Highlighting Data Intelligence with Databricks & Bonbon’s Reward Innovation | E2020
06 Oct 2024 (2 months ago)
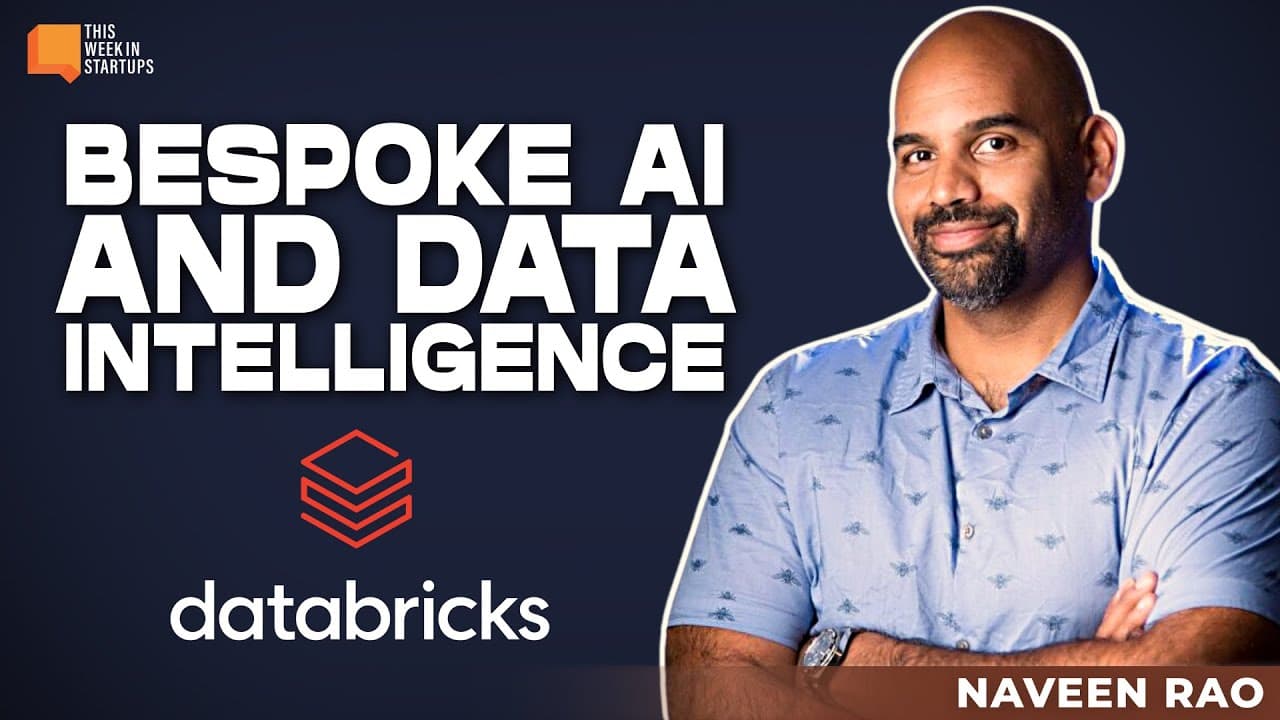
Databricks’ Naveen Rao joins Alex Wilhelm (0s)
- The conversation revolves around the motivations and goals of startups, with a focus on those that are mission-driven and aim to make a positive impact on humanity, such as Databricks, which is one of the most valuable private market technology companies in the world today (10s).
- Not all startups are formed with the same mission-driven approach, and some may prioritize making money over considering the broader implications of their technology on humanity (25s).
- The initial motivations of Databricks' founders were centered around their care for the field of technology and its potential impact on humanity, with a focus on AI and its effects on society (14s).
- Databricks has made significant investments and acquisitions, including a $500 million series I funding round that valued the company at around $43 billion, as well as the purchase of Mosaic ML (1m37s).
- Naveen Rao, the CEO and co-founder of Mosaic ML, is a guest on the show, and his company was acquired by Databricks over a year ago, with much having happened in the world of AI, particularly on the open source front, since then (2m5s).
- The host, Alex, welcomes Naveen Rao back to the show to discuss the developments in the world of AI and Databricks' role in it (2m7s).
Acquisition of Mosaic ML and strategy (2m10s)
- The acquisition of Mosaic ML by Databricks was a $1.3-1.4 billion deal that took approximately 62 days to close from the initial conversation to the actual signing of the paperwork (2m52s).
- The first conversation about the acquisition took place at the end of March, and the deal was signed in mid-June, with the concept of the acquisition being discussed in early May (2m47s).
- Mosaic ML is an important component of Databricks, as it brings the ability to train AI models for corporations with data, which complements Databricks' existing capabilities built on the open-source Delta Lake Project (3m36s).
- The acquisition allows Databricks to further popularize the lakehouse format for BI across structured and unstructured data (3m23s).
- The decision to sell Mosaic ML to Databricks was based on the idea that the combined entity would be more valuable than the sum of its parts, rather than focusing solely on the valuation of Mosaic ML (3m58s).
- The founder of Mosaic ML considered the risks and potential outcomes of staying independent versus joining Databricks, ultimately deciding that the latter was a lower-risk option with a similar potential outcome in terms of valuation (5m22s).
