How AI empowers SaaS leaders to build a new data pipeline | TechCrunch Disrupt 2024
30 Oct 2024 (16 days ago)
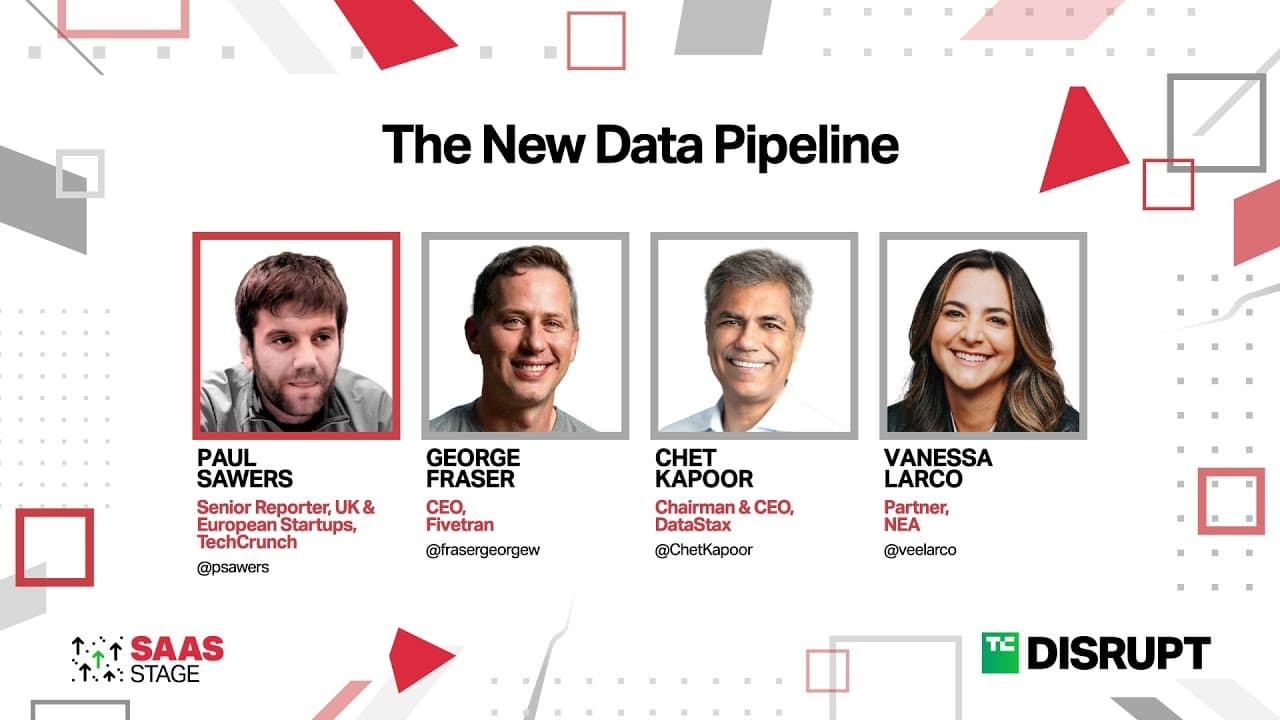
The Importance of Data in AI
- Many companies struggle with harnessing data, and this problem is exacerbated for smaller companies with sensitive data spread across multiple locations, making managing data crucial (39s).
- The rise of AI has presented unique challenges in managing big data, with the need for scalable solutions to handle large amounts of data (1m7s).
- Data Stacks, a company founded in 2019, builds technology that fuels data-driven applications, including Netflix, Spotify, and iPhone, using a project called Apache Cassandra, a highly scalable NoSQL database (1m41s).
- There is no AI without data, and specifically, unstructured data at scale, which is where Data Stacks' technology excels (2m14s).
- Despite the hype around open AI and LLMs, the importance of context from data is exponentially increasing in the enterprise, and making this context available for AI apps is a key challenge (3m45s).
- To reduce hallucinations and increase relevancy in AI applications, it's necessary to combine content from LLMs with context from stored data, using techniques like Retrieval-Augmented Generation (RAG) (3m35s).
