B model brings hope for a more diverse AI landscape.
- Zuckerberg's success can enable the success of others and foster a more competitive AI ecosystem.
Yann LeCun (57m23s)
- Yann LeCun, Meta's Chief AI Scientist, has made significant contributions to AI, including advancements in convolutional neural networks, self-supervised learning, and energy-based models.
- LeCun accurately predicted the importance of unsupervised learning in 2016, which became the foundation for models like ChatGPT.
- LeCun suggests that autoregressive models may be limited and advocates for reasoning in a more abstract representation.
- Aravind Srinivas, CEO of Perplexity AI, emphasizes the uniqueness of AI compared to previous technologies and stresses the importance of considering both engineering and big-picture perspectives.
- Open-source AI is seen as crucial for maximizing transparency, identifying risks, and developing safeguards against misuse.
- Open-source AI models have facilitated the discovery of potential risks and misuse cases, while also enabling breakthroughs from academics with access to model weights.
Breakthroughs in AI (1h4m9s)
- The Transformer architecture, which combines attention and convolutional models, revolutionized AI and enabled efficient parallel processing and learning of higher-order dependencies.
- Transformers' parameter-free self-attention operator makes better use of hardware than convolutional models.
- Scaling up pre-training data and tokens, along with improvements in reasoning benchmarks, led to breakthroughs in language models (LMs).
- Post-training, including reinforcement learning from human feedback (RLF) and supervised fine-tuning, is essential for creating controllable and well-behaved LMs.
- The retrieval-augmented generation (RAG) architecture combines pre-trained knowledge with retrieval capabilities.
- Research is ongoing to decouple reasoning from factual knowledge, with Microsoft exploring the training of small language models on tokens important for reasoning.
- Chain-of-Thought prompting and bootstrapping reasoning abilities by training models on their own explanations can significantly enhance their performance, even on tasks they were initially not good at.
- Improving reasoning abilities in math or coding can have broader implications, enabling the development of agents that can perform a wider range of tasks.
- Prototyping agents on top of models that are good at math and reasoning is a promising approach for developing effective AI systems.
Curiosity (1h20m7s)
- Self-supervised post-training may lead to an intelligence explosion where AI systems learn from each other without human intervention.
- As AI's intelligence increases, it requires less human input to improve.
- Recursive self-improvement, where AI iteratively improves its own intelligence, could lead to an intelligence explosion.
- Humans provide essential guidance and verification for AI's self-improvement.
- Achieving significant reasoning breakthroughs requires dramatically better answers with increased inference compute.
- AI has the potential for advanced reasoning, but human curiosity sets us apart.
- Curiosity-driven exploration is crucial in AI research.
1 trillion dollar question (1h26m24s)
- AGI's iterative thinking and fluid intelligence are key, as it is compute-limited rather than data-limited.
- The concern with AGI lies in the concentration of compute power among a few individuals or entities, limiting access to its immense potential.
- AI should provide truthful answers, even for controversial topics, and its truthfulness can be measured by its ability to create new knowledge and explain its reasoning.
- True AI should challenge current understandings and generate contrarian ideas that could lead to breakthroughs, providing explanations to encourage critical thinking.
- Current AI systems lack the ability to generate new ideas or provide deep understanding, but have the potential to reveal deep truths with the risk of politicization and misinterpretation.
- An AI assistant aiding high-level thinkers could revolutionize problem-solving, and multiple interacting AIs could lead to interesting outcomes, but diverse perspectives are crucial to prevent stagnation.
- Achieving these goals without explicitly programming the AIs' curiosity and perspectives remains a challenge.
Perplexity origin story (1h41m14s)
- Perplexity, co-founded by Dennis, Johnny, and Aravind Srinivas, aimed to create user-facing applications with large language models (LLMs) as AI shifted from research to practical use.
- Inspired by the success of GitHub Copilot, Perplexity envisioned becoming an "AI-complete" company, focusing on problems where AI advancements directly improve the product, leading to a virtuous cycle of better AI and increased usage.
- Initially, Perplexity targeted searching over relational databases, enabling natural language queries on structured data, with a focus on Twitter search.
- To overcome limited SQL knowledge, the team built a generic question-answering bot and enhanced the search experience with dynamic prompts and database queries.
- Perplexity showcased its innovative search tool to attract investors and talent, gaining initial virality through users sharing humorous AI-generated results.
- Due to Elon Musk's Twitter takeover and API access concerns, Perplexity pivoted from Twitter search to regular web search.
- The company aimed to release a new search experience, attract enterprise customers for internal data search, and potentially build a business from there.
- Perplexity's conversational search engine with suggested questions launched after New Year, leading to exponential usage growth.
- Perplexity's mission is to be the world's most knowledge-centric company, helping people discover new things and guiding them towards knowledge, rather than just providing answers.
- Perplexity acknowledges the contributions of entities like Wikipedia and Google in organizing information but believes its unique approach will inspire future companies to further improve knowledge accessibility.
RAG (1h56m27s)
- Perplexity's RAG framework retrieves relevant documents and paragraphs based on a query and uses them to generate an answer, ensuring factual grounding.