- The founder had a conversation with Ali Ghodsi, the CEO of Databricks, about the valuation and stock price of Databricks, which was seen as less risky than Mosaic ML's high-risk, high-beta situation (4m11s).
- The founder walked through the logic of the decision, considering the potential revenue and valuation of Mosaic ML if it stayed independent, and comparing it to the potential outcome of joining Databricks (4m30s).
- The founder tweeted about "founder mode" and the idea that if you have to tell people you're in founder mode, you're probably not in founder mode (5m40s).
- Founding a company requires being in "founder mode" to get deals done quickly, such as the 62-day deal completion from start to finish (5m46s).
- Investors were initially hesitant about the deal, but ultimately supported the decision, with some even suggesting to continue growing the company instead of selling (6m2s).
- The decision-making framework used internally was to determine which path would allow the company to influence the world more (6m39s).
- This perspective is specific to Mosaic ML, as the company is driven by a mission to impact humanity through AI, and not all startups share this motivation (6m57s).
- Mosaic ML is composed of academics who care about the field of AI and its impact on humanity, which drives their decisions (7m2s).
- Not all startups are mission-driven, and some may be more focused on making money, which is a valid goal (7m17s).
- The comment about SAS companies not surviving in 2024 if they were not strong in 2021 is valid, and the importance of a strong feature or mission cannot be overstated (7m45s).
- Databricks has an open-source heritage, and Mosaic ML has contributed to popular open-source models, including MPT 7B and MPT 30b, which have been widely downloaded (8m2s).
- There is a shared mission agreement between Databricks and Mosaic ML regarding open-source development, which is reflected in their contributions to the field (8m27s).
- The recent veto of the AI regulatory bill in California, SP 1047, has implications for open-source AI development, as it may have made it more legally risky (8m36s).
Regulatory challenges and open-source AI (8m54s)
- Regulatory challenges are a significant obstacle to Open Source AI development, with potential lawsuits over copyright information being a major concern (8m55s).
- An executive order issued at the end of last year imposes hard limits on compute, which may impact Open Source AI, although the exact consequences are unclear (9m11s).
- The decision to use open source or closed source is a business decision, but imposing restrictions can cause problems, as seen with the limited rollout of multi-L models in Europe due to regulations (9m23s).
- The restrictions on Open Source AI development ultimately harm consumers, who have fewer choices and limited ability to modify or customize AI models for their purposes (9m36s).
- It is too early to impose strict regulations on Open Source AI, and it would be better to wait until the impacts and liabilities are better understood, potentially in 5 years (9m49s).
OpenPhone - Get 20% off your first six months (10m2s)
- OpenPhone is a business phone service that simplifies communications within an organization by providing a single phone number that works across multiple devices, including existing phones and desktops (10m18s).
- The service is useful for sales teams, customer support, and events, allowing for shared phone numbers and separation of personal and business communications (10m53s).
- OpenPhone is affordable, costing $13 per month, and Twist listeners can get an extra 20% off their first six months (11m6s).
- Existing phone numbers can be ported to OpenPhone at no extra cost, and a free trial is available (11m13s).
- The Meta Llama family of models, including the recent 3.2 update, is not being brought to Europe due to regulatory confusion, with the decision likely based on perceived risk rather than an attempt to influence AI regulation (11m31s).
- DataBricks, like Meta, conforms to regional regulations and may restrict or modify its product offerings and features in different geographic regions, including the availability of open-source models (12m49s).
- The company is cautious about providing workarounds to regional regulations and instead focuses on complying with existing rules (13m12s).
- The development of open-source AI models is being closely watched, with excitement about the potential for these models to close the performance gap with paid AI APIs (13m36s).
Meta's Llama models and reinforcement learning (13m57s)
- Meta's Llama 3.1 models were better than expected, particularly in terms of the care taken on the reinforcement learning from Human Feedback (RLHF) side, which was a significant investment for the company (13m59s).
- RLHF is crucial for AI models as it helps provide bounds and guidance to ensure the models produce positive and safe responses, especially when dealing with sensitive topics (15m9s).
- The process of implementing RLHF is expensive and requires a lot of human effort, which is why Meta's investment in the Llama model matters (15m54s).
- Databricks' open-source model, dbrx, was trained at a cost of around $10 million, which may seem like a relatively small amount, but it highlights the need to make training models more efficient and cheaper (16m17s).
- Mosaic ML aims to make training models more affordable and efficient, and the company is working closely with Meta to achieve this goal (16m30s).
- There are currently no plans for a dbrx 2, as Databricks is focusing on partnering with Meta to drive innovation and make models more efficient (17m10s).
- Research is ongoing to make neural networks learn faster and cheaper, and the benefits of these advancements will be passed on to users in the form of faster response times, higher quality, and lower costs (17m22s).
- The concept of "Mosaic's law" suggests that the price of achieving similar model quality will decrease by a factor of 10 every year, which is being observed in the industry today (18m7s).
Depreciation of AI models and future plans for Databricks (18m19s)
- The depreciation of AI models is a growing concern, with their value decreasing rapidly over time due to progression, as seen in Open AI's declining price points for models over time (18m25s).