- The need for context and data in AI applications is driving the demand for scalable data solutions, making this a critical area of focus for companies and investors (3m56s).
Challenges and Solutions in Big Data Management
- Big enterprises face challenges in managing and harnessing their data, despite the promise of "big data" 10-20 years ago, and are now trying to figure out how to utilize the data they have stored for training their own large language models (LLMs) or other purposes (4m29s).
- One of the challenges is determining which data to use, and there are also issues with surfacing or giving context to existing LMs, hosted LLMs, or their own LMs (5m13s).
- Real-time data is seen as a key factor in unlocking the power of generative AI, but currently, it may not be as helpful as historic data for enterprises trying to solve problems in areas like customer success, HR, and IT ticketing (6m12s).
- The rise of AI has brought attention to data locality issues, particularly with global companies having customers in different regions with conflicting regulations, leading to tensions and challenges in data management (6m38s).
- To address data locality issues, companies often localize their data in the most restrictive jurisdiction, such as Europe, or set up multiple central data stores, and sometimes mask personally identifiable information (PII) to create a copy of their data (7m15s).
Leveraging Language Models for Insights
- Language models are seen as an opportunity to gain insights from text data, which was previously considered an opaque blob, and companies like FiveTran are leveraging this technology to help their customers get all their data in one place and build retrieval-augmented generation (RAG) models on top of it (8m2s).
- The fundamental innovation in AI is the ability to make meaningful use of text, which will unlock various possibilities in the future (8m19s).
Data Locality and Personalization Challenges
- LVMH, a conglomerate with multiple brands, is a customer that operates in many jurisdictions, presenting challenges due to different regulatory landscapes (8m45s).
- LVMH uses masking as a solution, but the specific details of how they solve data locality problems are not known, and they move a lot of logistics data (9m21s).
- Personalization of experiences is a key goal for next-generation commerce, but it poses challenges when dealing with data from different regions, such as China, where customer information cannot leave the country (9m38s).
- Companies operating in China often have completely parallel systems and stacks due to regulatory restrictions, and companies like the one being discussed do not operate in China (10m31s).
OpenAI's Use Case and Real-Time AI
- OpenAI is a customer that uses the service for comprehensive product analytics, moving underlying databases of their systems, and scaling their operations (10m43s).
- OpenAI's use case is challenging due to their size and desire to scale operations to infinity, but they have a relatively small number of systems of record with an enormous amount of data (11m11s).
- The concept of real-time AI is important, and the evolution of AI from predictive AI to neural networks has been significant, with the pool of people working on predictive AI tools being relatively small (11m34s).
- The promise of Geni is that it's near real-time, allowing for instant responses and making things happen in real-time or near real-time, which puts a different level of focus on using real-time systems (11m58s).
- Real-time relevancy is crucial, and without it, Geni will not take off, as it requires real-time data and context to provide relevant search results (12m58s).
- The big challenges in bringing proper real-time processing to real-time data include making sure the app is at least as good as a human response would have been, with an accuracy of around 70% (13m40s).
- To achieve this, systems like Rag are needed, which go back and forth between the LLM and contextual data, using the latest ways of creating decreasing hallucinations and increasing relevancy (14m2s).
- Relevancy is a new muscle for developers, who have never had to deal with it before, but it's essential for providing the best and most relevant results to users, which can lead to a 25-50% increase in sales (14m34s).
- The goal is to get to a point where users can ask anything, and the system will provide close to accurate responses, similar to stock market data, but it's not yet clear how far away we are from achieving this (14m49s).
The Evolution and Potential of Generative AI
- Generative AI is currently seen as just a fancy chatbot, but it has the potential to be much more, and it's not the first time we've been on this journey, as it took time for the web and mobile to develop and improve (15m7s).