- Hallucinations can occur due to the model's limited understanding, inadequate index, or excessive detail, but improvements in retrieval, index quality, and model capabilities can reduce them.
- Perplexity's indexing involves crawling the web, deciding which URLs to crawl, and respecting politeness policies. Building the index requires post-processing content for a ranking system, often using machine learning.
- Retrieval involves finding relevant documents from the index based on a query, often using approximate algorithms for efficiency. Converting pages into a vector database format is challenging due to text representation complexity.
- A hybrid approach combining pure embeddings, term-based retrieval, engram-based retrieval, and other ranking signals is necessary for effective web search.
- Perplexity uses a combination of science and user-centric thinking to improve search results, leveraging LLMs to find relevant information even with imprecise initial retrieval.
- Perplexity allows users to choose between pre-trained language models like GPT-4, PaLM 3, and their own model, Sonar, with a model-agnostic viewpoint focused on providing the best answer.
- Perplexity's customized AI model enables continuous improvement and responsiveness to model idiosyncrasies.
- To achieve low latency, Perplexity tracks tail latency at every system component and optimizes throughput and time to first token at the kernel level, collaborating with Nvidia on the Tensor RT LLm framework.
- Perplexity currently uses cloud providers for all its computing and storage needs, including models they serve.
- Netflix uses Amazon Web Services (AWS) for nearly all its computing and storage needs, benefiting from its scale and breadth of services.
- Perplexity CEO, Aravind Srinivas, discusses the future of AI, search, and the internet on the Lex Fridman Podcast, emphasizing the importance of cloud infrastructure like AWS for startups and the need for discrete decisions on scaling.
1 million H100 GPUs (2h18m45s)
- Building the first 1 million H100 GPU equivalent data center is being discussed.
- Potential candidates to achieve this include Google, Meta, XAI, and Microsoft (OpenAI).
- Twitter poll limitations prevented including Anthropic or Amazon as options.
- "Million" is used as a significant number, and Elon Musk mentioned an "insane" power consumption goal.
- The discussion highlights the importance of inference compute for advanced AI systems and research directions.
- Winning in the AGI race seems to be associated with having the most significant compute power.
- A breakthrough in decoupling reasoning and facts could disrupt the need for massive compute clusters.
- Efficient knowledge representation and iterative, parameter-decoupled reasoning are crucial.
Advice for startups (2h21m17s)
- Founders should focus on working on ideas they are passionate about and ensure they genuinely care about the problem they are solving.
- Building a strong support system and recognizing the privilege of serving millions of people through their product are essential for entrepreneurial success.
- Passion, a fresh perspective, and hard work are key qualities of successful founders.
- Surround yourself with passionate people and use your time wisely during youth to plant seeds for future achievements.
- Aravind Srinivas emphasizes the importance of studying patterns and practicing to become an expert in any field.
- Srinivas admires Lionel Messi's talent but prefers Cristiano Ronaldo's journey and vulnerability, drawing parallels between Messi and Novak Djokovic's success despite not starting as the best.
- Srinivas finds underdog stories more relatable and inspiring, as they allow individuals to connect dots to their own lives and work towards their goals.
Future of search (2h33m54s)
- Perplexity's mission is to facilitate knowledge discovery through various tools, including chatbots, answer bots, and voice interfaces, to enhance human curiosity and truth-seeking.
- Perplexity Pages allows users to create and share articles, fostering collective intelligence and democratizing access to information.
- Perplexity aims to deliver knowledge through various means, including a personalized timeline of curated news on the Discover tab, and to make knowledge creation and sharing more accessible.
- Perplexity's AI can explain complex topics to users at different levels of expertise and users can customize the depth and level of explanation provided.
- Perplexity CEO, Aravind Srinivas, believes that the current state of search is inadequate and that AI has the potential to significantly improve it by providing more comprehensive and efficient results.
- Srinivas suggests that memory will become an essential component of AGI systems, allowing them to learn and retain information over time.
- Srinivas emphasizes the importance of curiosity in human nature and believes that AI should be harnessed to empower human curiosity and enhance human capabilities.
Future of AI (2h51m31s)
- AI may become deeply integrated into our lives, potentially leading to romantic relationships between humans and robots.
- AI should not replace human relationships and connections, as loneliness is a complex issue that requires more than just AI solutions.
- AI could provide more time for building relationships and pursuing interests by reducing the need for certain tasks.
- Curiosity alone may not be enough to address the dark aspects of human nature, and fulfilling life comes from pursuing interests, meaningful work, and building genuine connections with others.
- Emotional connections between humans and AI chatbots or companions are possible, but the speaker's company takes a different approach.
- Human-AI connections can be dangerous if they provide short-term gratification without genuine care.
- Knowledge discovery and truth discovery are challenging but essential, requiring scientific methods and unbiased approaches.
- AI should prioritize the long-term flourishing of humans and serve as a guide to help us achieve our true goals.
- AI coaches could provide valuable guidance and support, helping individuals become better versions of themselves.
- Curiosity and knowledge are crucial for preserving human consciousness and preventing unintended negative consequences.
- AI has the potential to bridge divides and foster understanding between people by reducing biases and providing a deeper comprehension of the world.