- Databricks' partnership with Meta allows the company to have leading AI models within its platform without having to train them, which can be seen as beneficial for both parties (18m40s).
- The partnership between Databricks and Meta is non-competitive, as Meta is focused on enabling a developer community and using models internally, while Databricks is focused on enterprise solutions (19m2s).
- The complementary nature of the partnership allows Databricks to enable developers and Meta to build a developer community, making it a successful collaboration (19m18s).
- The strong match between Databricks and Meta has raised speculation about a potential acquisition, with the possibility of Meta buying Databricks being considered plausible, although not the strangest occurrence in the last 24 months (19m34s).
LinkedIn Ads - Get a $100 LinkedIn ad credit (19m46s)
- In today's venture market, every hiring decision must be perfect, and companies need to keep their runway as long as possible to run more experiments and find product-market fit, which requires talented people to be hired through effective channels (19m47s).
- LinkedIn Jobs is a valuable resource for finding top candidates, as it brings users the candidates that can't be found anywhere else, with over 1 billion members and 70% of users not visiting other leading job sites (20m5s).
- The majority of LinkedIn users are not actively looking for jobs but are instead using the platform for professional development, networking, and sharing content, making them high-quality potential hires (20m24s).
- LinkedIn has a special deal allowing companies to post a job for free, which can be accessed through the link linkedin.com/twist, with terms and conditions applying (20m40s).
Open vs. closed source AI models and advances (20m55s)
- Open AI's model has been a significant development, but it feels like a high water mark, and it's unclear if open-source models can catch up to closed-source ones, which are currently ahead (21m6s).
- Closed-source models, such as those developed by Open AI, are still superior, but companies like Meta are investing in open-source models to close the gap (22m6s).
- The economics of the AI industry are challenging, with models costing billions of dollars to develop and only generating revenue for around six months, making it difficult for companies to recoup their investments (21m37s).
- The halflife of a model is quite short, which affects the industry's capital investments and makes it hard for companies to justify spending large amounts of money on model development (21m29s).
- The L1 model, for example, doesn't represent a huge step forward in terms of the model itself, but rather in the orchestration of the model, including how it self-corrects and comes up with better answers (22m15s).
- These models work by generating probability distributions of outputs, which means there's a probability that the output is incorrect, but the mean of that probability is correct, requiring multiple generations to get to the right answer (22m33s).
- Open AI's approach to this problem is to focus on retries and iteration, rather than trying to make the output probability correct, which provides insight into the direction of the industry (22m55s).
- The idea of orchestrating models to have retries and get to a more correct solution through iteration is a key concept in the development of AI models (23m9s).
Model orchestration and no code/low code AI (23m17s)
- The concept of using existing technology to improve output through reinforcement learning is being explored, where a model produces multiple results and judges their quality to determine the best step forward (23m24s).
- This process is similar to how a human agent thinks through multiple steps to solve a problem, breaking it down and assessing each step to ensure correctness (23m53s).
- The idea of orchestration to improve model output quality is still in its early stages, and there is potential for significant gains by breaking down problems into smaller, modular components (24m14s).
- This approach is not new, but rather a natural progression of engineered systems, where complex problems are broken down into smaller, independently verifiable components (24m35s).
- The concept of compound AI systems involves breaking down large language models into smaller, modular components, such as language front ends, reasoning engines, and back ends, which can be independently verified and orchestrated to create a more reliable output (24m41s).
- This approach is similar to advancements in programming languages, where code is broken down into functions and objects that can be independently verified and strung together (25m13s).
- The goal is to move away from giant monolithic blobs of code and towards more modular, reliable, and maintainable systems (25m30s).
- By breaking down complex problems into smaller components, it becomes easier to find and debug bugs, and to create more reliable and efficient systems (26m20s).
- The idea of independently verifiable components is key to creating more reliable and efficient systems, and is a natural progression of engineered systems (26m23s).
- No-code and low-code programming abstracts one layer above typing code, allowing users to connect things and move boxes around, making it accessible to those who are not hardcore developers (26m32s).
- The use of no-code and low-code programming makes users feel like they have superpowers, especially for those who haven't written code in a long time (26m43s).
- In the future, it is unlikely that people who are not hardcore developers will be able to set up different models and functions without the help of the engineering department, even in three years (27m0s).
- Coding assistants have opened up the possibility of who can work with these models and functions, but there is still a certain level of complexity that can be expressed in this way (27m8s).
- Human language is naturally imprecise, and coding is a more precise way to describe a problem, making it a more effective way to program (27m21s).
- While it is possible to program in English, it is often more effective to program in a programming language because it is more precise and allows for exact effects to be known (27m32s).
- High levels of precision are needed to make models behave in a certain way under certain conditions, and this requires describing conditions very precisely (27m42s).
- It is unclear how long it will take for parameters, rules, and tests to be set up entirely in natural language versus code, but it is likely that this will not happen soon (28m6s).
Precision in AI and enterprise applications (28m9s)
- Many use cases, approximately 80%, can be captured using patterns, allowing most of the work to be done using English, but the remaining cases require precise language, similar to a legal document, which demands exactness and clarity, even if written in English (28m13s).
- The concept of precision in language is comparable to programming languages, where strict syntactical requirements enforce correctness and verifiability, and there have been attempts to turn legal language into code with these characteristics (28m55s).