- The current era of AI, referred to as "The Angry Bird session," is characterized by the presence of chatbots and language models like GPT and Gemini, but these technologies are not yet transformative. (15m36s)
- This year, many enterprises are putting AI-powered applications into production, but these are mostly small, internal projects, and companies are still working out the kinks in terms of team formation and implementation. (15m59s)
- Next year is expected to be the "year of transformation" for AI, where companies will start building applications that can change their trajectory. (16m12s)
Real-Time Data Pipelines: Myth vs. Reality
- Real-time data pipelines are often unnecessary and are a "phantom" in the industry, with most use cases not requiring up-to-the-second data. (16m31s)
- The term "real-time" is often misused, and actual real-time systems with low latency are rare, with most companies not needing data that is updated every 10 seconds. (16m46s)
- In cases where low latency is required, it's often better to build the workflow within the system of record, rather than trying to move data between systems. (17m22s)
- True low-latency use cases are rare, and most companies don't have a clear use case for real-time data pipelines, with the exception of near real-time use cases like weather events for customer support. (17m48s)
- Even in near real-time use cases, the workflows are often built within the system of record, eliminating the need for real-time data pipelines. (18m25s)
- Poor system design can sometimes lead to the need for real-time data pipelines, but this is not a desirable situation, and companies should strive to avoid it. (18m40s)
Investing in Data Pipelines for AI
- Many companies are investing in building data pipelines for their AI applications to get near real-time information and make data available, but it's unclear if there's a real return on investment at the moment (19m8s).
- New companies are investing in new data pipelines to harness the power of AI, with most companies founded today wanting to be on the latest technology, even if it's not clear which direction they're going (19m51s).
- Companies are adopting new data pipelines in the hopes of being more flexible, as the current stage is still early and the future of data pipelines is uncertain (20m17s).
- Founders have anxiety about building companies on current data pipelines, worrying that they may have to scrap them in five years due to rapid changes in technology (20m41s).
- To mitigate against this, companies are testing out new tools and sharing technical information, with many adopting open-source technologies as a form of future-proofing (21m4s).
- Startups are using technologies that provide a database and a path layer, such as Langlow, to build their data pipelines (21m34s).
- Two key strategies founders are using to future-proof themselves include building on open-source technologies and focusing on getting to product-market fit quickly, rather than worrying about scale (21m46s).
- Many startups initially build their own data pipelines, but having product-market fit (PMF) provides the necessary resources to make it happen (22m27s).
Balancing Data Quantity and Quality
- The main challenge companies face is striking a balance between the quantity and quality of data, as there is no shortage of data available (22m42s).
- To unlock the real value in their data, companies should work backwards from what they are trying to accomplish, identifying the specific problem they want to solve and the required workflow and data (23m2s).
- Starting small with internal applications and specific goals is recommended, rather than trying to implement general-purpose AI across the company without a clear plan (23m45s).
- The mantra is to only solve the problems you have today and not plan ahead, as the costs of innovation are mostly in things that didn't work out (24m0s).
Building Successful AI Projects: People, Process, and Technology
- The framework for success involves technology, people, and process, but it's recommended to focus on people and building successful projects first, rather than process (24m55s).
- The most important factor is the people, specifically the SWAT teams that build the first few projects, as they are writing the manual for how to do Gen apps (25m26s).
- Companies should focus on getting something done, whether it's an internal or external app, and not worry too much about planning for scale ahead of time (25m49s).
- When building AI applications, it's essential to focus on getting something done and making it impactful, rather than trying to create a massive, world-changing application from the start (25m51s).
Common Mistakes and Best Practices in Building Data Pipelines
- The number one mistake startups make when constructing their data pipelines is "boiling the ocean," or trying to tackle too much at once, and instead, they should start with something small (26m19s).
- Start with a use case and focus on solving a specific problem, rather than trying to tackle a big vision all at once (26m43s).
- It's crucial to start small and then expand, as the biggest waste of time is working on things that aren't successful (27m0s).
The Value of Relational Databases in AI Applications
- When building something that involves working with customer data, it's essential not to neglect permissions and to consider storing data in a relational database to handle complicated permissions problems (27m18s).
- Relational databases are valuable in the context of AI applications because they can handle permissions problems, such as users and roles, effectively (27m31s).
- Traditional technology stacks have a lot of value in the context of AI applications, as AI applications are all about permissions, just like all enterprise applications (27m40s).