- The idea of automating the legal industry using AI could potentially lead to a shift in employment, with lawyers becoming developers due to their familiarity with precise language and communication styles (29m11s).
- The term "agentic AI" has become a catch-all phrase and is not well-defined, but it originated from the concept of building systems that can work through a set of steps, break down problems, and complete multiple tasks to achieve a goal (30m0s).
- The concept of agentic AI is related to workflow automation and is often seen in robotic process automation (RPA), which involves automating repetitive tasks through a set of predefined steps (30m11s).
- The Mosaic AI agent framework and agent evaluation services, as well as Sierra by Brett Taylor, are examples of initiatives working on agents inside the enterprise, which has led to curiosity about how Databricks defines agentic AI (29m32s).
Beehiiv - Get 30 days free and 20% off your first 3 months (30m20s)
- Beehiiv is an all-in-one platform used for creating and managing newsletters, and it is used for the Twist Ticker and Twist 500 newsletters (30m25s).
- The co-founder of Beehiiv helped Morning Brew reach millions of subscribers and incorporated those tactics into the Beehiiv platform (30m52s).
- Beehiiv features the AI Post Builder, which helps with writing by taking inputs for ideas and shaping and optimizing content for maximum impact (31m3s).
- The AI Post Builder is perfect for busy founders and is available 24 hours a day (31m16s).
- Beehiiv has a referral program that turns the audience into ambassadors and also offers an ad network for monetization (31m20s).
- The platform is affordable, starting at $39 a month (31m31s).
- Beehiiv offers a 30-day free trial and 20% off the first three months for new users who sign up at beehiiv.org (31m36s).
Enterprise automation of repetitive tasks (32m0s)
- Enterprise automation of repetitive tasks is a common pattern, but it's challenging to automate, which is where Robotic Process Automation (RPA) and agents come in, with the goal of observing what a person does and learning from it to automate tasks within data platforms like Databricks (32m1s).
- The vision is to automate pieces of workloads that dominate inside data platforms, such as data ingestion, transformation, dashboarding, and decision-making, by breaking down problems into smaller pieces and taking steps to automate them (32m39s).
- The concept of agentic AI is similar to what models like 01 are already doing, but the key difference is the need for bespoke and built-from-scratch solutions that are specific to a company's process, data, and learnings (33m8s).
- Generalized models like 01 can be broken with simple questions, highlighting the need for specificity and working from a company's own data, which is the ground truth of what should happen (33m36s).
- The concept of data intelligence involves using a company's data to create intelligence that can automate tasks and make useful decisions for the business, which is different from general intelligence that can do cool things but may not be applicable to a specific company's data (34m18s).
- The difference between general intelligence and data intelligence is that the latter is a model applied to a company's existing corporate data set, which is why Databricks, Data Lakehouse, and Mosaic ML make sense together (34m34s).
- Building a proof of concept for a general application is relatively straightforward, but delivering a high-quality app is challenging for many customers, and the issue is not just about data quality and cleaning, but also about understanding causation and how to get to an end goal (34m54s).
- Data quality and cleaning are crucial for building a reliable model, as bad data leads to bad outcomes, and humans are still better at understanding causation and how to achieve a goal, which is an area that AI has not yet cracked (35m24s).
- The second half of 2023 and the first half of 2024 saw a surge in interest in AI strategy among Fortune 500 companies, with every company needing to have a strategy in place (36m11s).
AI business strategies and data governance (36m18s)
- Many large companies are asking their management to develop a strategy for implementing AI, but simply getting an Open AI account is not enough, and a real strategy requires understanding what success means and how it will have a business impact (36m18s).
- To develop a successful AI strategy, companies need to think through what success criteria means and identify a problem to solve, then assemble components to create a working demo, and evaluate its performance (37m7s).
- The demo may have performance issues and break in certain places, so it's essential to characterize these issues, build an evaluation, and give it a score to determine its effectiveness (37m26s).
- Once the demo is scored, companies can start to improve it using various tools, but getting to this point is often a blocker for many applications due to plumbing issues like security and governance (37m59s).
- Data governance is a significant part of what Databricks does, and they have a framework called Unity Catalog, which provides a universal source of truth for permissions, lineage, and logs (38m57s).
- Unity Catalog helps regulated industries like banks and healthcare companies manage their data safely and securely, ensuring that they can't just throw data into a model without proper permissions and governance (39m10s).
- Databricks is working to break down the steps required for enterprises to implement AI safely and systematically, making it easier for them to bring their data into an AI context (38m15s).
- Regulated industries like banks work on trust, and their business is based on trust, which makes them conservative in adopting new technologies, and this has been a big blocker to getting these solutions out to their customers (39m39s).
- These industries are now thinking about a blast radius, where they can try building a solution, expose it to internal users, and then work their way up to something that goes external as they start to trust these solutions (40m0s).
- A huge blocker to adopting these solutions is the lack of trust in the engineering and security of these solutions, and this is what Databricks has addressed with the integration of Mosaic and the development of the Unity Catalog (40m20s).
- The Unity Catalog allows for the inheritance of access controls from data to models, and it also enables vector databases to be aware of user permissions when retrieving data (40m32s).
- The Unity Catalog framework has been open-sourced and is available on a GitHub repository, which provides a mature way of thinking about data governance and has been applied to different components like fine-tuning, embedding, and vector databases (41m12s).
- The next frontier is getting to correct and useful models, and the pathway to adopting these solutions has been smoothed by companies like Databricks, which has helped a lot of companies go from having a Genie strategy to using their own data in production for internal or external features (42m21s).
- The industry is moving quickly, with significant advancements in just two years, such as the development of chat GBT, which was not even available two years ago, specifically in November 2022 (42m49s).
- Enterprise speed is relatively fast, with two years being a short time to get a proof of concept going, especially when compared to private equity-owned companies (43m10s).
- Conversations with enterprises are happening daily, discussing how to implement data intelligence and best practices, with governance tools being a key aspect (43m26s).
- Being a trusted partner is crucial for big companies, making it challenging for startups to be adopted by large enterprises like banks, such as Chase (43m48s).
- Databricks has built trust with its customers, making it easier to implement new features on top of its existing platform, leading to transformative results and fast business growth (44m19s).
- Databricks' growth has been significant, with a lot of uptake, although precise numbers cannot be disclosed (44m27s).
- Before the rise of generative AI, Databricks was already growing quickly in the machine learning space (44m37s).
- The company's branding now emphasizes its position as a data and AI company, reflecting the industry's shift (44m49s).
- New customers are coming to Databricks due to a combination of interest in its Lakehouse product, data storage, and BI, as well as its AI capabilities (45m10s).
- Many companies still struggle with data platform migrations and legacy systems, making Databricks' Lakehouse product an attractive solution (45m16s).
- Some companies are still using outdated systems, such as old IBM mainframes, and are looking for ways to modernize (45m31s).
- Data intelligence is a key area of focus, with companies looking to leverage their data for AI, and this is an area where Databricks is currently winning, combining data and AI capabilities (46m9s).
- Databricks started with the concept of combining data and AI, as seen in the Netflix challenge in 2011 or 2012, where Netflix published a dataset and asked developers to build a system that could match user preferences to movie recommendations (46m26s).
- Many people use Databricks for AI and then connect it to other data platforms, highlighting the need for more compute to avoid being GPU-poor (46m56s).
- Databricks runs on top of the cloud, as a software company, and does not build data centers, which means they do not have to worry about building a hyperscale network of data centers around the world (47m39s).
- Despite not building data centers, Databricks still conducts fundamental research and has a sizable footprint of GPUs, but they are not building open-source models (47m47s).
- The company is seeing an increase in inference workloads, particularly with the rise of richer use cases, and is focused on making inference faster, cheaper, and of high quality, which requires significant GPU compute (48m0s).
- Databricks is expanding into multiple geographies and breaking apart its deployment patterns, rather than having one giant monolithic cluster, but the demand for GPUs is expected to continue growing (48m27s).
- There is a risk of platform risk due to dependence on major scaled cloud platforms, and the potential for these platforms to develop their own AI models and BI tools, which could impact Databricks' business (48m42s).
- The idea of offering a Databricks Cloud alternative to the big tech companies is a possibility, but it would require significant investment and resources (49m4s).
- The current focus is on establishing a particular point in the stack of trust, owning abstractions for data, and creating models that are really great, with the ability to deploy them, which can be a challenging task when moving up the stack (49m30s).
- Typically, moving up the stack becomes very hard, as seen in hardware companies moving to the cloud, or cloud companies trying to build their own hardware, with every cloud company currently building their own inference chips (49m50s).
- Databricks is not doing hardware, nor are they doing cloud, but they are working with cloud companies, driving a lot of initial revenue to them, and storing a ton of data, with a good control point due to the tabular acquisition (50m29s).
- The clouds are incentivized to work with Databricks, but this may not always be true, and there is a concern that the largest tech companies owning the major cloud platforms could lead to less innovation over time (51m9s).
- Building a hyperscale cloud requires a lot of money, and only a few companies can do it, making it difficult for new companies to enter the market, which is a concern for the future of innovation (51m32s).
- The hope is that in the future, Databricks will consider building their own cloud, but currently, it's not in their plans, and they are focused on working with existing cloud companies (51m44s).
- Historically, incumbency events in tech have led to the rise of new companies, such as Apple and Microsoft, which both started in the late 1970s with the PC, and have since become two of the largest companies in the world (52m2s).
Tech incumbents' evolution and AI competition (52m8s)
- Tech incumbents have maintained their advantage over time through multiple tech transitions, and despite being behind in innovation, they often buy into or acquire startups that are doing something innovative, allowing them to stay ahead (52m8s).
- The evolution of ecosystems requires certain capital to achieve incumbency, and tech giants have this capital, which keeps their momentum going (52m37s).
- Working with multiple tech giants allows companies to be present wherever their customers are, making it beneficial for businesses to collaborate with these incumbents (52m46s).
- The debate between small and large models is ongoing, but the key is to build the right model for a specific application, considering factors like latency and quality (53m8s).
- Trends show that no one has built a model bigger than GP4, which happened 16 months ago, and even Open AI is building smaller models, such as GPT 40 and 40 Mini (53m20s).
- Smaller models can achieve higher levels of quality, and chaining these models together in compound AI systems is the way forward for economic and modular development (53m58s).
- Large models can exist as a way to help create smaller derivative models by taking the outputs of the larger model and modifying smaller models for better performance (54m14s).
- This approach is seen in Microsoft's work with the FI model, which used 1.3 billion parameters to achieve high-quality results, and in the use of synthetic data generation from bigger models to train smaller models (54m30s).
- Building a large model can be a necessary step to create a high-quality smaller model, and this approach may be the way forward for AI development (54m51s).
AI model sizes and synthetic data quality (54m55s)
- Synthetic data is considered to be of slightly lesser quality than non-synthetic data, and this perception may be a bias that needs to be reassessed (55m6s).
- The quality of synthetic data can degrade with each subsequent generation, similar to the concept of making copies of copies, leading to a loss of accuracy and a phenomenon known as model collapse (55m44s).
- Synthetic data can still be a useful technique for augmenting models and allowing them to explore real-world distributions, but it is essential to maintain quality and keep the data grounded (56m5s).
- The field of AI has major issues, including the use of brute force methods, such as training models on massive amounts of data, which is not how humans or animals learn (56m22s).
- Humans and animals use significantly less data to develop high-quality causal models, and there is still much scientific research to be done to build efficient and low-power AI models (56m34s).
- The brain's efficiency, using only 20 watts of energy, is an encouraging example of what can be achieved with low power consumption, and researchers have been exploring the comparison between brain watts and data center watts (56m47s).
- There was an effort to replicate the human brain's neural network, which is highly efficient, but this approach seems to have been set aside during the Transformer Revolution, and it is unclear if research will shift back towards biology in the future (57m11s).
AI inspiration from biology and future innovation (57m29s)
- The human brain project and similar initiatives were considered poor facsimiles of what would lead to intelligence, as they tried to replicate something without understanding why it exists, making the systems delicate and prone to falling apart with small parameter errors (57m29s).
- Extracting principles from biology might be a more sensible approach, and looking at biology could still be a useful path forward, as there's a different dimension to more data that's currently missing in these systems (57m51s).
- Current AI systems lack the ability to understand how to make better decisions and self-critique, unlike the human brain, which has multiple systems that interact in interesting and precise ways (58m8s).
- The book "Thinking Fast and Slow" by Daniel Kahneman has roots in neurobiology and explores the different systems in the brain, including those that make learning without parameters possible and those that simulate different realities to make good decisions (58m21s).
- AI systems today don't have a good grounding in reality and are mostly pattern-matching against training data, but taking inspiration from biology without direct mimicry could lead to improvements (58m48s).
- There's still much work to be done, but the idea that there's still so much improvement coming in the ability to build intelligent software systems is a significant takeaway (59m10s).
- The next 10 years are expected to bring incredibly quick development in AI, leading to something truly marvelous, similar to the rapid progress made in the development of the internet (59m24s).
- The progress made in cloud computing, live updates, and applications running within a browser was not contemplated when the first web browser came out, and similar innovations can be expected in AI (59m40s).
- Companies like Google have figured out how to build resilient infrastructure, and this engineering has to happen to achieve high reliability and uptime (1h0m9s).
- ChatGPT was released just two years ago, and there's still 10 years of innovation left to make these AI systems really amazing (1h0m21s).
AI startup landscape and healthcare applications (1h1m1s)
- As an angel investor, investments are typically made in companies or founders that can be useful to, and often focus on new technologies or solving vertical problems, with a particular interest in applying AI to healthcare due to its fundamental importance to human life (1h1m38s).
- Healthcare is a key area of interest because despite having the necessary pieces to make it accessible to many people, structural reasons have prevented this from happening, and there is a desire to invest in companies that can help address this issue (1h1m51s).
- Many AI founders reach out to discuss their companies, and there is a willingness to talk to any founders who are building something cool (1h2m1s).
- Databricks is open to making acquisitions, having a corporate development team that scans for companies that fill in holes or solve problems that Databricks does not have a good solution for, but is thoughtful about its approach and only acquires companies that strategically align with its goals (1h2m22s).
- Acquisitions are assessed based on factors such as whether they solve a problem that Databricks does not have a good solution for, whether they have a customer base that Databricks wants access to, and whether they can be integrated into Databricks' existing products (1h2m40s).
- An example of a successful acquisition is Wac AI, which created a user interface for embedding data and visualizing it, and has since been morphed into Databricks' products (1h2m55s).
Databricks' growth trajectory and potential IPO (1h3m6s)
- Databricks is interested in making more acquisitions to grow and have a bigger impact on its customers, with the company currently growing fast and expanding its revenue by over 60% year on year (1h3m7s).
- The company's rapid growth makes it a potential major player for other companies looking for an exit, and it is likely to be a key player in the industry in the future (1h3m20s).
- Databricks' growth trajectory has led to speculation about a potential initial public offering (IPO), with the company's response being that it will happen eventually, but not yet (1h3m34s).
- There is speculation that Databricks may go public as early as H1 2025, although this has not been officially confirmed by the company (1h3m39s).
- Naveen Rao, a representative of Databricks, can be found online on Twitter, where he is active and engages in discussions about AI, using the handle @navengrao (1h4m1s).
- Rao is also on LinkedIn, where people can find him by searching for his name (1h4m8s).
Elliot Easterling of bonbon joins TWIST for a Jam with JCal. (1h4m25s)
- A "Jam with JCal" session is a discussion where a startup founder shares their work, customers, product, goals, and vision for changing the world (1h4m26s).
- The founder is then asked about their biggest challenges and struggles, and a dialogue ensues to solve problems, which is a key aspect of startups (1h4m41s).
- The host has experience investing in 400 companies, taking over 10,000 pitches from founders, and conducting 2,000 episodes of "This Week in Startups" (1h4m48s).
- The guest for the session is Elliot Easterling (1h5m13s).
Bonbon.tech and the rewards platform overview (1h5m16s)
- Bonbon.tech is a company that offers a rewards platform for publishers, allowing them to reward anything and drive more engagement and higher registration rates (1h5m58s).
- The company aims to solve the pain points of ad-focused publishers, who have been suffering from big tech changes such as cookie deprecation, reduced search results, and social media algorithms referring less traffic (1h6m12s).
- Bonbon.tech's platform provides consumers with relevant rewards, access to unique content, simple and transparent data and privacy controls, and a better user experience (1h7m0s).
- Publishers benefit from the platform by getting logins, which re-enable cookies and lost IDs, and allow them to build direct relationships with their users (1h7m13s).
- The platform also offers a gamified engagement points program that drives repeat visitors, page views, and video watches, resulting in five times more monetization per user (1h7m26s).
- Bonbon.tech's optimization engine drives outcomes such as 300% higher registration rates, 100% more engagement, and 250% higher ad rates (1h8m6s).
- The company has found that 54% of people who log in will complete their data profiles, providing a richer understanding of the publisher's site users (1h8m15s).
Demonstrating Bonbon's technology and user stats (1h8m29s)
- Bonbon's technology allows publishers to trigger a rewards window, either inline or as a pop-up, which runs multiple offers simultaneously to determine what users care about most through machine learning, resulting in a 3X higher registration rate (1h8m46s).
- The rewards window offers users the chance to win prizes, such as a television set, in exchange for logging in or registering, and users are automatically entered into the contest upon registration (1h9m13s).
- After registration, the process is gamified, encouraging users to provide more information, such as their name, zip code, and gender, with high response rates: 94% for name, 91% for zip code, 89% for gender, and 54% for phone number verification (1h9m30s).
- Users also earn points for reading articles, which drives 100% more engagement (1h9m48s).
- Bonbon's platform consists of three parts: the Open Identity Manager, which collects and manages first-party data, the Rewards Engine, which runs hundreds of offers, and frontend tools that deliver the product to consumers (1h9m57s).
- The platform also includes an API that allows publishers to issue rewards on their own (1h10m13s).
- Bonbon has deployed its technology on 27 websites, with 60,000 registered Bonbon members, and has built a first-party data file of 60,000 users as of last week (1h10m27s).
- The company's publisher network generates 60 million monthly page views (1h10m45s).
Publisher financials and Bonbon's business models (1h11m15s)
- Many publications, such as Gadget, Auto blog, The Verge, and others created by Vox, are currently facing challenges, including constrained budgets, flat growth, or contraction, making it essential to carefully select ideal customer profiles (1h11m15s).
- To address the budget constraints of publishers, two solutions are offered: a SaaS platform for Enterprise publishers, where they can pay a SaaS fee, and a free version with ads, which requires publishers to meet a minimum size requirement to qualify (1h11m51s).
- The free version with ads injects ads into all modules, essentially paying for the full program, including rewards, making it a risk-free option for publishers (1h12m2s).
- The Enterprise version allows publishers to opt-out of owning user profiles if they pay enough money, enabling them to keep their user data exclusive (1h12m16s).
Privacy and user engagement in Bonbon's platform (1h12m27s)
- Bonbon's platform is a cross-publisher rewards program that issues rewards across multiple publishers, allowing the cost of rewards to be abstracted and split among them, making it more manageable for individual publishers (1h12m27s).
- The platform provides a privacy guarantee, allowing users to opt out of any publisher they want, which is a key part of Bonbon's value proposition (1h13m7s).
- Users have the option to participate or not participate in the rewards program, and they can choose to share their data with publishers in exchange for personalized content and potential rewards (1h13m23s).
- The platform allows for personalization, enabling users to receive content and offers that are relevant to their interests, such as sales for men's products or tickets to specific sports games (1h13m56s).
- The data collected through the platform can be used to personalize content for users and provide them with opportunities to participate in sweepstakes and other gamification elements (1h14m25s).
- Gamification is a planned feature for the platform, although no specific examples have been implemented yet (1h14m39s).
Gamification strategies and user engagement (1h14m44s)
- After people register, a weekly email campaign is sent to them with personalized articles that earn extra points, enabling gamification through a newsletter program (1h14m45s).
- Point bonuses are offered for completing specific tasks, such as visiting a publisher's website and playing one of their games, which can earn users 100 points (1h15m3s).
- The goal is to create hyper-engaged users through communication, directing traffic back to the publisher's site, and rewarding them for their behavior and activity (1h15m23s).
- A tried and true gamification strategy is inviting a friend or referring a member, where users can earn points by entering a friend's email and having them register (1h15m34s).
- This referral strategy is similar to those used by companies like Robin Hood, Uber, and Dropbox, where users are rewarded for gifting or sharing services with others (1h16m3s).
- The business model has two ways to win: through tools and networks, making it an interesting and potentially successful approach (1h16m14s).
Fundraising and network effect business challenges (1h16m22s)
- In today's fundraising environment, venture capitalists (VCs) prioritize revenue growth, which can be challenging for network effect businesses that focus on building distribution and user acquisition (1h16m22s).
- Bonbon's business model involves building users at zero cost, unlike most rewards businesses that pay for users, and its go-to-market strategy should focus on building distribution and getting more users (1h16m40s).
- However, VCs and the market want to see revenue growth, which can put pressure on publishers to pay for users, potentially slowing down the business (1h16m55s).
- Network-based businesses have a unique monetization approach, and proving the value of a large user base through experiments and data can help demonstrate growth potential to investors (1h17m11s).
- Running small experiments, such as sweepstakes or contests, can help drive user engagement and page views for publishers, and demonstrate the potential for growth and revenue (1h17m43s).
- By proving the effectiveness of these experiments and demonstrating user growth and engagement, businesses can correlate their efforts to revenue growth and demonstrate their potential to investors (1h19m30s).
- Ultimately, it is up to the business to run these experiments, prove their value, and demonstrate growth potential to investors, rather than relying on publishers to pay for users (1h19m2s).
Proving user engagement and strategies for growth (1h19m42s)
- To achieve viral growth in a business, especially for sales and SaaS products, a growth rate of 10% a month is not sufficient, and instead, a 5 to 10% week-over-week growth rate is needed, which can be achieved through numerous tests and experiments with low dollar amounts (1h19m42s).
- A key factor in achieving growth is to tell a unit economic story to investors, showcasing that users are acquired for free and experiments demonstrate revenue traction and engagement on a per-user basis (1h20m7s).
- To prove user engagement and strategies for growth, it's essential to show that the business can scale by investing small dollar amounts to demonstrate the potential for growth and then adding zeros to the velocity (1h21m15s).
- A business can achieve this growth by running experiments with a small number of users and then scaling up, with the goal of getting publishers addicted to the product and eventually charging them (1h20m55s).
- To get started, a business may need to invest small amounts of money to show the potential for growth on a micro basis and then imagine adding zeros to the velocity (1h21m17s).
- The team working on this project consists of a product person, a couple of engineers, and offshore part-time engineers, with a total team size that is relatively small (1h21m35s).
- The business is in its early stages, having raised $1.4 million in funding, and is looking to achieve a network effect by investing in giving away its product and leveraging social media and platforms like TikTok (1h22m0s).
Exploring growth opportunities and market expansion (1h22m18s)
- The publishing industry is experiencing a decline in attention, and businesses in this sector are contracting, making it challenging to sell tools to them, much like trying to sell deck chairs on the Titanic (1h22m21s).
- In contrast, growing areas include TikTok, shorts, video podcasts, and other emerging trends, which could be potential opportunities for expansion (1h22m35s).
- It's essential to consider the potential impact of engaging with a large number of users, such as 60,000 TikTokers, and how this could shape the future of the business (1h22m45s).
- The success of a business can be likened to surfing, where the size and quality of the waves (or market trends) can greatly impact performance, and it's crucial to identify and ride the big waves (1h22m57s).
- The current market or "beach" being surfed may have a tide that's going out faster than the business can grow, making it essential to reassess and adapt to changing trends (1h23m26s).
- The importance of catching emerging trends is highlighted by personal experiences of catching the blog, magazine, podcasting, and angel/seed investing waves, which have led to successful outcomes (1h23m35s).
Content formats and e-commerce strategies (1h23m46s)
- Investing in an incubator can be beneficial, but sometimes it's necessary to catch bigger waves outside of it to achieve success (1h23m48s).
- Alternative content formats such as live streaming and shorts on platforms like TikTok and YouTube can be effective for businesses (1h23m57s).
- The speaker encourages businesses to think about live streaming as a potential format for their content (1h24m8s).
- Having an interesting business idea is not enough; it's essential to have product-market fit or market pull to succeed (1h24m12s).
- Raising a lot of money without having product-market fit or market pull can put a business in a challenging position (1h24m19s).
- Finding another "beach" or market with bigger waves can be necessary for success, and e-commerce is another area to consider (1h24m31s).
- Direct-to-consumer businesses have struggled with Facebook advertising, but some have found success on platforms like TikTok, social media, and podcasting (1h24m36s).
- The environment and platform used can significantly impact a business's success, and it's essential to experiment and find the right fit (1h24m50s).
- Bonbon's Reward Innovation is testing new areas and experimenting with different approaches, but the details are not disclosed (1h25m2s).
- The domain name "bonbon.b.te" is mentioned, and listeners are encouraged to visit the website to learn more (1h25m19s).
- The episode ends with a promotion for the domain name registrar "get.g.te" and an invitation to tune in to the next episode of "Jam with Jake" (1h25m29s).